Attention Residual Capsule Network for Dermoscopy Image Classification
Communications in computer and information science(2023)
摘要
Automated analysis of dermoscopic images for detecting malignant lesions can improve diagnostic performance and reduce premature deaths. While several automated classification algorithms using deep convolutional neural network (DCNN) models have been proposed, the need for performance improvement remains. The key limitations of developing a robust DCNN model for the dermoscopic image classification are (a) sub-sampling or pooling layer in traditional DCNN has theoretical drawbacks in capturing object-part relationship, (b) increasing the network depth can improve the performance but is prone to suffer from the vanishing gradient problem, and (c) due to imbalanced dataset, the trained DCNN tends to be biased towards the majority classes. To overcome these limitations, we propose a novel deep Attention Residual Capsule Network (ARCN) for dermoscopic image classification to diagnose skin diseases. The proposed model combines the concept of residual learning, self-attention mechanism, and capsule network. The residual learning is employed to address the vanishing gradient problem, the self-attention mechanism is employed to prioritize important features without using any extra learnable parameters, capsule network is employed to cope up with information loss due to the sub-sampling (max-pooling) layer. To deal with the classifier’s bias toward the majority classes, a novel Mini-Batch-wise weight-balancing Focal Loss strategy is proposed. HAM10000, a benchmark dataset of dermoscopic images is used to train the deep model and evaluate the performance. The ARCN-18 (modification of ResNet-18) network trained with the proposed loss produces an accuracy of 0.8206 for the considered test set.
更多查看译文
关键词
attention residual capsule network,dermoscopy image classification
AI 理解论文
溯源树
样例
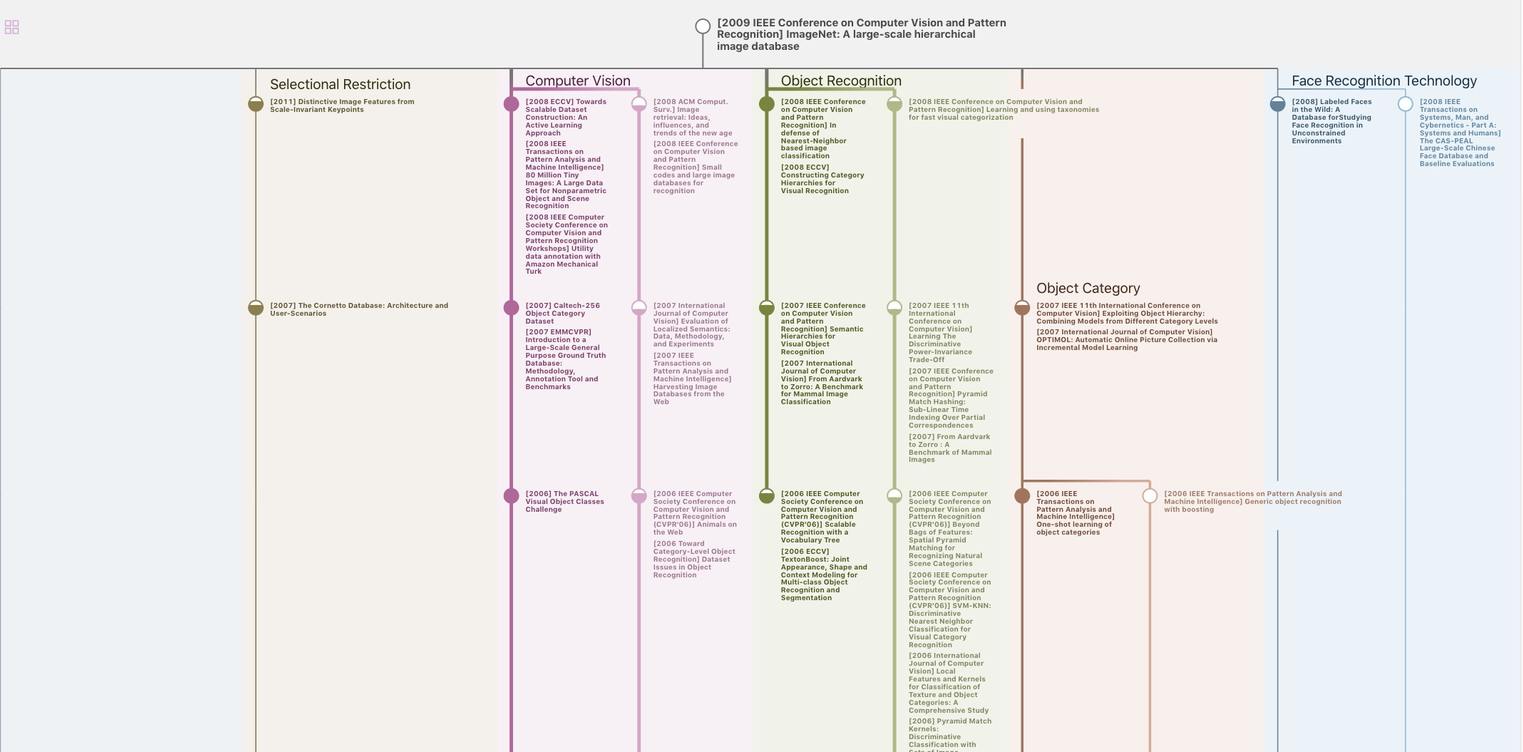
生成溯源树,研究论文发展脉络
Chat Paper
正在生成论文摘要