From Data to Insight: Predicting Interstitial Glucose in Healthy Cohort with Non-invasive Sensor Technology and Machine Learning
Research Square (Research Square)(2023)
摘要
Continuous glucose monitoring (CGM) has transformed the care of diabetes mellitus patients. It is increasingly used to support nutritional management in various pathophysiologic conditions, including obesity and migraine, where avoiding postprandial hyperglycemia is critical to prevent hyperinsulinemia and low glucose levels after meal intake. However, the current CGM devices have significant limitations, such as invasiveness, availability, high cost, and limited shelf life, which must be overcome for broader use, specifically in disease prevention and obesity management. Therefore, we collected over 15,500 interstitial glucose measurements by CGM with a large amount of corresponding multimodal non-invasive wearable sensor data for healthy cohorts and used the machine learning (ML) approach to discover their correlations and accurately predict the postprandial glucose response (PPGR) in real-time over ten days, with an average Root Mean Squared Error (RMSE) of 18.49 ± 0.1 mg/dL and an average Mean Absolute Percentage Error (MAPE) of 15.58 ± 0.09%. It demonstrated that ML could facilitate non-invasive blood glucose measurements and opened new opportunities for efficient and objective nutritional-based disease prevention.
更多查看译文
关键词
interstitial glucose,machine learning,predicting,healthy cohort,non-invasive
AI 理解论文
溯源树
样例
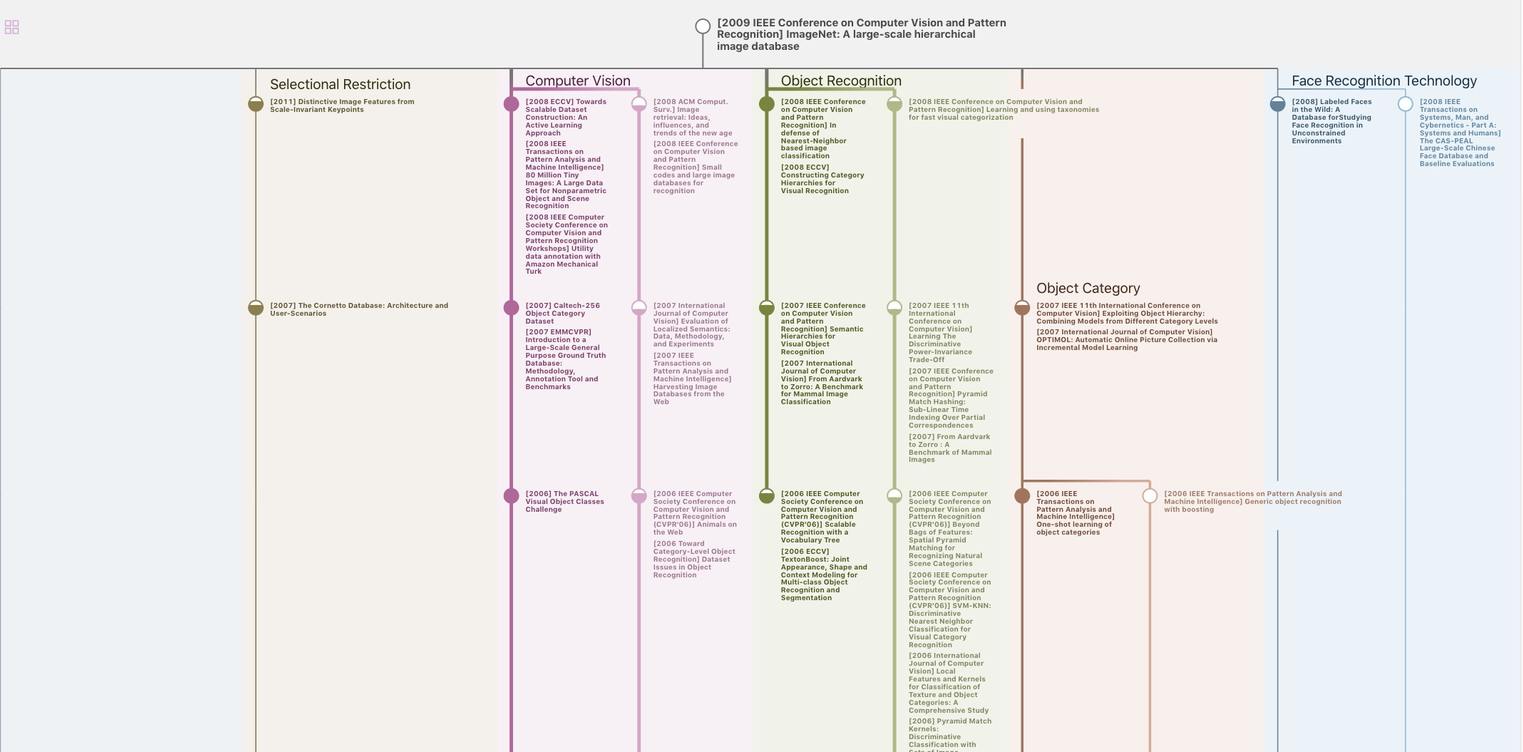
生成溯源树,研究论文发展脉络
Chat Paper
正在生成论文摘要