Fault?fracture reservoir identification method based on SRGAN and reconstructed super?resolution seismic signals
Geophysics(2023)
摘要
To address the problem of fault?fracture reservoir identification, a new method based on super?resolution (SR) seismic signal reconstruction is established to identify faults, sliding fracture zones and induced fracture zones. First, based on a super?resolution generation countermeasure (SRGAN) deep learning method, an SR seismic signal reconstruction network framework is designed with a discriminant network (D), a generation network (G) and a visual geometry group network (V). Through the perceptual loss, objective control functions and iterative parameter updates, the nonlinear feature learning advantages of the deep network are introduced, the noise is eliminated, weak signals are recovered, and low resolution (LR) signals are restored, allowing the seismic signal to be reconstructed into an SR signal. Second, the SR seismic signal is used to extract the geometric attributes, such as the coherence based on the gradient structure tensor (GST) and the curvature based on the fractional derivative approximation (FDA). Third, principal component analysis (PCA) is used to reduce the feature dimension of the seismic attributes such as the GST coherence and FDA curvature and extract the principal components with the strongest correlations, thus eliminating redundant and residual noise interference, highlighting the spatial distribution and internal details of the fault?fracture reservoir, and allowing a fine description of the fault?fracture reservoir to be developed. Finally, this method achieves a good application effect for reconstructing SR seismic signals and identifying fault?fracture reservoirs in the Sichuan Basin of China.
更多查看译文
关键词
srgan,faultfracture,reservoir
AI 理解论文
溯源树
样例
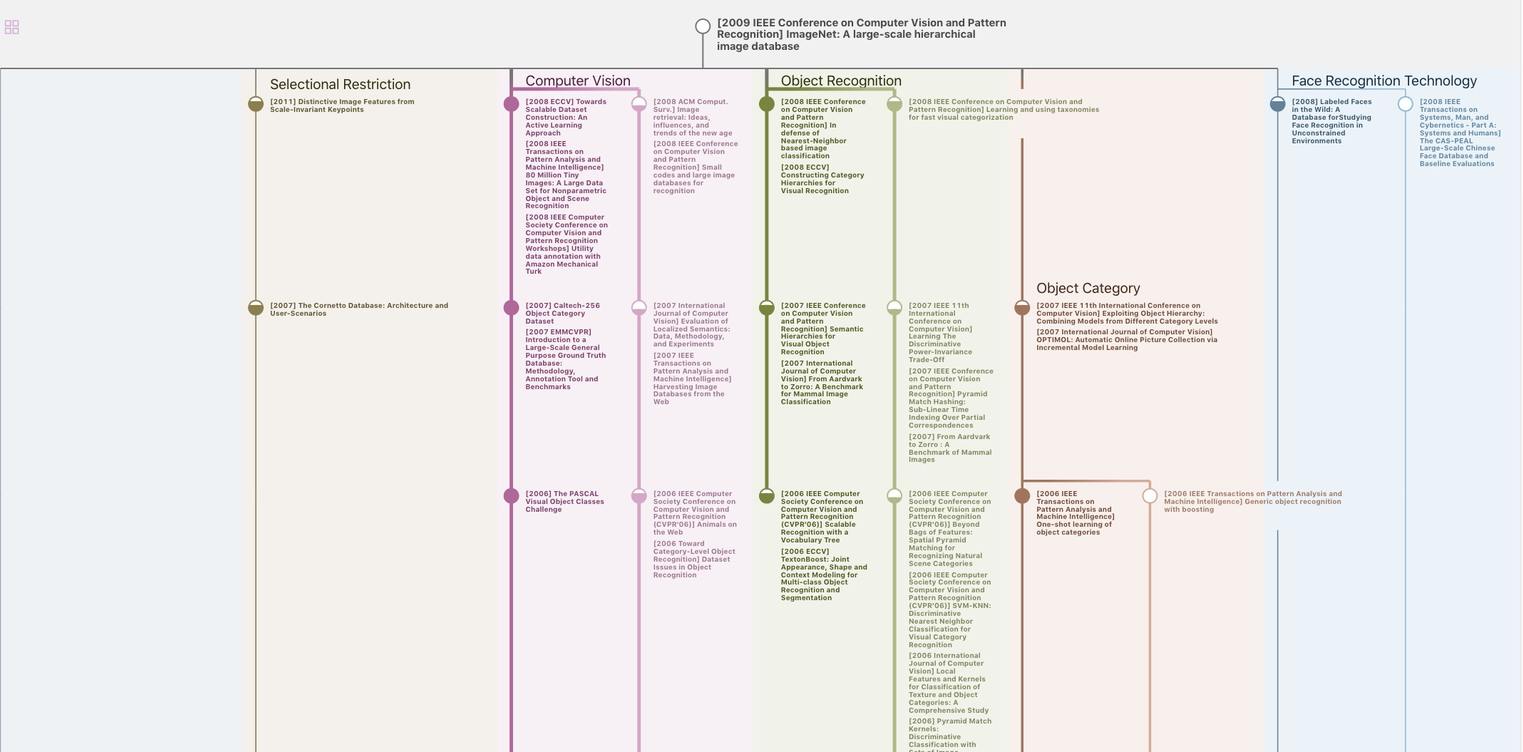
生成溯源树,研究论文发展脉络
Chat Paper
正在生成论文摘要