Deconvolution of ex-vivo drug screening data and bulk tissue expression predicts the abundance and viability of cancer cell subpopulations
bioRxiv (Cold Spring Harbor Laboratory)(2023)
摘要
Abstract Ex-vivo drug sensitivity screening (DSS) allows the prediction of cancer treatment effectiveness in a personalized fashion. However, it only provides a readout on mixtures of cells, potentially occulting important information on clinically relevant cell subtypes. To address this shortcoming, we developed a machine-learning framework to deconvolute bulk RNA expression matched with bulk drug sensitivity into cell subtype composition and cell subtype drug sensitivity. We first determined that our method could decipher the cellular composition of bulk samples with top-ranking accuracy compared to state-of-the-art deconvolution methods. We then optimized an algorithm capable of estimating cell subtype- and single-cell-specific drug sensitivity, which we evaluated by performing in-vitro drug studies and in-depth simulations. We then applied our deconvolution strategy to Acute Myeloid Leukemia (AML) context using the beatAML cohort dataset, currently the most extensive database of ex-vivo DSS. We generated a landscape of cell subtype-specific drug sensitivity and focused on four therapeutic compounds predicted to target leukemic stem cells: crenalotinib, AZD1480, bosutinib, and venetoclax. We defined their efficacy at the single-cell level and characterized a population of venetoclax-resistant cancer stem-like cells. Our work provides an attractive new computational tool for drug development and precision medicine.
更多查看译文
关键词
bulk tissue expression,screening,cancer,ex-vivo
AI 理解论文
溯源树
样例
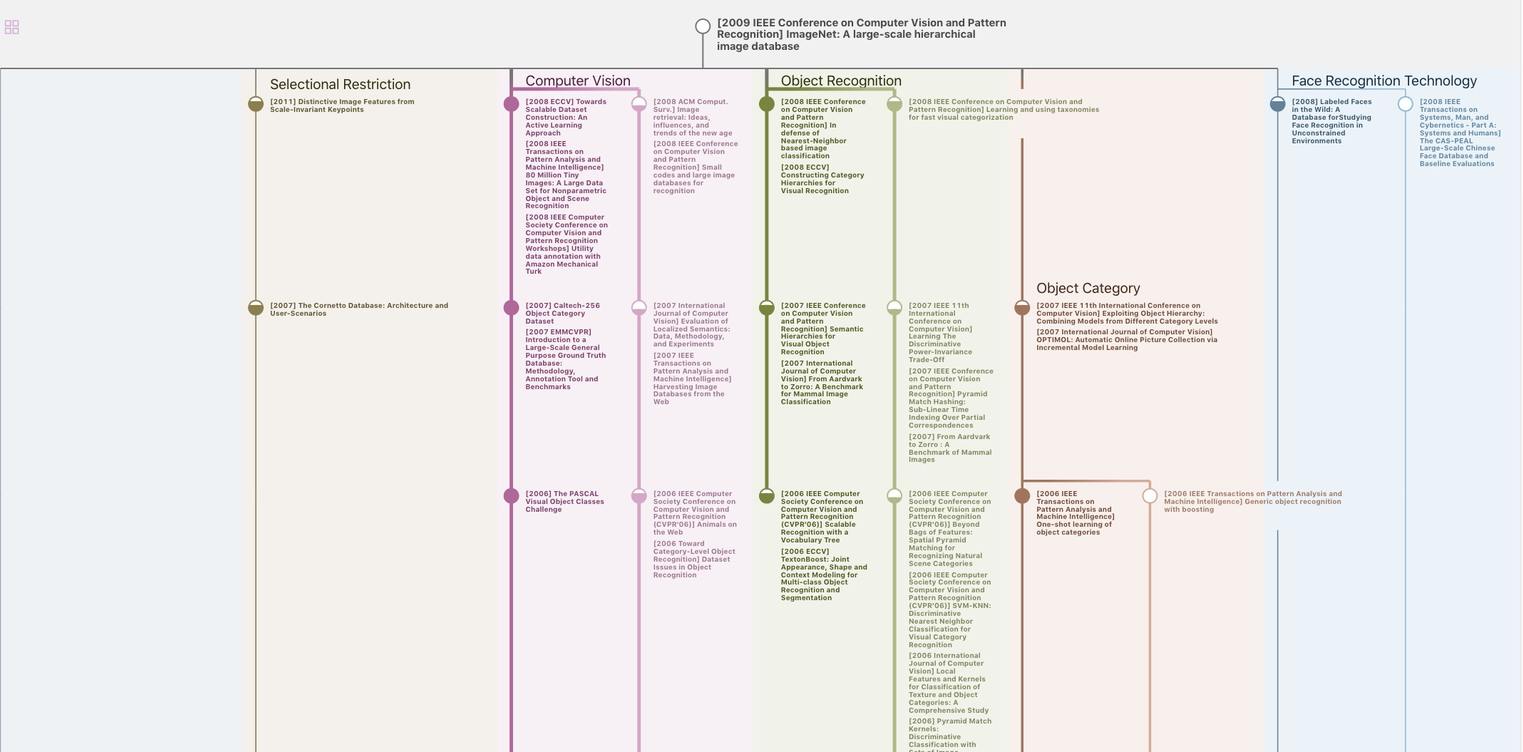
生成溯源树,研究论文发展脉络
Chat Paper
正在生成论文摘要