OP06.05: Automatic fetal mid‐sagittal plane detection using deep learning‐based 3D segmentation network
Ultrasound in Obstetrics & Gynecology(2023)
摘要
Increased fetal nuchal translucency (NT) on mid-sagittal plane (MSP) is considered biomarker associated with increased risk for chromosomal and structural anomalies, or twin–twin transfusion syndrome. Because accurate MSP acquisition depends on operator ability as well as time and labour consuming, an automated system has been proposed, but its clinical use has been limited due to its limitations. In light of this, we present a novel solution for automated MSP detection from three-dimensional (3D) ultrasound images. The provided images were obtained by an expert with W10 (Samsung Medison, Seoul, Republic of Korea) ultrasound machine using a 2–6 MHz transabdominal transducer. Herein, we propose a method that uses a deep learning-based algorithm to segment key landmark structures, such as the fetal head, midlines, choroid plexus, nuchal translucency, and diencephalon, and automatically navigate the MSP based on these landmarks. The output MSP images were audited by 2 ultrasound experts for plane acceptance check. A total of 300 3D volume data set of fetal head and upper thorax was obtained from 268 patients during first trimester NT screening at our institution of which 200 were used for training and 100 testing for 3D autolabelling system, and validation of automated NT detection system, respectively. A success rate of 93% for 3D segmentation and an accuracy of 91.5% for MSP detection was achieved with an inference time of 0.5 seconds. Of note, MSP was successfully abstracted from the volume data set irrespective of angulation or fetal prone positioning. The proposed method using deep learning-based 3D segmentation and automatic MSP navigation algorithm demonstrated high performance in accurately obtaining fetal MSP.
更多查看译文
关键词
plane,segmentation,deep learning‐based
AI 理解论文
溯源树
样例
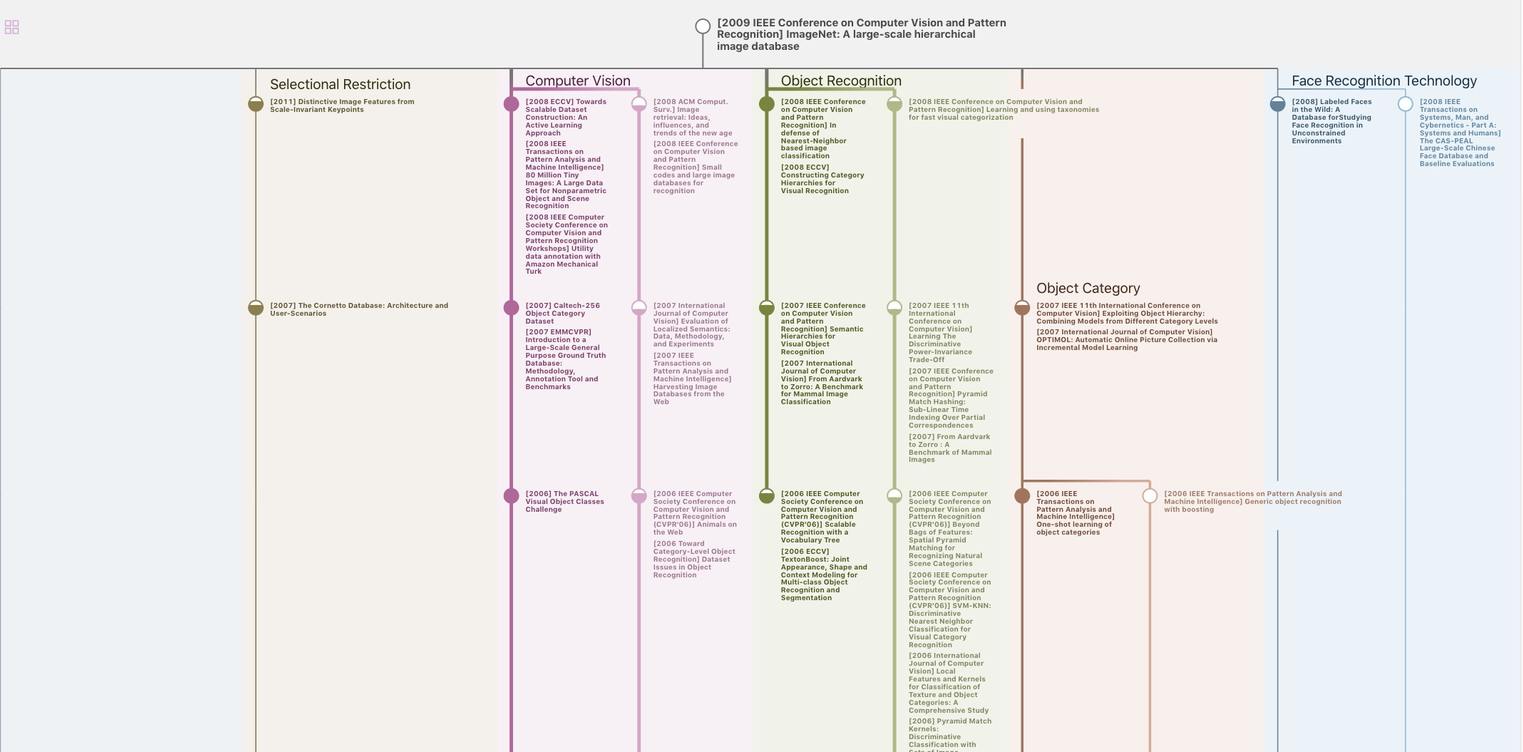
生成溯源树,研究论文发展脉络
Chat Paper
正在生成论文摘要