Semi-Supervised Medical Report Generation via Graph-Guided Hybrid Feature Consistency
IEEE TRANSACTIONS ON MULTIMEDIA(2024)
摘要
Medical report generation generates the corresponding report according to the given radiology image, which has been attracting increasing research interest. However, existing methods mainly adopt supervised training which rely on large amount of medical reports that are actually unavailable owing to the labor-intensive labeling process and privacy protection protocol. In the meanwhile, the intrinsic relationships between local pathological changes in the image are often ignored, which actually are important hints to high quality report generation. To this end, we propose a Relation-Aware Mean Teacher (RAMT) framework, which follows a standard mean teacher paradigm for semi-supervised report generation. The key to the encoder of the backbone network is the Graph-guided Hybrid Feature Encoding (GHFE) module, which exploits a prior disease knowledge graph to encode the intrinsic relations between pathological changes into the graph embedding and learns a word dictionary to retrieve the semantic embedding for each potential pathological change. GHFE combines the graph embedding, semantic embedding and visual features to form hybrid features, which are sent to a Transformer-based decoder for report generation. Extensive experiments on the MIMIC-CXR and IU X-Ray datasets demonstrate the effectiveness of our proposed approach.
更多查看译文
关键词
Knowledge graph,mean teacher,medical report generation,semi-supervised learning
AI 理解论文
溯源树
样例
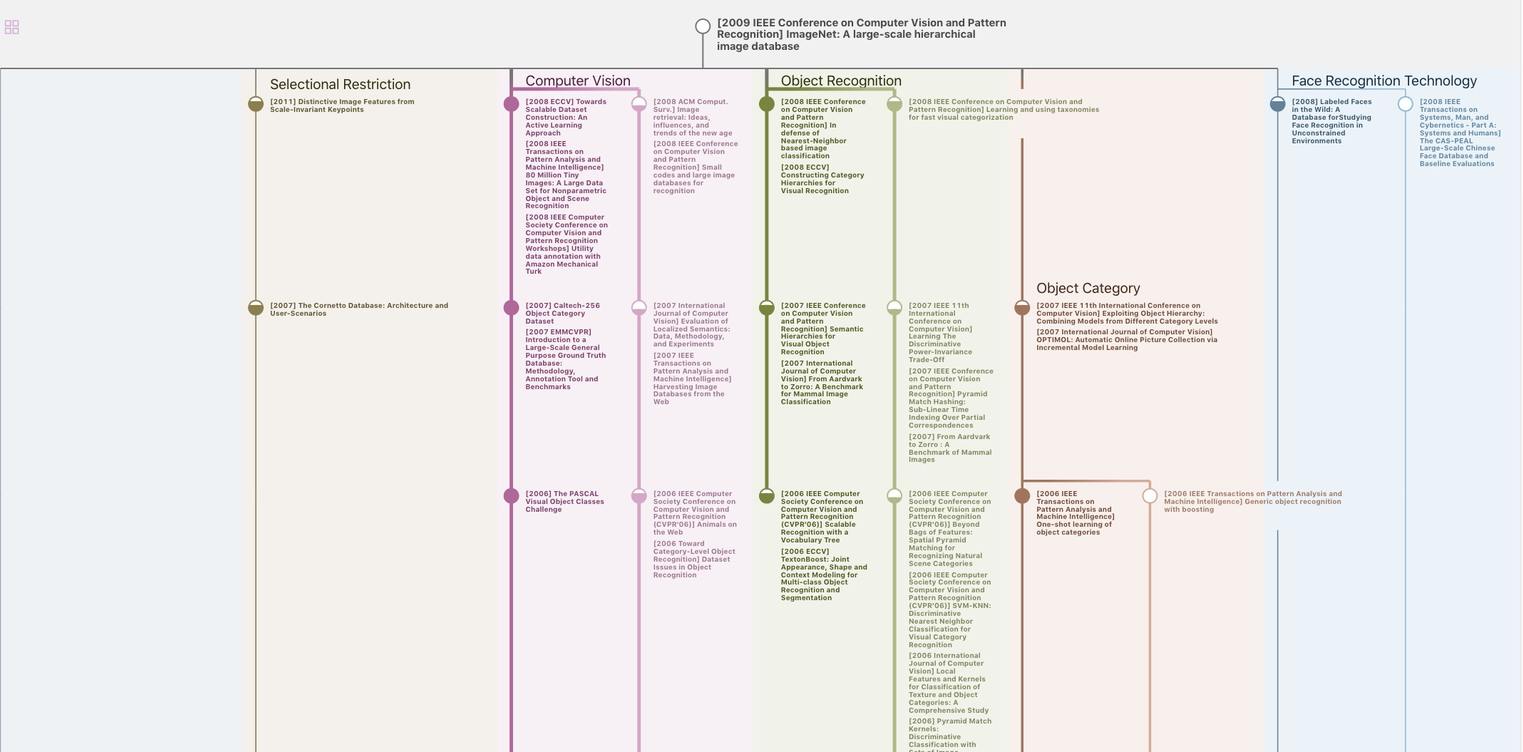
生成溯源树,研究论文发展脉络
Chat Paper
正在生成论文摘要