Prior-Aware Cross Modality Augmentation Learning for Continuous Sign Language Recognition
IEEE TRANSACTIONS ON MULTIMEDIA(2024)
Abstract
Continuous sign language recognition (CSLR) aims to map a sign video into a sentence of text words in the same order as the signs. Generally, word error rate (WER), i.e., editing distance, is adopted as the main evaluation metric. Since this metric is not differentiable, current deep-learning-based CSLR methods usually resort to connectionist temporal classification (CTC) loss during optimization, which maximizes the posterior probability over the sequential alignment. Due to the optimization gap between CTC loss and WER, the decoded sequence with the maximum probability in CTC may not be the one with the lowest WER. To tackle this issue, we propose a novel prior-aware cross modality augmentation learning method. In our approach, we first generate the pseudo video-text pair by cross modality editing, i.e., substitution, deletion and insertion on the paired real video-text data. To ensure the pseudo data quality, we guide the editing with both textual grammar prior and visual pose transition consistency prior. In this way, the generated pseudo video and text sentence follow the underlying distribution of the sign language data, and sever as more genuine hard examples for the cross modality representation learning of our CSLR task. Based on the real and generated pseudo data, we optimize our CSLR framework with three loss terms. We evaluate our approach on popular large-scale CSLR datasets and extensive experiments demonstrate the effectiveness of our method.
MoreTranslated text
Key words
Cross modality augmentation learning,editing with prior incorporated,continuous sign language recognition
AI Read Science
Must-Reading Tree
Example
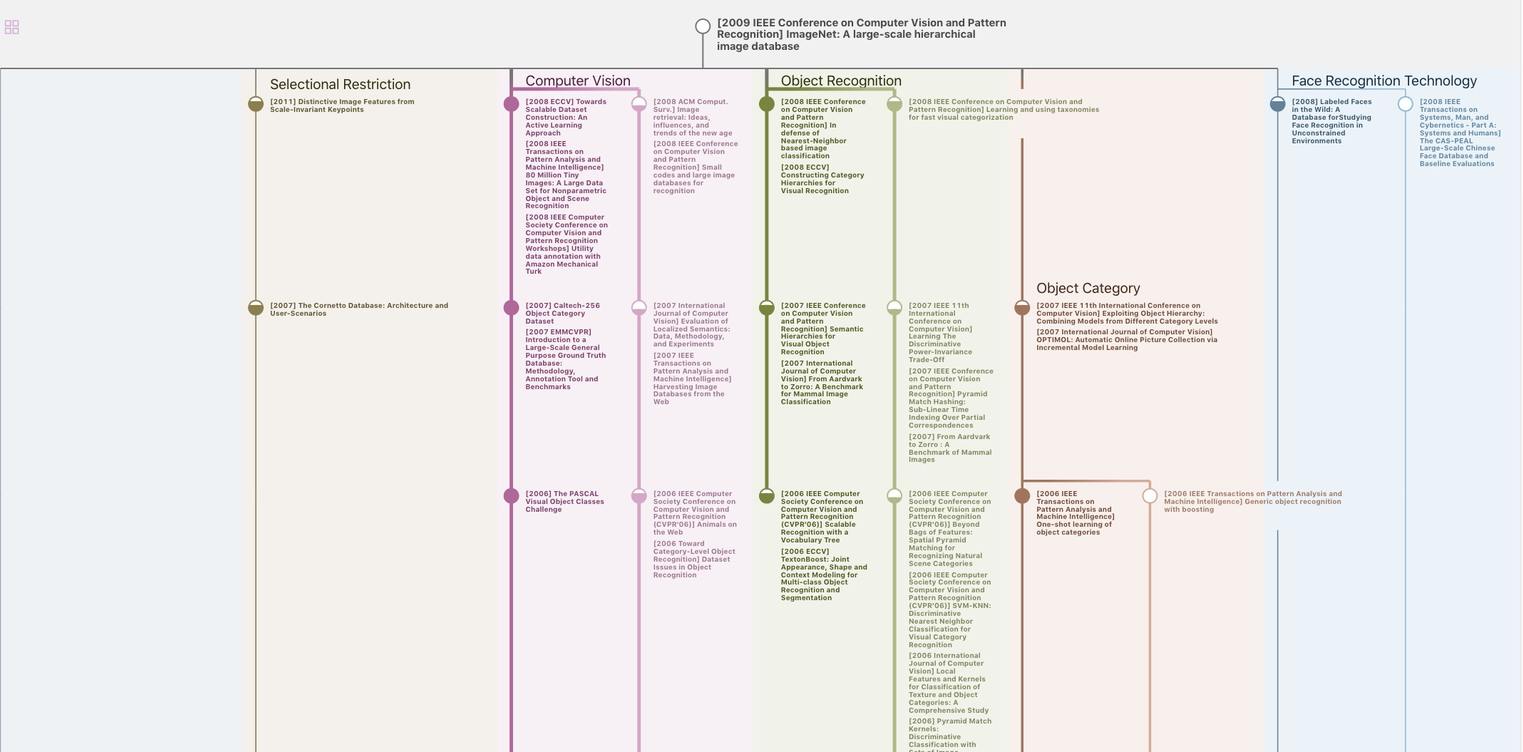
Generate MRT to find the research sequence of this paper
Chat Paper
Summary is being generated by the instructions you defined