Identification of Biomarkers Associated with Diagnosis of Diabetic Nephropathy Patients Based on Bioinformatics and Machine Learning
Research Square (Research Square)(2023)
摘要
Abstract Diabetic nephropathy (DN) is a common microvascular complication of diabetes, and early diagnosis is crucial for the prognosis of patients. The purpose of this study was to identify novel diagnostic biomarkers of DN and to analyze possible associations between key genes and infiltrating immune cells. Download the GSE1009, GSE30528, and GSE47183 datasets, including human DN and normal samples, from the GEO dataset. Differentially expressed genes (DEGs) were identified in 33 DN cases and 26 control cases. SVM-RFE analysis and LASSO regression model were used to screen for possible markers. The composition patterns of 22 immune cell components in DN were determined by using CIBERSORT technique. In this study, 57 genes were identified as significantly up-regulated and 80 genes as significantly down-regulated. Analysis of KEGG signaling pathway showed that complete and coalition cascades, PI3K-Akt signaling pathway, focal adhesion, RAP1 signaling pathway and age-rage signaling pathway were significantly enriched. Based on the results of machine learning, we identified C1orf21, TGFBI, ATF3, and BHMT as key diagnostic genes for DN, which were further confirmed by ROC analysis. Immunocyte infiltration analysis revealed four key diagnostic gene expressions associated with multiple immunocytes in DN. Overall, our findings may offer some new insights into the exploration of new markers for the diagnosis of DN. Key genes and their relationship with immune infiltration may provide new insights into the development of DN.
更多查看译文
关键词
diabetic nephropathy patients,bioinformatics,biomarkers associated,machine learning
AI 理解论文
溯源树
样例
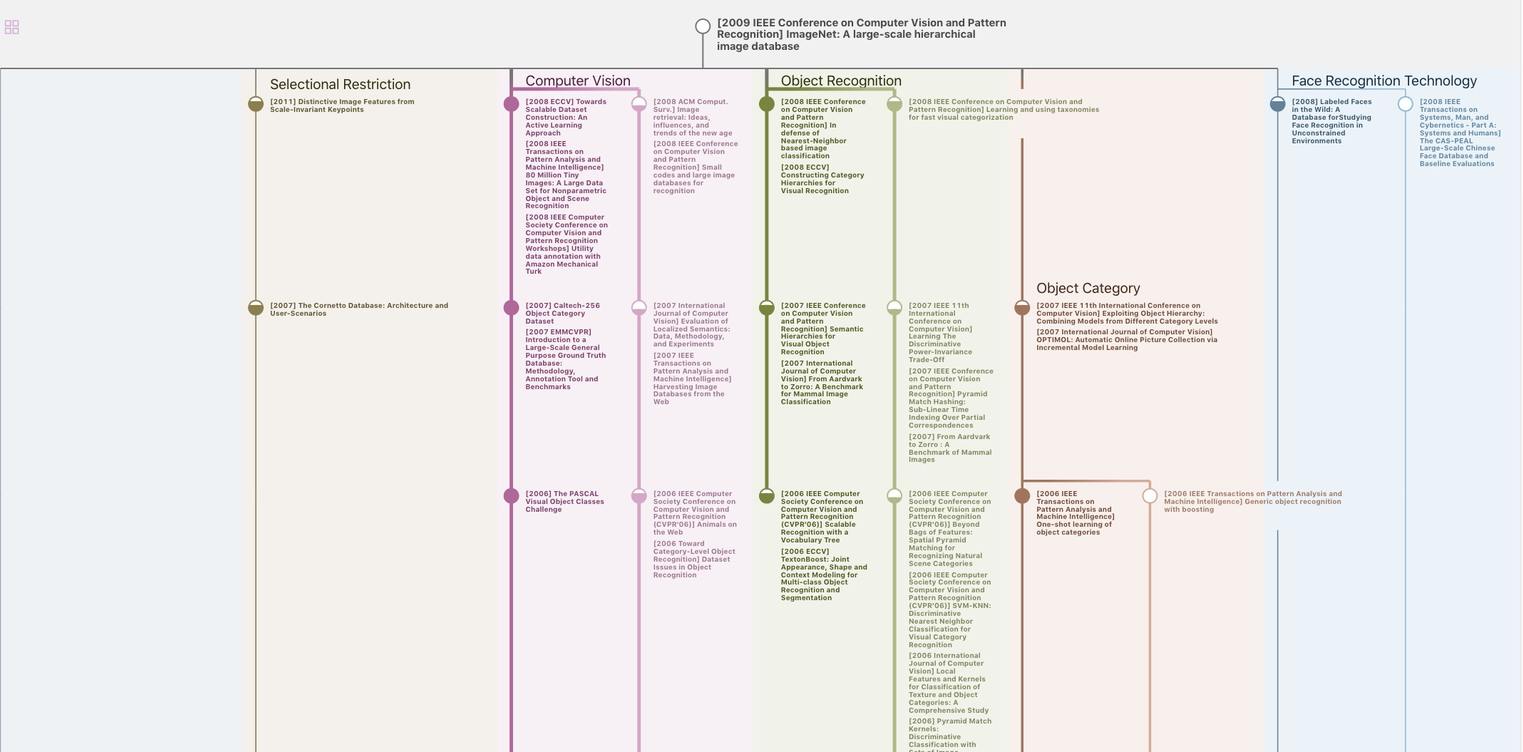
生成溯源树,研究论文发展脉络
Chat Paper
正在生成论文摘要