PARAMOUNT: Towards generalizable deeP leARning for mmwAve beaM selectiOn using sUb-6GHz chaNnel measuremenTs
IEEE Transactions on Wireless Communications(2023)
摘要
Deep neural networks (DNNs) in the wireless communication domain have been shown to be hardly generalizable to scenarios where the train and test datasets follow a different distribution. This lack of generalization poses a significant hurdle to the practical utilization of DNNs in wireless communication. In this paper, we propose a generalizable deep learning approach for millimeter wave (mmWave) beam selection using sub-6 GHz channel state information (CSI) measurements, referred to as PARAMOUNT. First, we provide a detailed discussion on physical aspects of the electromagnetic wave scattering in the mmWave and sub-6 GHz bands. Based on this discussion, we develop the augmented discrete angle delay profile (ADADP) which is a novel linear transformation for the sub-6 GHz CSI that extracts the angle-delay attributes and provides a semantic visual representation of the multi-path clusters. Next, we introduce a convolutional neural network (CNN) structure that can learn the signatures of the path clusters in the sub-6 GHz ADADP representation and transform it to mmWave band beam indices. We demonstrate by extensive simulations on several different datasets that PARAMOUNT can generalize beyond the training dataset which is mainly due to transfer learning principles that allow transferring information from previously learned tasks to the learning of new unseen tasks.
更多查看译文
关键词
mmwave beam selection,deep learning
AI 理解论文
溯源树
样例
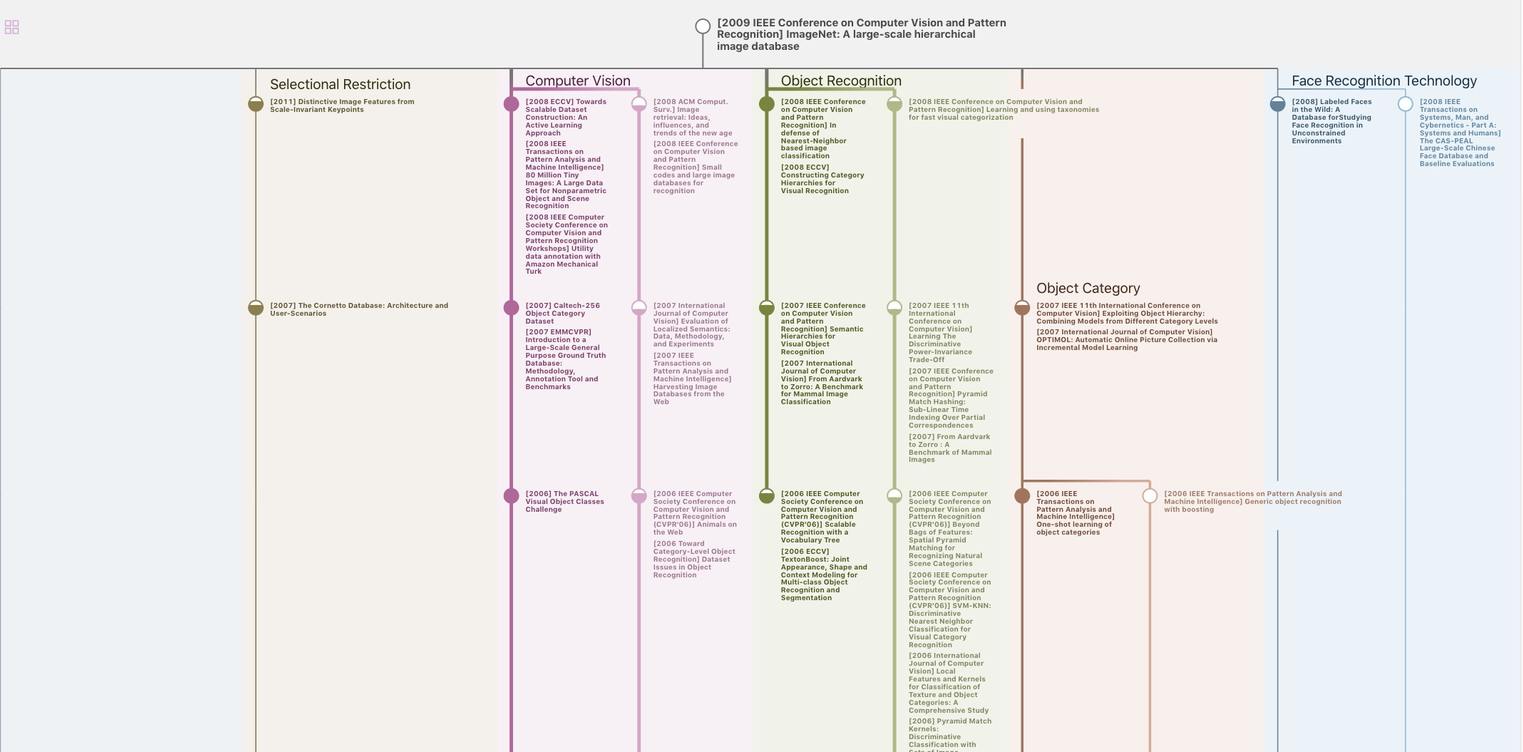
生成溯源树,研究论文发展脉络
Chat Paper
正在生成论文摘要