Amortized Variational Inference: When and Why?
arXiv (Cornell University)(2023)
摘要
Variational inference is a class of methods to approximate the posterior distribution of a probabilistic model. The classic factorized (or mean-field) variational inference (F-VI) fits a separate parametric distribution for each latent variable. The more modern amortized variational inference (A-VI) instead learns a common \textit{inference function}, which maps each observation to its corresponding latent variable's approximate posterior. Typically, A-VI is used as a cog in the training of variational autoencoders, however it stands to reason that A-VI could also be used as a general alternative to F-VI. In this paper we study when and why A-VI can be used for approximate Bayesian inference. We establish that A-VI cannot achieve a better solution than F-VI, leading to the so-called \textit{amortization gap}, no matter how expressive the inference function is. We then address a central theoretical question: When can A-VI attain F-VI's optimal solution? We derive conditions on the model which are necessary, sufficient, and verifiable under which the amortization gap can be closed. We show that simple hierarchical models, which encompass many models in machine learning and Bayesian statistics, verify these conditions. We demonstrate, on a broader class of models, how to expand the domain of AVI's inference function to improve its solution, and we provide examples, e.g. hidden Markov models, where the amortization gap cannot be closed. Finally, when A-VI can match F-VI's solution, we empirically find that the required complexity of the inference function does not grow with the data size and that A-VI often converges faster.
更多查看译文
关键词
inference
AI 理解论文
溯源树
样例
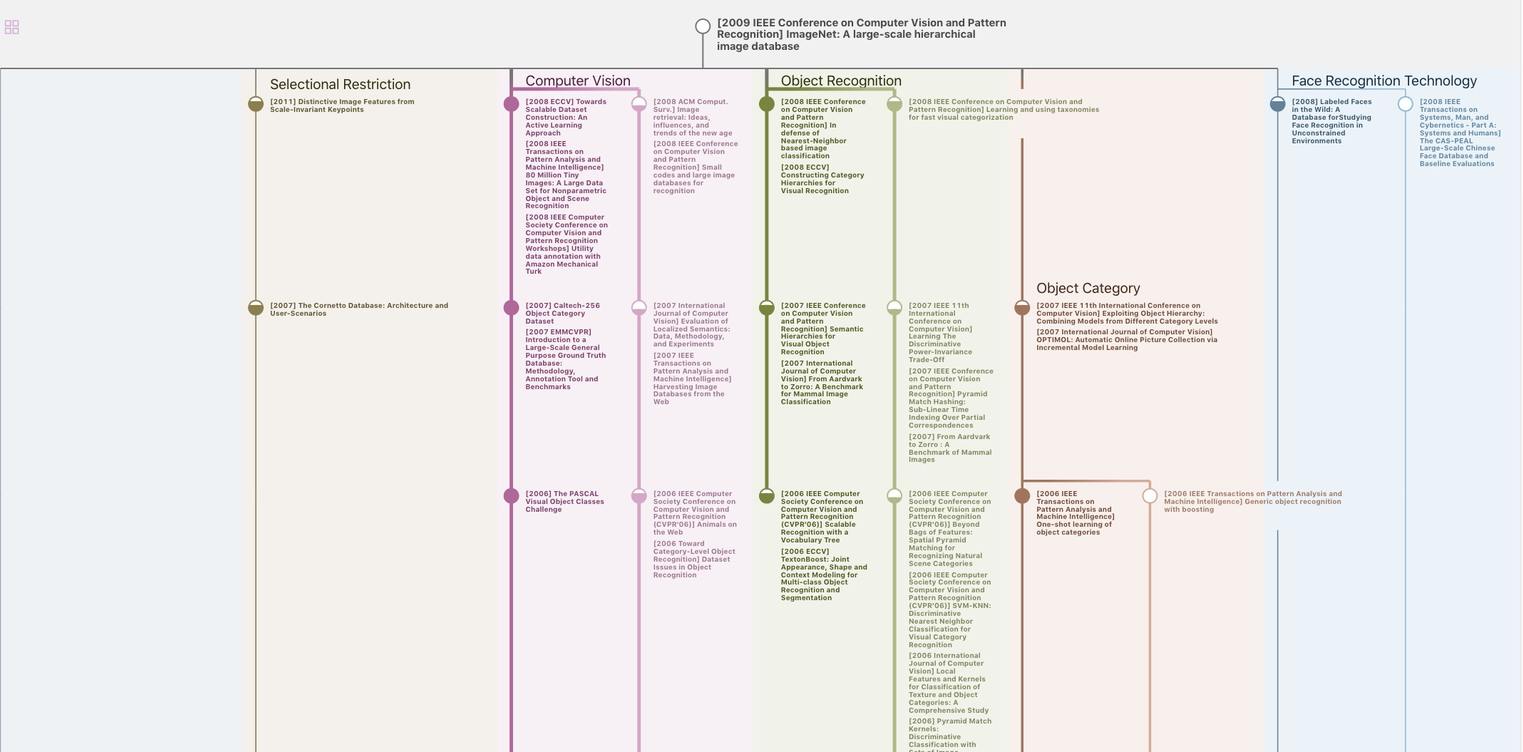
生成溯源树,研究论文发展脉络
Chat Paper
正在生成论文摘要