The Role of Preprocessing for Word Representation Learning in Affective Tasks.
IEEE Trans. Affect. Comput.(2024)
摘要
Affective tasks, including sentiment analysis, emotion classification, and sarcasm detection have drawn a lot of attention in recent years due to a broad range of useful applications in various domains. The main goal of affect detection tasks is to recognize states such as mood, sentiment, and emotions from textual data (e.g., news articles or product reviews). Despite the importance of utilizing preprocessing steps in different stages (i.e., word representation learning and building a classification model) of affect detection tasks, this topic has not been studied well. To that end, we explore whether applying various preprocessing methods (stemming, lemmatization, stopword removal, punctuation removal and so on) and their combinations in different stages of the affect detection pipeline can improve the model performance. The are many preprocessing approaches that can be utilized in affect detection tasks. However, their influence on the final performance depends on the type of preprocessing and the stages that they are applied. Moreover, the preprocessing impacts vary across different affective tasks. Our analysis provides thorough insights into how preprocessing steps can be applied in building an effect detection pipeline and their respective influence on performance.
更多查看译文
关键词
Affective tasks,emotion classification,language representation,pretrained language models,sarcasm detection,sentiment analysis,text preprocessing,word embeddings,word representation
AI 理解论文
溯源树
样例
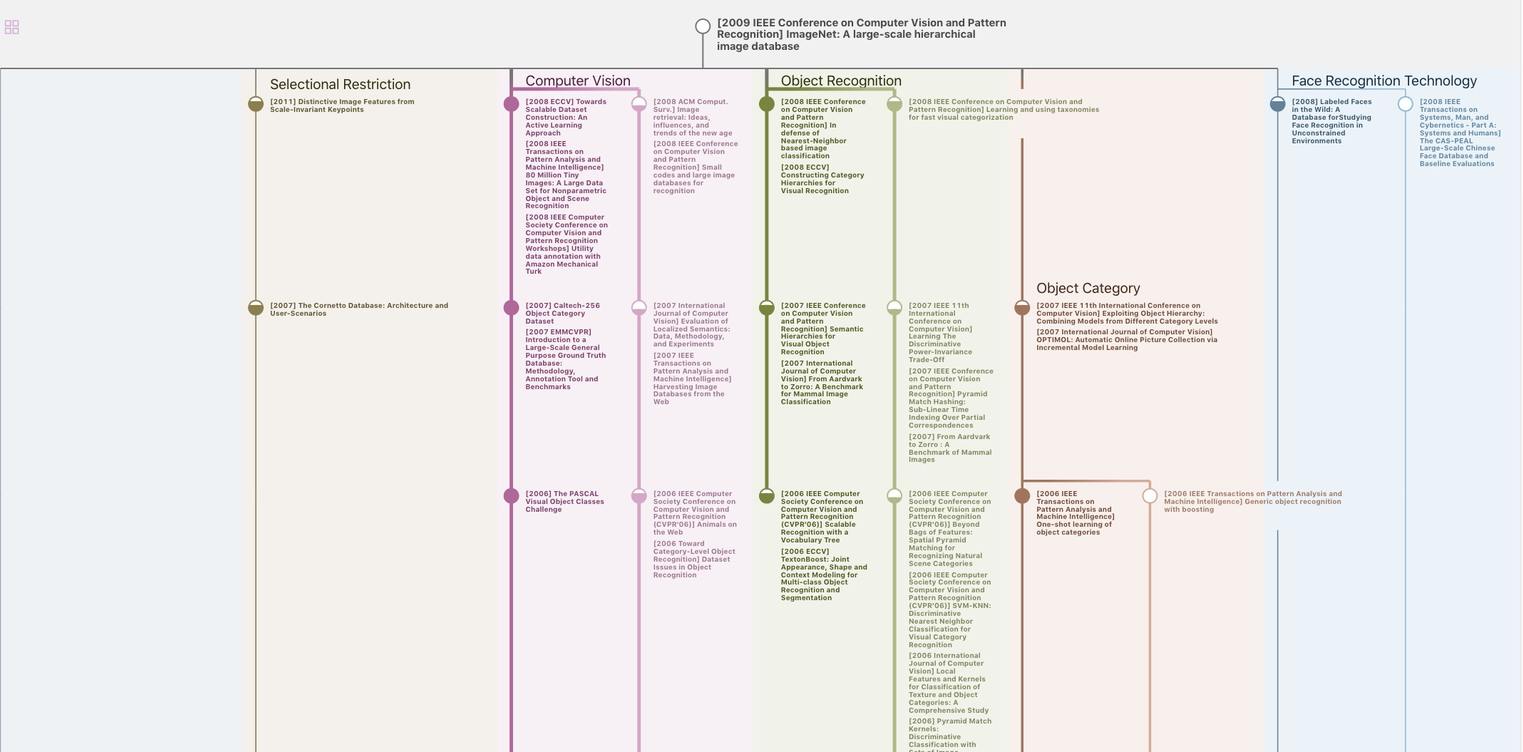
生成溯源树,研究论文发展脉络
Chat Paper
正在生成论文摘要