HEaaN-STAT: a privacy-preserving statistical analysis toolkit for large-scale numerical, ordinal, and categorical data
IEEE Transactions on Dependable and Secure Computing(2023)
摘要
Statistical analysis of largescale data is useful as it enables the extraction of a large amount of information, despite its simplicity. Therefore, fusing and analyzing data from different security domains is an attractive and promising approach, unless it jeopardizes the privacy of the data in any security domain. In this study, we proposed the HEaaN-STAT toolkit that can efficiently fuse data from different domains to enable largescale statistical analysis while protecting data privacy. Moreover, we proposed an efficient inverse operation and a table lookup function for Cheon-Kim-Kim-Song (CKKS) encrypted data, as well as a data encoding method for counting encrypted data. Based on this, we proposed a method for generating a contingency table with a large number of cases and k-percentile for largescale data that is hundreds to thousands of times faster than the method proposed by Lu et al. in NDSS' 17. The validity of the proposed toolkit was verified through practical use for business applications using real-world data.
更多查看译文
关键词
Homomorphic encryption,Privacy preserving statistical data analysis,Applied cryptography,Information security
AI 理解论文
溯源树
样例
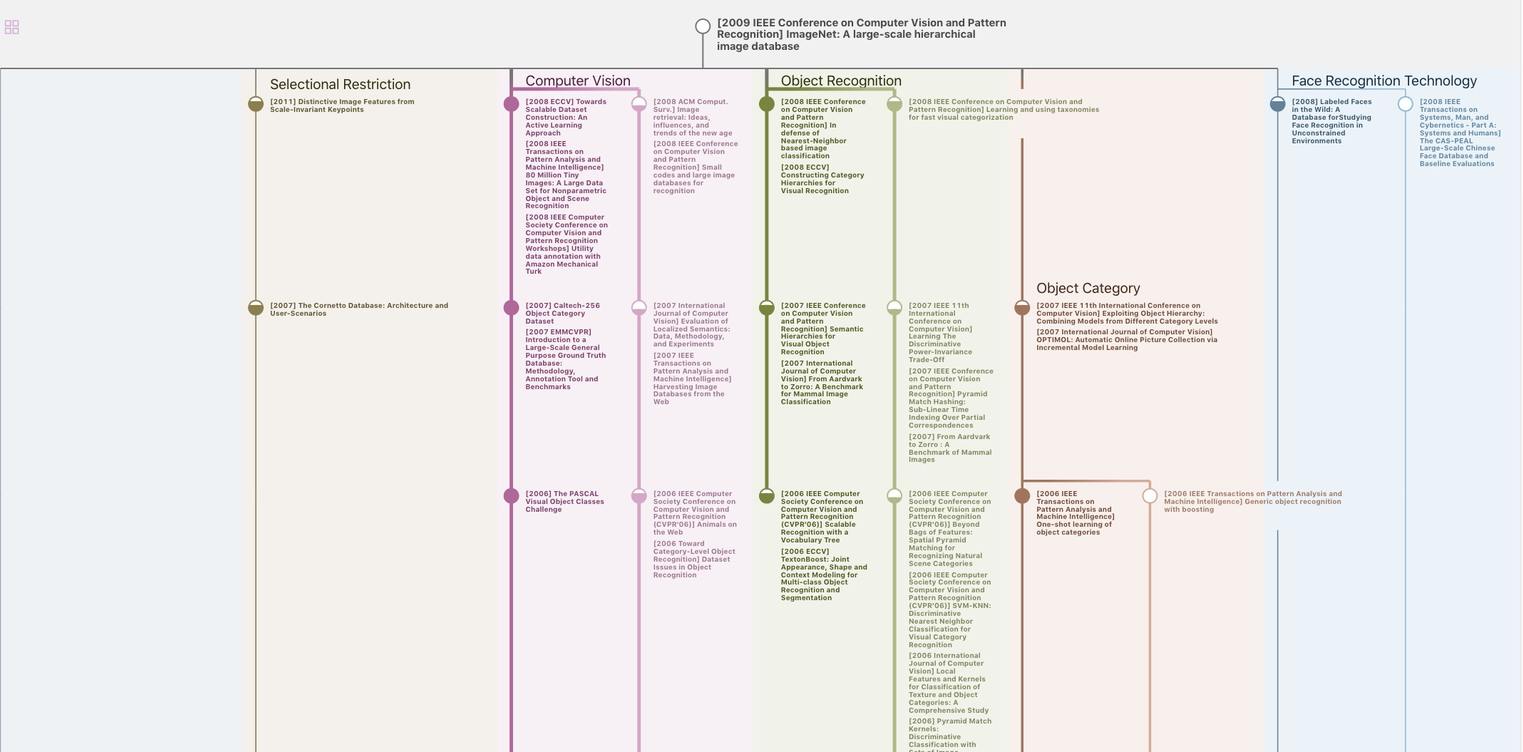
生成溯源树,研究论文发展脉络
Chat Paper
正在生成论文摘要