Physics-Informed Neural Networks for Elliptical-Anisotropy Eikonal Tomography: Application to Data From the Northeastern Tibetan Plateau
JOURNAL OF GEOPHYSICAL RESEARCH-SOLID EARTH(2023)
摘要
We develop a novel approach for multi-frequency, elliptical-anisotropic eikonal tomography based on physics-informed neural networks (pinnEAET). This approach simultaneously estimates the medium properties controlling anisotropic Rayleigh waves and reconstructs the traveltimes. The physics constraints built into pinnEAET's neural network enable high-resolution results with limited inputs by inferring physically plausible models between data points. Even with a single source, pinnEAET can achieve stable convergence on key features where traditional methods lack resolution. We apply pinnEAET to ambient noise data from a dense seismic array (ChinArray-Himalaya II) in the northeastern Tibetan Plateau with only 20 quasi-randomly distributed stations as sources. Anisotropic phase velocity maps for Rayleigh waves in the period range from 10-40 s are obtained by training on observed traveltimes. Despite using only about 3% of the total stations as sources, our results show low uncertainties, good resolution and are consistent with results from conventional tomography. Anisotropy refers to the directional dependence of seismic wave velocities, which can arise from a variety of factors such as crystal alignment, stress fields, or fluid-filled cracks. Elliptical-anisotropic eikonal tomography is a variant of eikonal tomography that can be used to estimate medium properties and reconstructed traveltimes from ambient noise data. In this study, we propose a new algorithm to implement multi-frequency, elliptical-anisotropic eikonal tomography based on physics-informed neural networks (pinnEAET), which combine data-driven models with theory-based models that include physics constraints on the system. We apply this architecture to data from a dense seismic array deployed on the northeastern Tibetan Plateau. Our results can achieve at least the same resolution as traditional methods while requiring less traveltime data. This strategy can provide new insights into the seismic imaging in case of limited or noisy data. We present a physics-informed deep learning eikonal tomography method for anisotropic velocity modelingThe algorithm incorporates wave physics to simultaneously process multi-frequency data, ensuring reliable tomographic modelsWe successfully recover the anisotropic velocity structure of the northeastern Tibet using less data than in traditional models
更多查看译文
关键词
elliptical-anisotropy eikonal tomography,anisotropy,physics informed neural network,deep learning,surface waves,Tibet
AI 理解论文
溯源树
样例
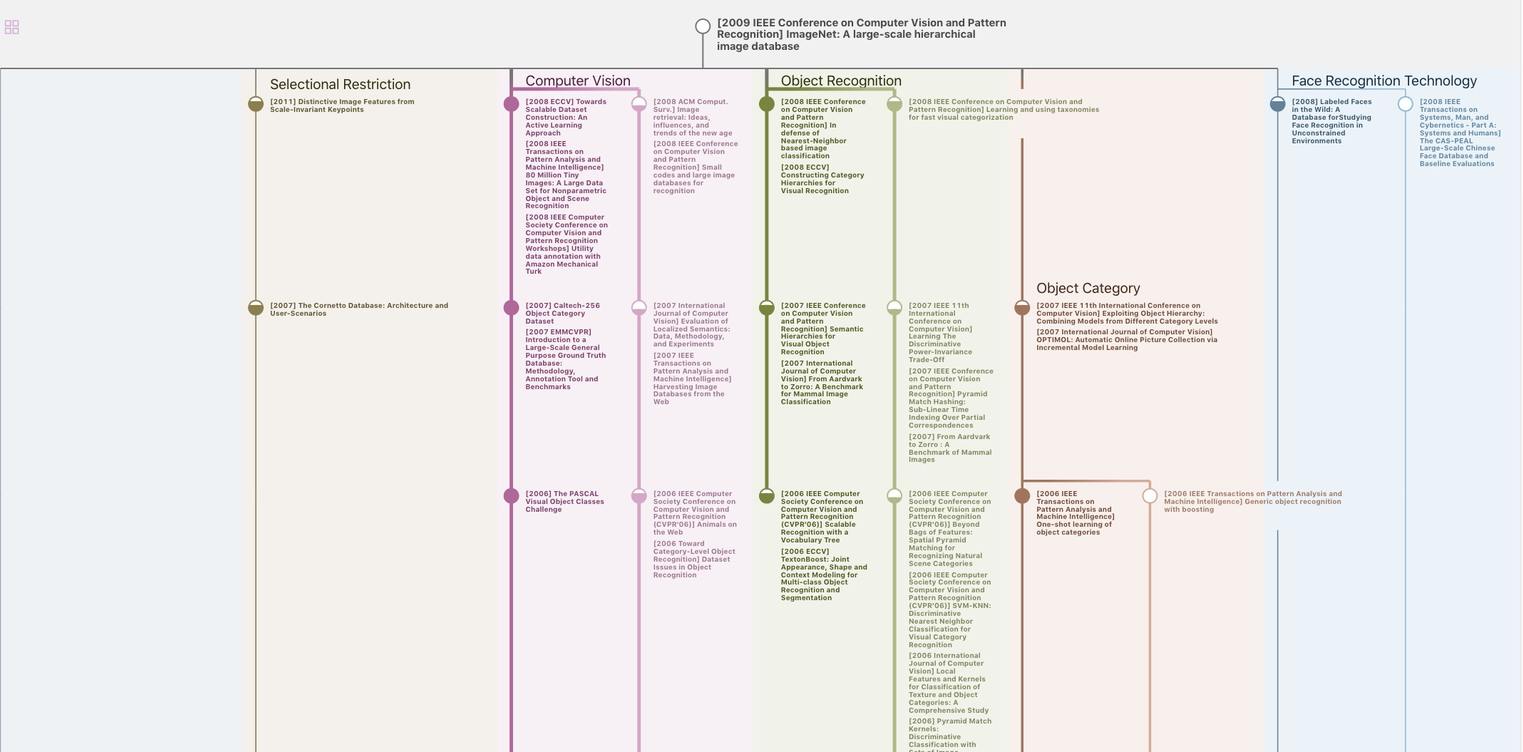
生成溯源树,研究论文发展脉络
Chat Paper
正在生成论文摘要