Knowledge transfer in multi-objective multi-agent reinforcement learning via generalized policy improvement.
Computer Science and Information Systems(2024)
摘要
Even though many real-world problems are inherently distributed and multi-objective, most of the reinforcement learning (RL) literature deals with single agents and single objectives. While some of these problems can be solved using a single-agent single-objective RL solution (e.g., by specifying preferences over objectives), there are robustness issues, as well the fact that preferences may change over time, or it might not even be possible to set such preferences. Therefore, a need arises for a way to train multiple agents for any given preference distribution over the objectives. This work thus proposes a multi-objective multi-agent reinforcement learning (MOMARL) method in which agents build a shared set of policies during training, in a decentralized way, and then combine these policies using a generalization of policy improvement and policy evaluation (fundamental operations of RL algorithms) to generate effective behaviors for any possible preference distribution, without requiring any additional training. This method is applied to two different application scenarios: a multi-agent extension of a domain commonly used in the related literature, and traffic signal control, which is more complex, inherently distributed and multi-objective (the flow of both vehicles and pedestrians are considered). Results show that the approach is able to effectively and efficiently generate behaviors for the agents, given any preference over the objectives.
更多查看译文
关键词
knowledge transfer,reinforcement learning,multi-objective,multi-agent
AI 理解论文
溯源树
样例
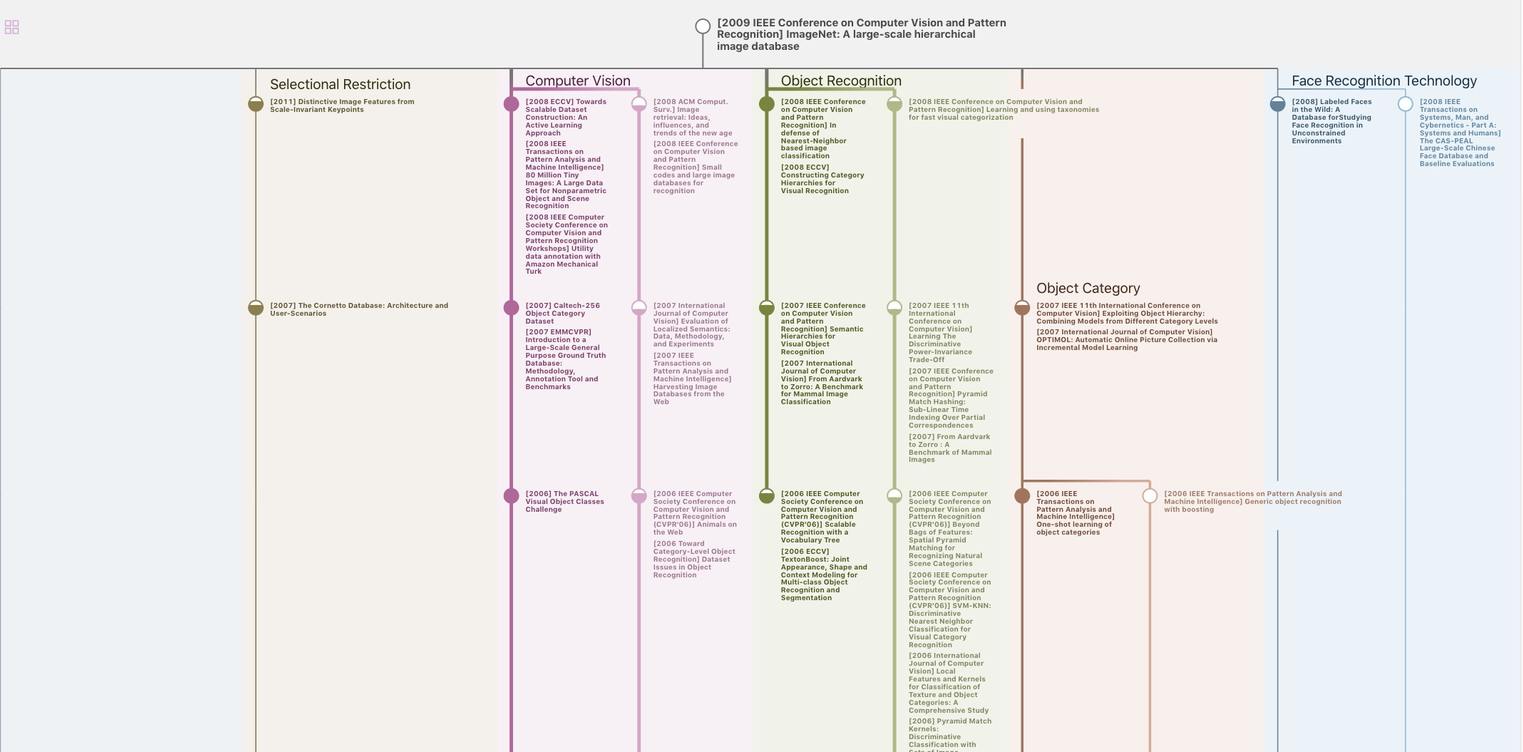
生成溯源树,研究论文发展脉络
Chat Paper
正在生成论文摘要