Deep learning-based intraoperative differentiation of primary CNS lymphoma and glioma: a discovery, multicenter validation, and proof-of concept study
Research Square (Research Square)(2023)
摘要
Abstract Intraoperative differentiation of primary central nervous system lymphoma (PCNSL) and glioma is of great importance to decision-making for neurosurgeons. However, distinguishing these two diseases based on frozen sections presents a challenge for pathologists. Here, we aim to develop and validate a deep learning model (LGNet) that could accurately differentiate PCNSL from glioma on haematoxylin and eosin (H&E)-stained frozen whole-slide images. In this study, the LGNet was developed and validated to distinguish PCNSL from glioma on independent cohorts, and its performance was compared to that of three pathologists with varying levels of expertise. Additionally, a human-machine fusion approach was designed to consider the diagnostic results from both pathologist and LGNet, to improve the integrative diagnostic performance. A proof of concept study was further evaluated with an online pathological decision support platform. The LGNet achieved high area under the receiver operating characteristic curves (AUROCs) of 0·965 and 0·972 for discriminating PCNSL and glioma on the two external validation cohorts. Moreover, the LGNet outperformed the three pathologists, and assisted them in making the distinction. The diagnostic performance human-machine fusion was further improved using the human-machine fusion. Notably, the performance of LGNet was verified with the proof of concept cohort, and it was shown that the time-consumption of LGNet was significantly less than that of pathologists ( P < 0·001) in practical scenario. Also, the study demonstrated the association between histopathological characteristics and the LGNet’s prediction as derived from the logistic regression model. These findings suggest that the LGNet accurately and timely differentiates PCNSL from glioma based on frozen sections, and adds to the enhancement of pathologists’ diagnostic performance. Thus, our deep learning model LGNet has the application potential during intraoperative diagnosis.
更多查看译文
关键词
primary cns lymphoma,glioma,intraoperative differentiation,learning-based
AI 理解论文
溯源树
样例
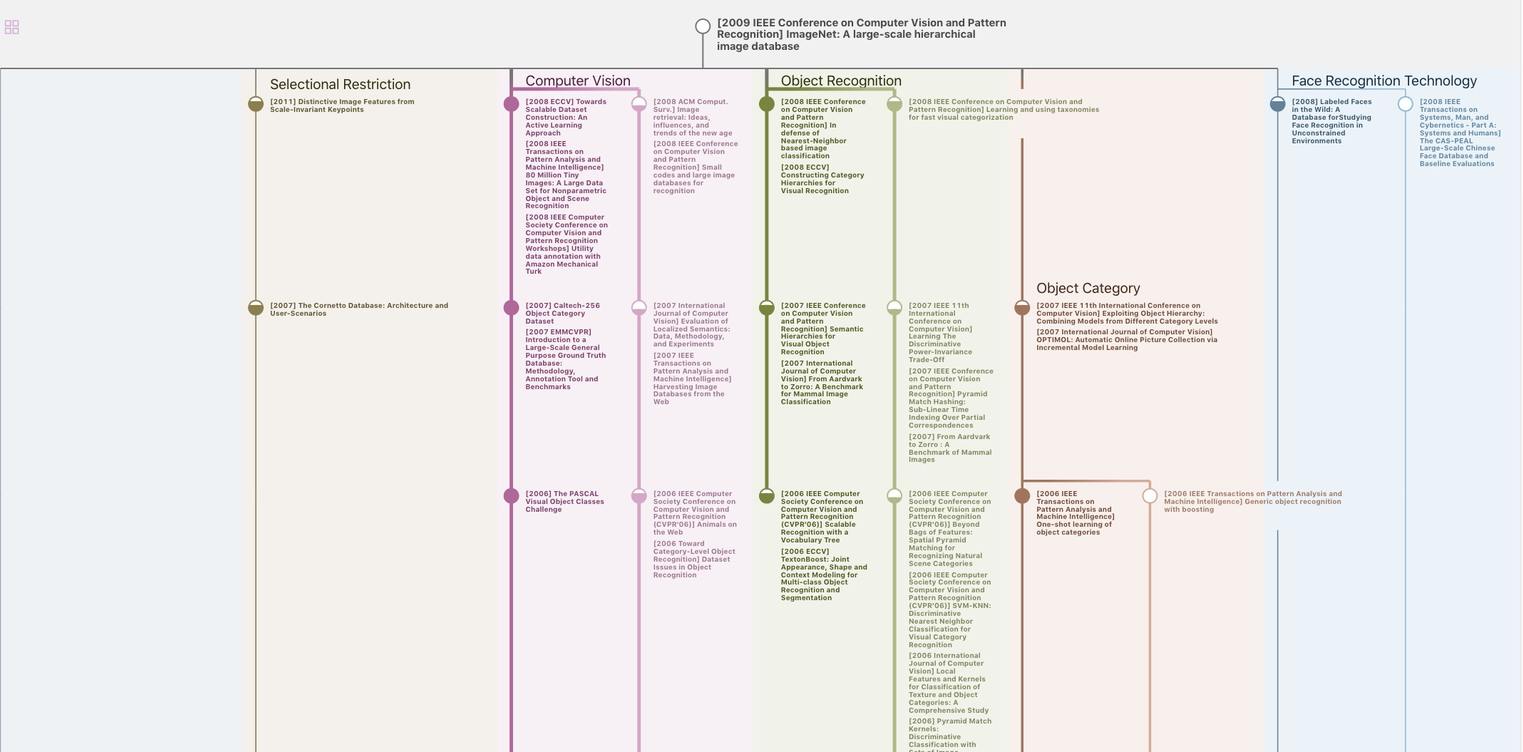
生成溯源树,研究论文发展脉络
Chat Paper
正在生成论文摘要