Set-Type Belief Propagation with Applications to Poisson Multi-Bernoulli SLAM
arXiv (Cornell University)(2023)
摘要
Belief propagation (BP) is a useful probabilistic inference algorithm for efficiently computing approximate marginal probability densities of random variables. However, in its standard form, BP is only applicable to the vector-type random variables with a fixed and known number of vector elements, while certain applications rely on RFSs with an unknown number of vector elements. In this paper, we develop BP rules for factor graphs defined on sequences of RFSs where each RFS has an unknown number of elements, with the intention of deriving novel inference methods for RFSs. Furthermore, we show that vector-type BP is a special case of set-type BP, where each RFS follows the Bernoulli process. To demonstrate the validity of developed set-type BP, we apply it to the PMB filter for SLAM, which naturally leads to new set-type BP-mapping, SLAM, multi-target tracking, and simultaneous localization and tracking filters. Finally, we explore the relationships between the vector-type BP and the proposed set-type BP PMB-SLAM implementations and show a performance gain of the proposed set-type BP PMB-SLAM filter in comparison with the vector-type BP-SLAM filter.
更多查看译文
关键词
slam,propagation,set-type,multi-bernoulli
AI 理解论文
溯源树
样例
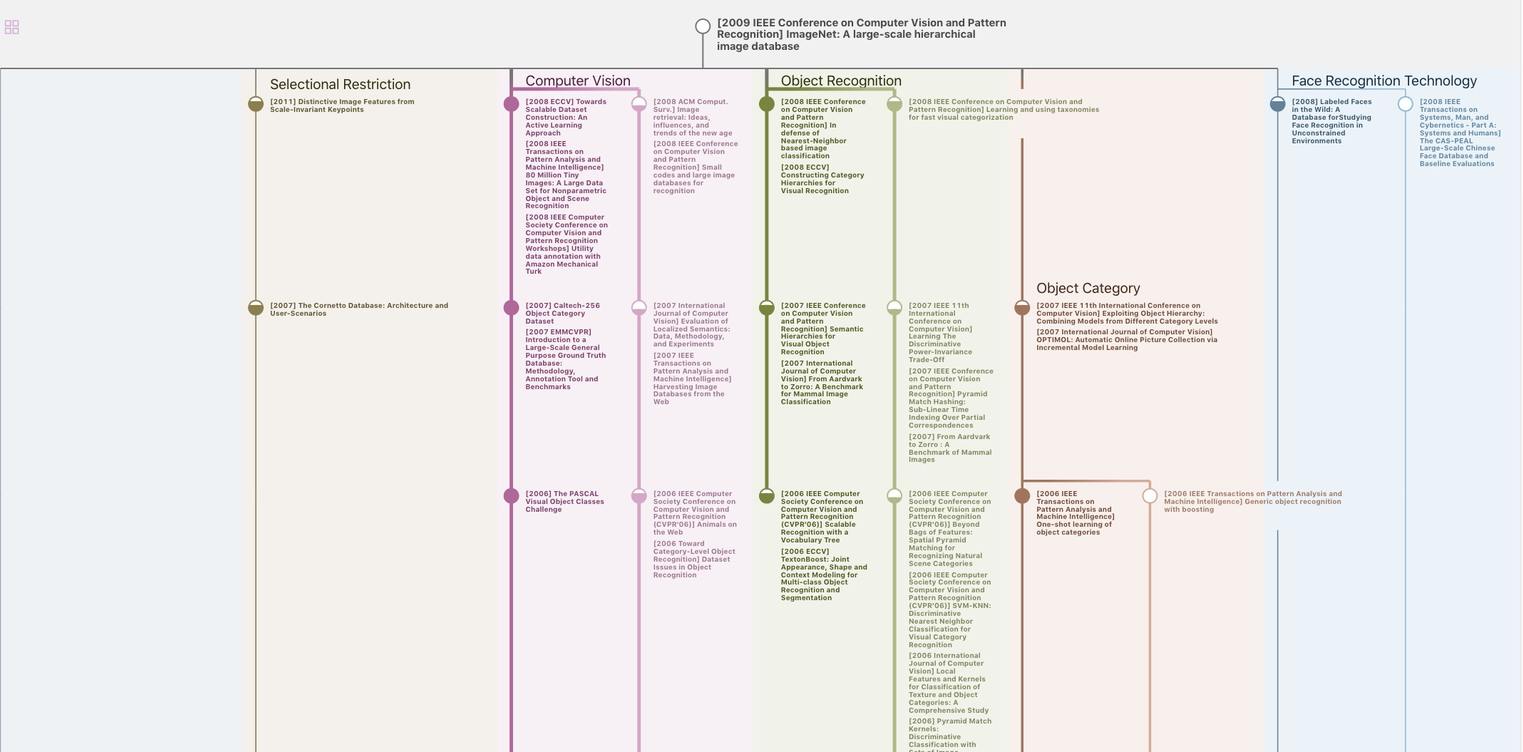
生成溯源树,研究论文发展脉络
Chat Paper
正在生成论文摘要