centroIDA: Cross-Domain Class Discrepancy Minimization Based on Accumulative Class-Centroids for Imbalanced Domain Adaptation
arXiv (Cornell University)(2023)
摘要
Unsupervised Domain Adaptation (UDA) approaches address the covariate shift problem by minimizing the distribution discrepancy between the source and target domains, assuming that the label distribution is invariant across domains. However, in the imbalanced domain adaptation (IDA) scenario, covariate and long-tailed label shifts both exist across domains. To tackle the IDA problem, some current research focus on minimizing the distribution discrepancies of each corresponding class between source and target domains. Such methods rely much on the reliable pseudo labels' selection and the feature distributions estimation for target domain, and the minority classes with limited numbers makes the estimations more uncertainty, which influences the model's performance. In this paper, we propose a cross-domain class discrepancy minimization method based on accumulative class-centroids for IDA (centroIDA). Firstly, class-based re-sampling strategy is used to obtain an unbiased classifier on source domain. Secondly, the accumulative class-centroids alignment loss is proposed for iterative class-centroids alignment across domains. Finally, class-wise feature alignment loss is used to optimize the feature representation for a robust classification boundary. A series of experiments have proved that our method outperforms other SOTA methods on IDA problem, especially with the increasing degree of label shift.
更多查看译文
关键词
imbalanced cross-domain adaptation,centroida,class-centroids
AI 理解论文
溯源树
样例
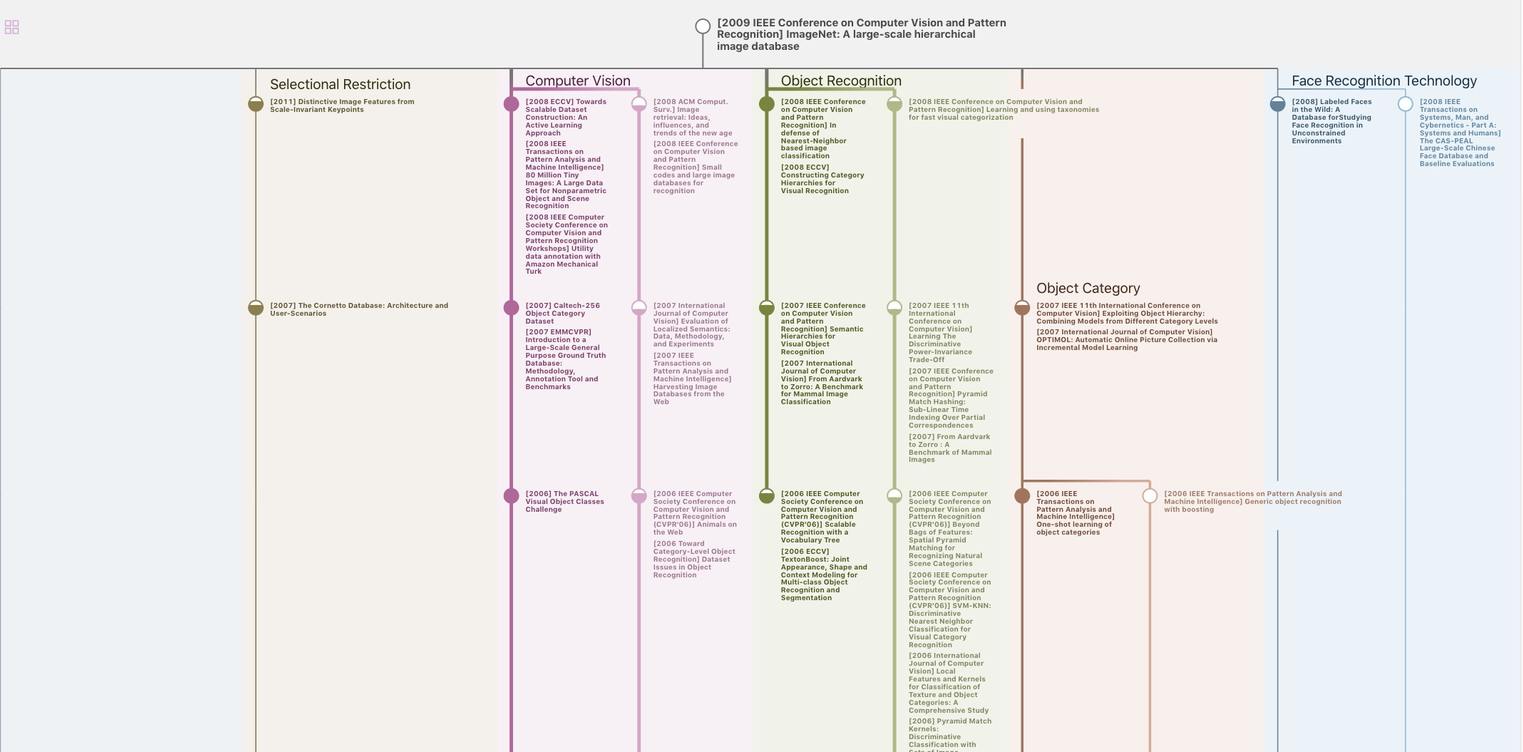
生成溯源树,研究论文发展脉络
Chat Paper
正在生成论文摘要