HCL: Hierarchical Consistency Learning for Webly Supervised Fine-Grained Recognition.
IEEE Trans. Multim.(2024)
摘要
Webly supervised fine-grained recognition aims to distinguish subordinate categories (e.g., bird species) with freely available web data. It has significant research and application value for alleviating the costly professional manual annotations' dependence in the fine-grained recognition task. Nevertheless, there exists label noise in web data to decrease the model's recognition performance. Most existing methods attempt to select clean data via loss analyses, which favors easy samples to hinder mining subtle differences contained in hard samples. Inspired by the intrinsic trait of consistent semantic predictions among different hierarchies of clean samples in fine-grained recognition, we propose a hierarchical consistency learning (HCL) approach for detecting noisy samples and capturing multi-hierarchy discriminative clues simultaneously. Specifically, our HCL approach works in a coarse-to-fine order, which first explores the semantic consistency between the image level and object level through prediction distribution conformance analyses. The open-set noise (i.e., samples irrelevant to any fine-grained subcategory) is thus detected, and the visual object information is highlighted with image-object contrastive learning. Then, the semantic consistency between object-level and part-level prediction distributions is utilized for detecting closed-set noise (i.e., samples mislabeled as other fine-grained subcategories), and local discriminative information is enhanced with object-part contrastive learning. Extensive experiments and analyses on three widely-used webly supervised fine-grained benchmark datasets demonstrate that the proposed HCL approach can achieve new state-of-the-art. The code is available at
https://github.com/PKU-ICST-MIPL/HCL_TMM2023
.
更多查看译文
关键词
Webly supervised fine-grained recognition,hierarchical consistency learning,open-set noise,closed-set noise
AI 理解论文
溯源树
样例
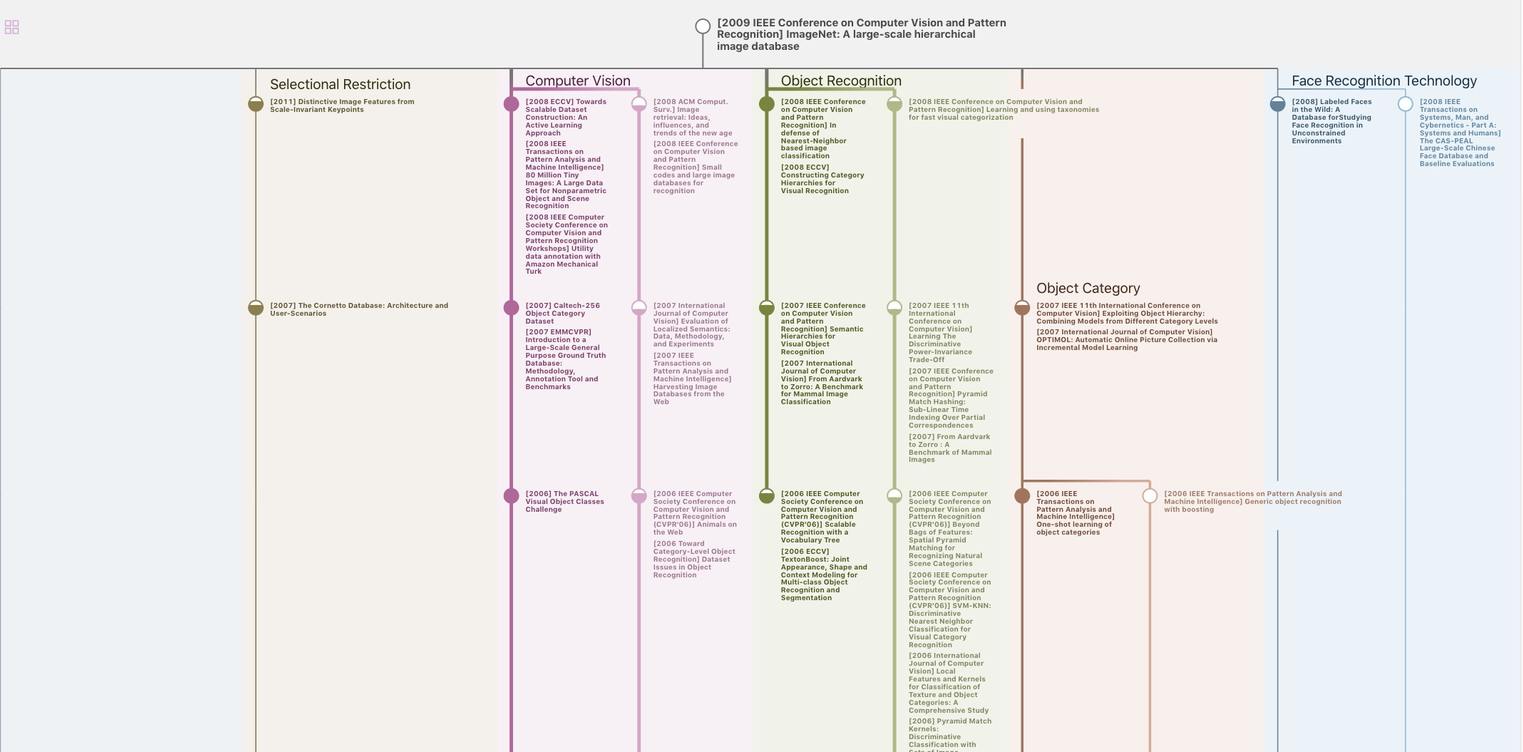
生成溯源树,研究论文发展脉络
Chat Paper
正在生成论文摘要