Distributed Deep Reinforcement Learning With Prioritized Replay for Power Allocation in Underwater Acoustic Communication Networks.
IEEE Internet Things J.(2024)
摘要
This paper studies the distributed power management problem in underwater acoustic communication networks (UACNs) with the coexistence of multiple transmitter-receiver pairs, where each transmitter selects its transmit power based only on local observations without the involvement of any central controller. This paper aims to maximize the network transmission rate based on maintaining Nash equilibrium (NE) among multiple transmitter-receiver pairs. We model the selfish behavior of each emitter as a non-cooperative game. In this game, on the one hand, the distance between the transmitter and the receiver is introduced as an interference weight factor to modify the effective interference model; on the other hand, the utility function of the game is constructed by combining the information transmission rate of the transmitter and its remaining energy. Moreover, it is successfully proved that the utility function has a NE solution. Subsequently, a multi-agent dual Q network algorithm (MA-DDQN-PR) based on priority replay is proposed to achieve the optimal transmission strategy in dynamically changing channel and interference environments. Each transmitter in this algorithm acts as an agent, interacting with the communication environment and receiving different observations. But at the same time, they have a common reward function and centrally train the Q network by summarizing the actions of other agents, thereby improving the power control selected by each agent. Finally, simulation results show that the proposed algorithm outperforms other existing learning algorithms in terms of network transmission rate, network energy efficiency, and transmitter lifetime.
更多查看译文
关键词
Underwater Acoustic Communication Networks,multi-transmitter-receiver,Nash equilibrium,multi-agent double-Q network,prioritized replay
AI 理解论文
溯源树
样例
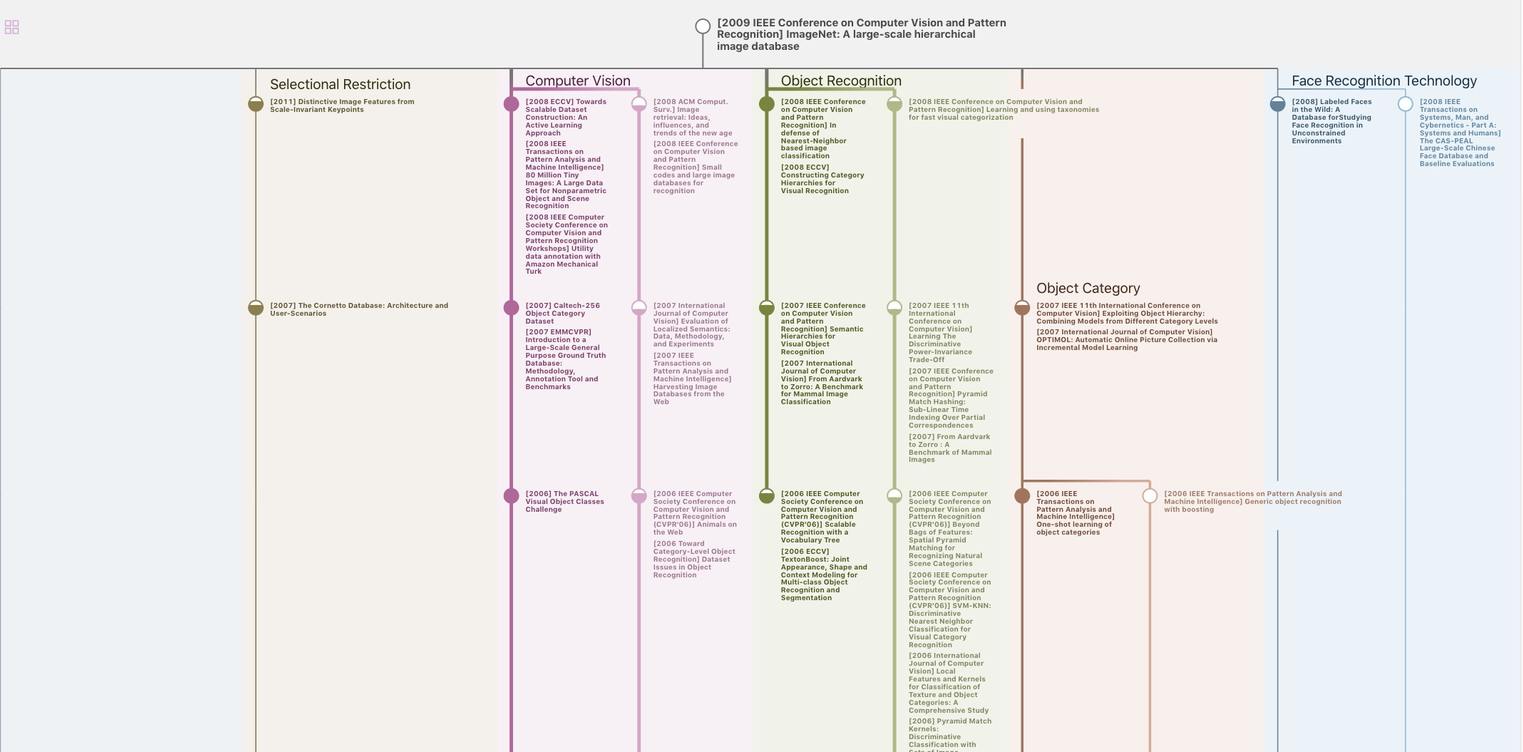
生成溯源树,研究论文发展脉络
Chat Paper
正在生成论文摘要