An ensemble 1D-CNN-LSTM-GRU model with data augmentation for speech emotion recognition
Expert Systems with Applications(2023)
摘要
Precise recognition of emotion from speech signals aids in enhancing human–computer interaction (HCI). The performance of a speech emotion recognition (SER) system depends on the derived features from speech signals. However, selecting the optimal set of feature representations remains the most challenging task in SER because the effectiveness of features varies with emotions. Most studies extract hidden local speech features ignoring the global long-term contextual representations of speech signals. The existing SER system suffers from low recognition performance mainly due to the scarcity of available data and sub-optimal feature representations. Motivated by the efficient feature extraction of convolutional neural network (CNN), long short-term memory (LSTM), and gated recurrent unit (GRU), this article proposes an ensemble utilizing the combined predictive performance of three different architectures. The first architecture uses 1D CNN followed by Fully Connected Networks (FCN). In the other two architectures, LSTM-FCN and GRU-FCN layers follow the CNN layer respectively. All three individual models focus on extracting both local and long-term global contextual representations of speech signals. The ensemble uses a weighted average of the individual models. We evaluated the model's performance on five benchmark datasets: TESS, EMO-DB, RAVDESS, SAVEE, and CREMA-D. We have augmented the data by injecting additive white gaussian noise, pitch shifting, and stretching the signal level to obtain better model generalization. Five categories of features were extracted from the speech samples: mel-frequency cepstral coefficients, log mel-scaled spectrogram, zero-crossing rate, chromagram, and root mean square value from each audio file in those datasets. All four models perform exceptionally well in the SER task; notably, the ensemble model accomplishes the state-of-the-art (SOTA) weighted average accuracy of 99.46% for TESS, 95.42% for EMO-DB, 95.62% for RAVDESS, 93.22% for SAVEE, and 90.47% for CREMA-D datasets and thus significantly outperformed the SOTA models using the same datasets.
更多查看译文
关键词
speech emotion recognition,data augmentation,d-cnn-lstm-gru
AI 理解论文
溯源树
样例
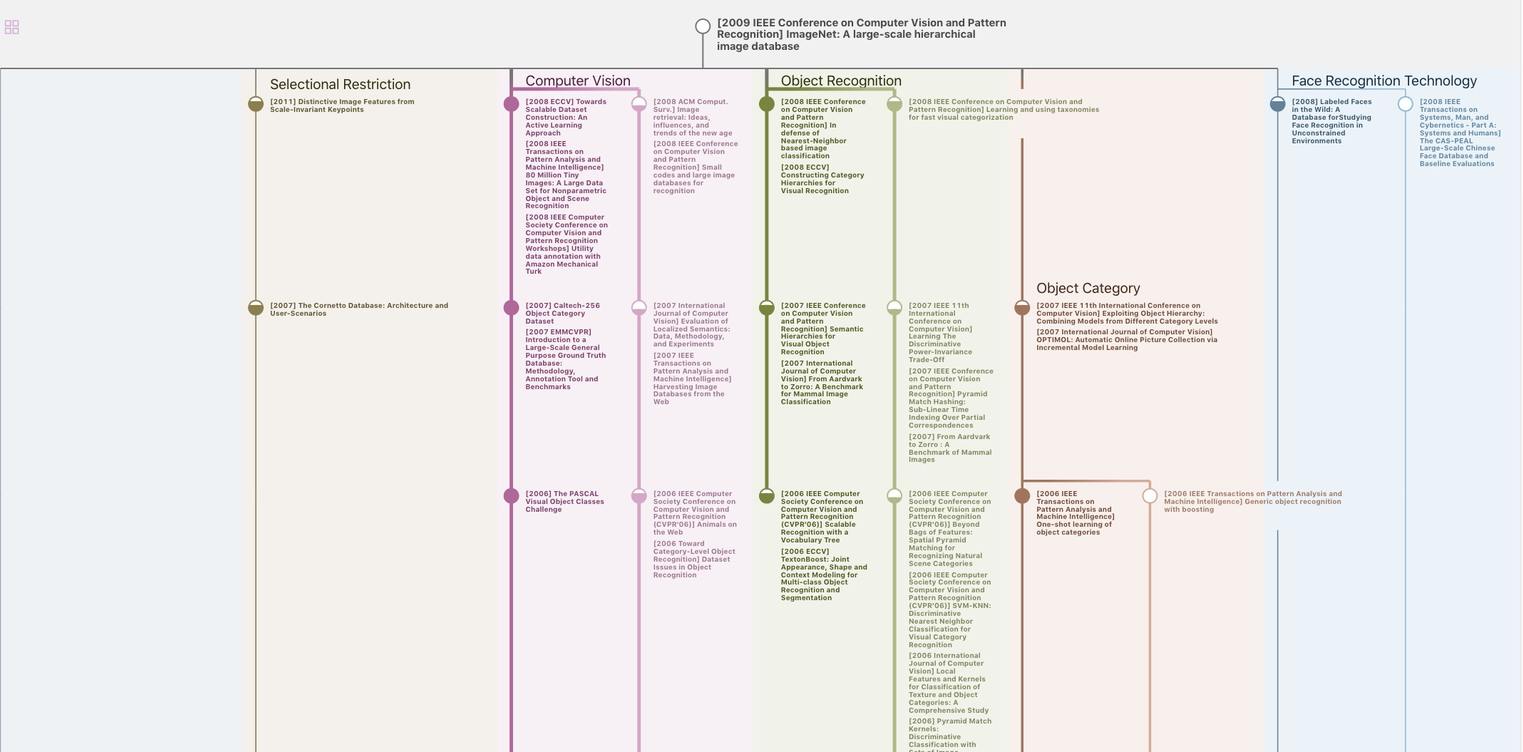
生成溯源树,研究论文发展脉络
Chat Paper
正在生成论文摘要