Discovering Key Aspects to Reduce Employee Turnover Using a Predictive Model
Communications in computer and information science(2023)
摘要
High employee turnover is a phenomenon that occurs in different types of companies and often leads to losses that could affect the organization’s productive continuity. This situation leads to the understanding, with solid evidence, of the factors that influence employees to leave their jobs and, thus, develop talent retention strategies proactively. This article proposes a predictive model to identify the most relevant factors that could cause employee turnover, specifically in the logistics process of a food and beverage production company. To achieve the objective, the CRISP-DM methodology was applied. Initially, various types of variables were identified, such as demographic, contractual, and payroll-related factors (N = 1517, period: 2017–2022). Then, five machine learning models, namely Logistic Regression, Random Forest, XGBoost, SVM, and AdaBoost, were trained, and optimal hyperparameters were used to improve the models’ performance and generalization. The performance evaluation of these models was conducted using classification metrics and the construction of confidence intervals for the accuracy metric through non-parametric Bootstrap. The results obtained demonstrate that the XGBoost and Random Forest models show the highest AUC value, with a result of 99%. This indicates that variables such as work environment, years of service, salary, workplace location, and monthly salary deductions are the most significant factors influencing the evaluated human talent to leave their job. Therefore, it is possible to conclude that the aforementioned two models are accurate and reliable for predicting employee turnover in the logistics process of the analyzed company.
更多查看译文
关键词
employee turnover,predictive model,key aspects
AI 理解论文
溯源树
样例
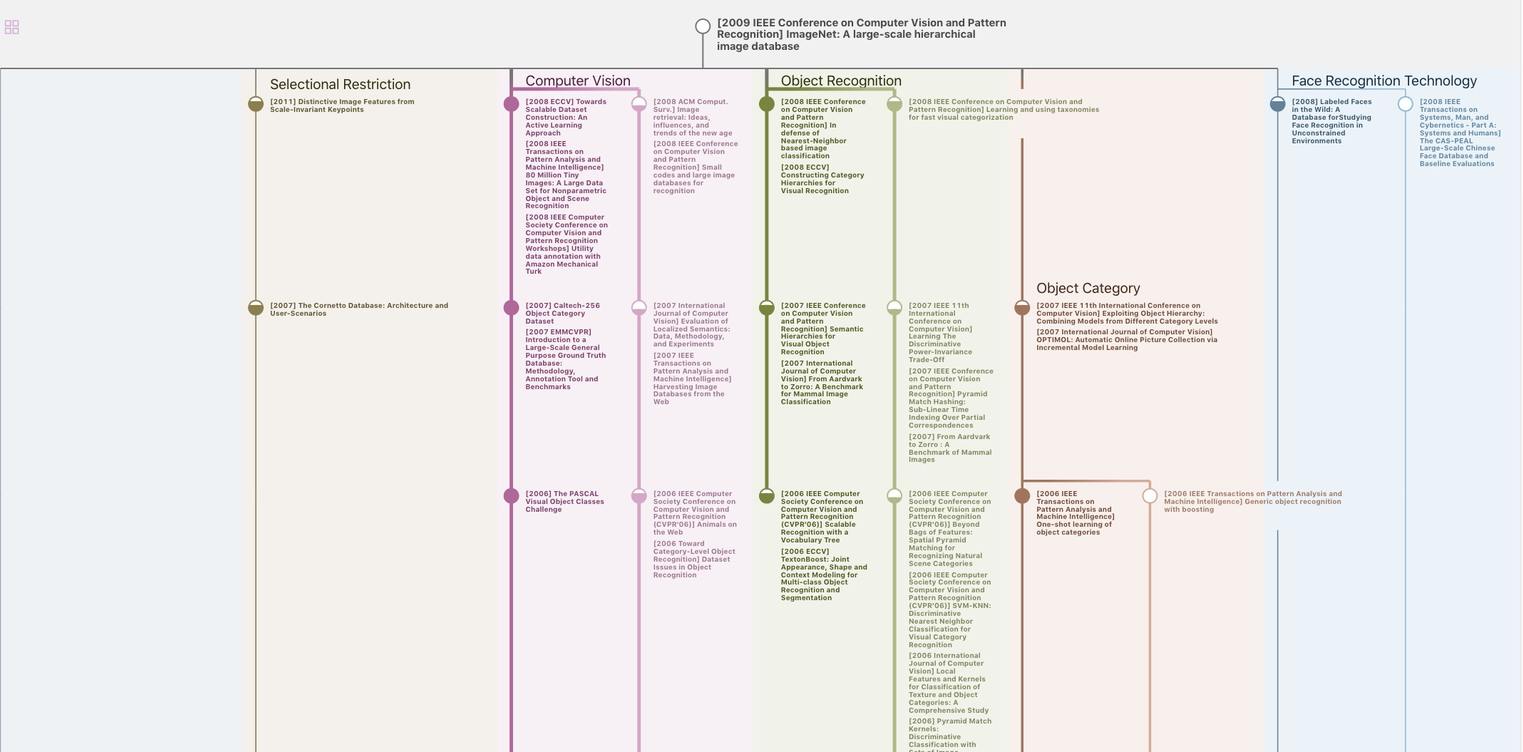
生成溯源树,研究论文发展脉络
Chat Paper
正在生成论文摘要