Unbiased Image Synthesis via Manifold-Driven Sampling in Diffusion Models
arXiv (Cornell University)(2023)
摘要
Diffusion models are a potent class of generative models capable of producing high-quality images. However, they can face challenges related to data bias, favoring specific modes of data, especially when the training data does not accurately represent the true data distribution and exhibits skewed or imbalanced patterns. For instance, the CelebA dataset contains more female images than male images, leading to biased generation results and impacting downstream applications. To address this issue, we propose a novel method that leverages manifold guidance to mitigate data bias in diffusion models. Our key idea is to estimate the manifold of the training data using an unsupervised approach, and then use it to guide the sampling process of diffusion models. This encourages the generated images to be uniformly distributed on the data manifold without altering the model architecture or necessitating labels or retraining. Theoretical analysis and empirical evidence demonstrate the effectiveness of our method in improving the quality and unbiasedness of image generation compared to standard diffusion models.
更多查看译文
关键词
unbiased image synthesis,sampling,diffusion
AI 理解论文
溯源树
样例
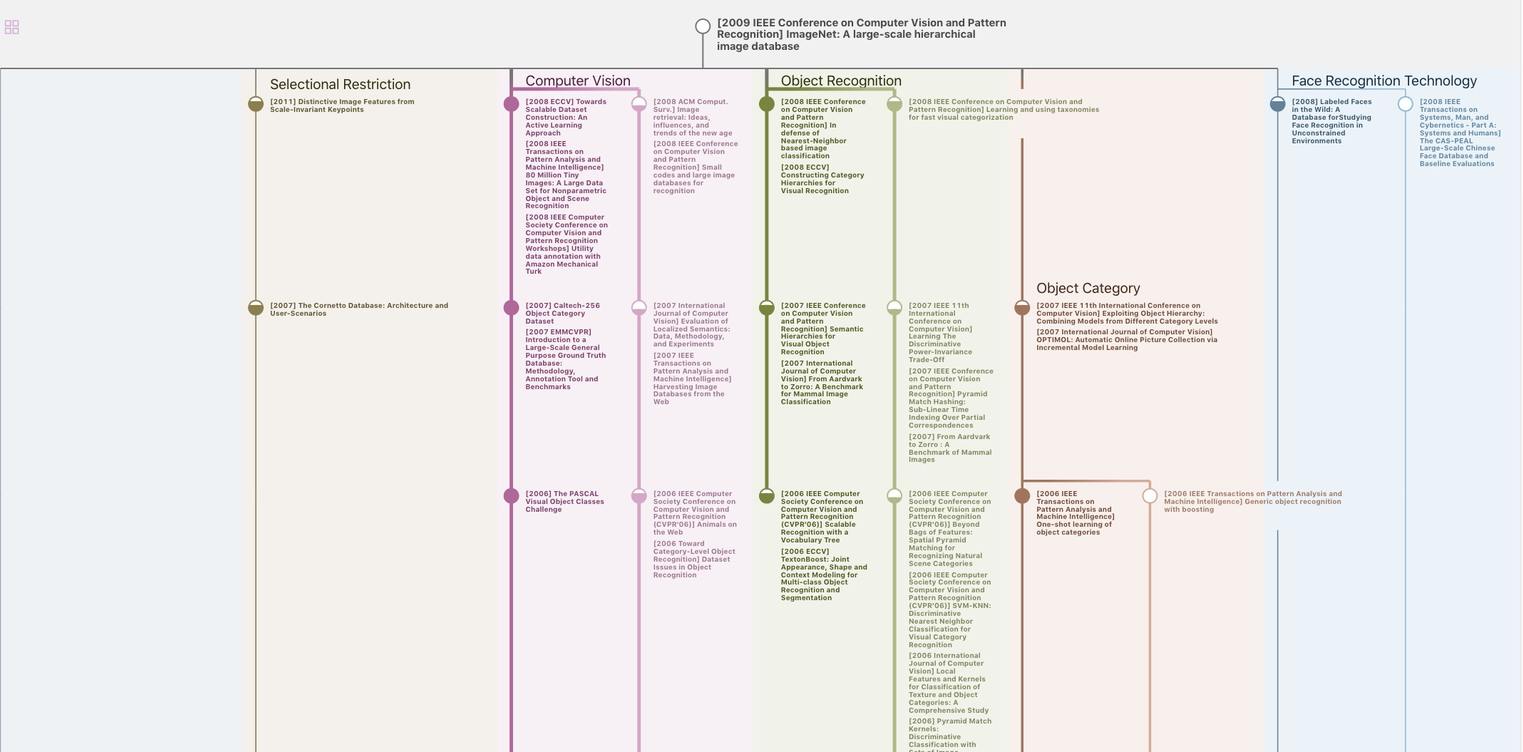
生成溯源树,研究论文发展脉络
Chat Paper
正在生成论文摘要