Multimodal Religiously Hateful Social Media Memes Classification based on Textual and Image DataJust Accepted
ACM Transactions on Asian and Low-Resource Language Information Processing(2023)
摘要
Multimodal hateful social media meme detection is an important and challenging problem in the vision-language domain. Recent studies show high accuracy for such multimodal tasks due to datasets that provide better joint multimodal embedding to narrow the semantic gap. Religiously hateful meme detection is not extensively explored among published datasets. While there is a need for higher accuracy on religiously hateful memes, deep learning-based models often suffer from inductive bias. This issue is addressed in this work with the following contributions. First, a religiously hateful memes dataset is created and published publicly to advance hateful religious memes detection research. Over 2000 meme images are collected with their corresponding text. The proposed approach compares and fine-tunes VisualBERT pre-trained on the Conceptual Caption (CC) dataset for the downstream classification task. We also extend the dataset with the Facebook hateful memes dataset. We extract visual features using ResNeXT-152 Aggregated Residual Transformations based Masked Regions with Convolutional Neural Networks (R-CNN) and Bidirectional Encoder Representations from Transformers (BERT) uncased for textual encoding for the early fusion model. We use the primary evaluation metric of an Area Under the Operator Characters Curve (AUROC) to measure model separability. Results show that the proposed approach has a higher AUCROC score of \(78\% \), proving the model’s higher separability performance and an accuracy of \(70\% \). It shows comparatively superior performance considering dataset size and against ensemble-based machine learning approaches.
更多查看译文
关键词
Social Media Mining,Multimodal,Hateful Memes,Transformers-based multimodal Bidirectional Encoder Representations,Memes,Deep Learning,Memes Classification
AI 理解论文
溯源树
样例
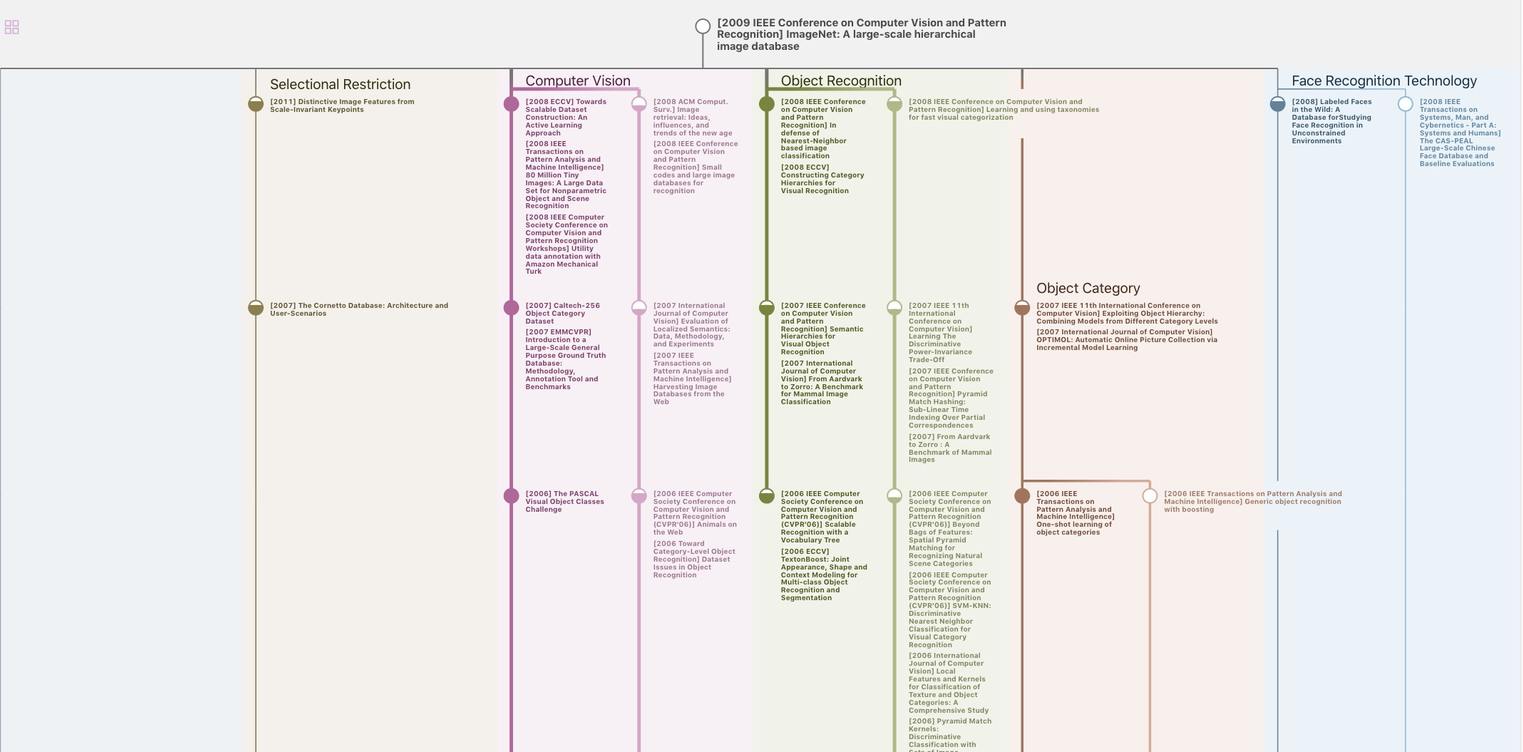
生成溯源树,研究论文发展脉络
Chat Paper
正在生成论文摘要