Variational quantum algorithm for node embedding
Fundamental Research(2023)
摘要
Quantum machine learning has made remarkable progress in many important tasks. However, the gate complexity of the initial state preparation is seldom considered in lots of quantum machine learning algorithms, making them non-end-to-end. Herein, we propose a quantum algorithm for the node embedding problem that maps a node graph’s topological structure to embedding vectors. The resulting quantum embedding state can be used as an input for other quantum machine learning algorithms. With O(log(N)) qubits to store the information of N nodes, our algorithm will not lose quantum advantage for the subsequent quantum information processing. Moreover, owing to the use of a parameterized quantum circuit with O(poly(log(N))) depth, the resulting state can serve as an efficient quantum database. In addition, we explored the measurement complexity of the quantum node embedding algorithm, which is the main issue in training parameters, and extended the algorithm to capture high-order neighborhood information between nodes. Finally, we experimentally demonstrated our algorithm on an nuclear magnetic resonance quantum processor to solve a graph model.
更多查看译文
关键词
Quantum machine learning,Quantum computation,Node embedding,Variational quantum algorithm,Nuclear magnetic resonance
AI 理解论文
溯源树
样例
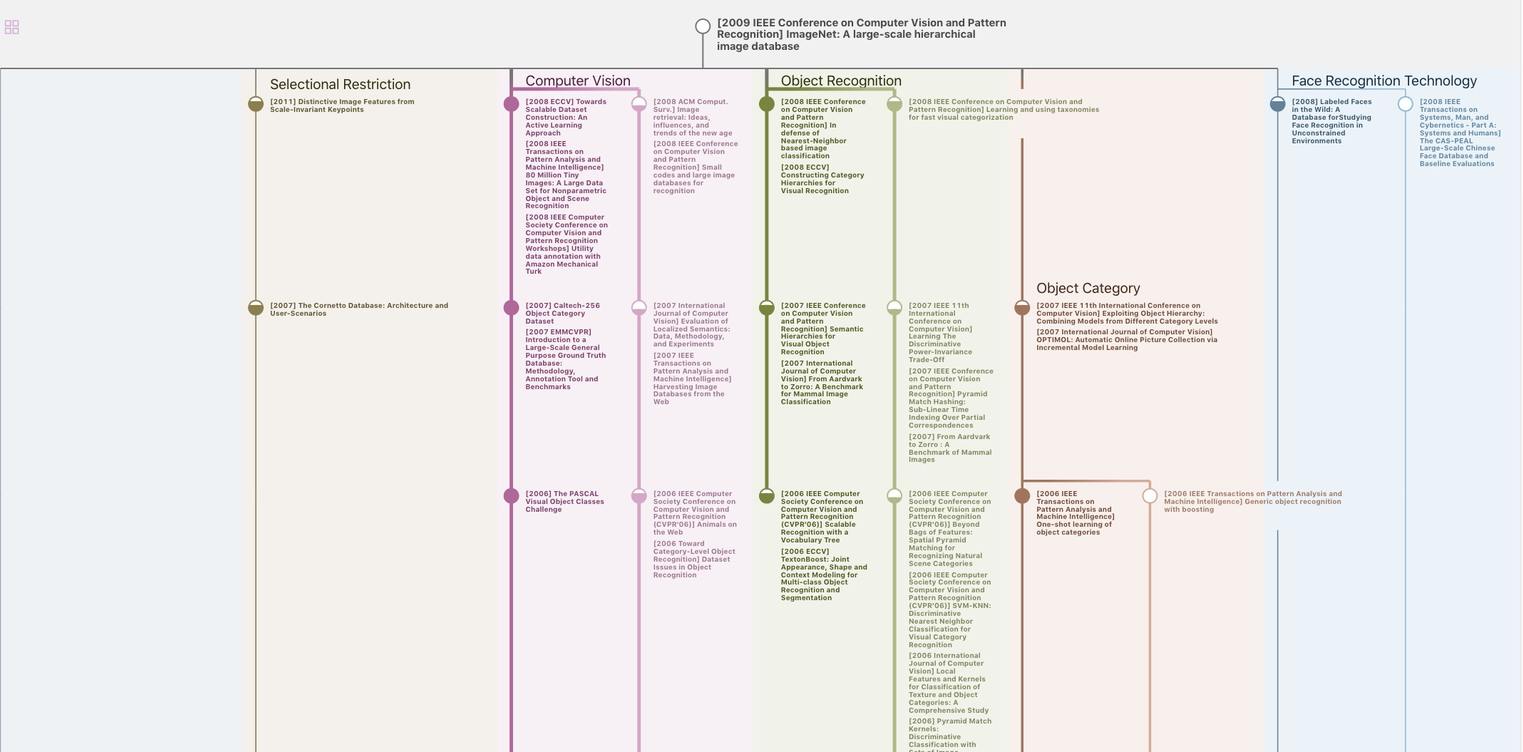
生成溯源树,研究论文发展脉络
Chat Paper
正在生成论文摘要