AL4SLEO: An Active Learning Solution for the Semantic Labelling of Earth Observation Satellite Images—Part 1
Springer tracts in nature-inspired computing(2023)
摘要
Active Learning is when a machine is interactively interrogating a user (information source) to label selected data samples with appropriate annotations. Our approach is based on Active Learning, a sub-category of supervised machine learning, where–based on user-selected representative data points and their interactive classification—the support by expert image analysts provides highly reliable image classification results for all unlabeled data points. The basic idea behind Active Learning is that a machine learning algorithm (e.g., a Support Vector Machine approach) selects some promising data from which it learns, thus achieving greater classification accuracy with fewer training points. Active Learning can also include Relevance Feedback that supports users to search for other images of interest (e.g., patches of large satellite images) in a large repository. In addition, any newly discovered classes that do not exist yet can then be defined by these expert users exploiting their specific domain knowledge; however, different experts with individual class-specific expertise might assign different labels to the new classes. We assumed that there is already a list of predefined semantic labels for the already known classes, but when using this method, new classes can be discovered, or some anomalies within the data can be detected and annotated. The idea behind this Active Learning method is that it is designed to obtain higher classification accuracies (depending on the given class) with very few training examples. The method can be applied to a single image, to multiple images (from different locations), to multi-temporal images (same location over a period of time), and even to multi-sensor images (same location acquired by different sensors). In this Part 1, a cascaded Active Learning method is proposed as an efficient solution to three problem cases being typical for Earth observation images: (1) Multi-label semantics, (2) Multi-sensor semantic labelling, and 3) Multi-temporal semantic labelling. In the following, the data used for demonstration was acquired by the space-borne sensors TerraSAR-X, Sentinel-1, WorldView-2, and Sentinel-2. The results obtained for the proposed cascaded Active Learning method will be given in Part 2.
更多查看译文
关键词
earth observation satellite images—part,active learning solution,semantic labelling
AI 理解论文
溯源树
样例
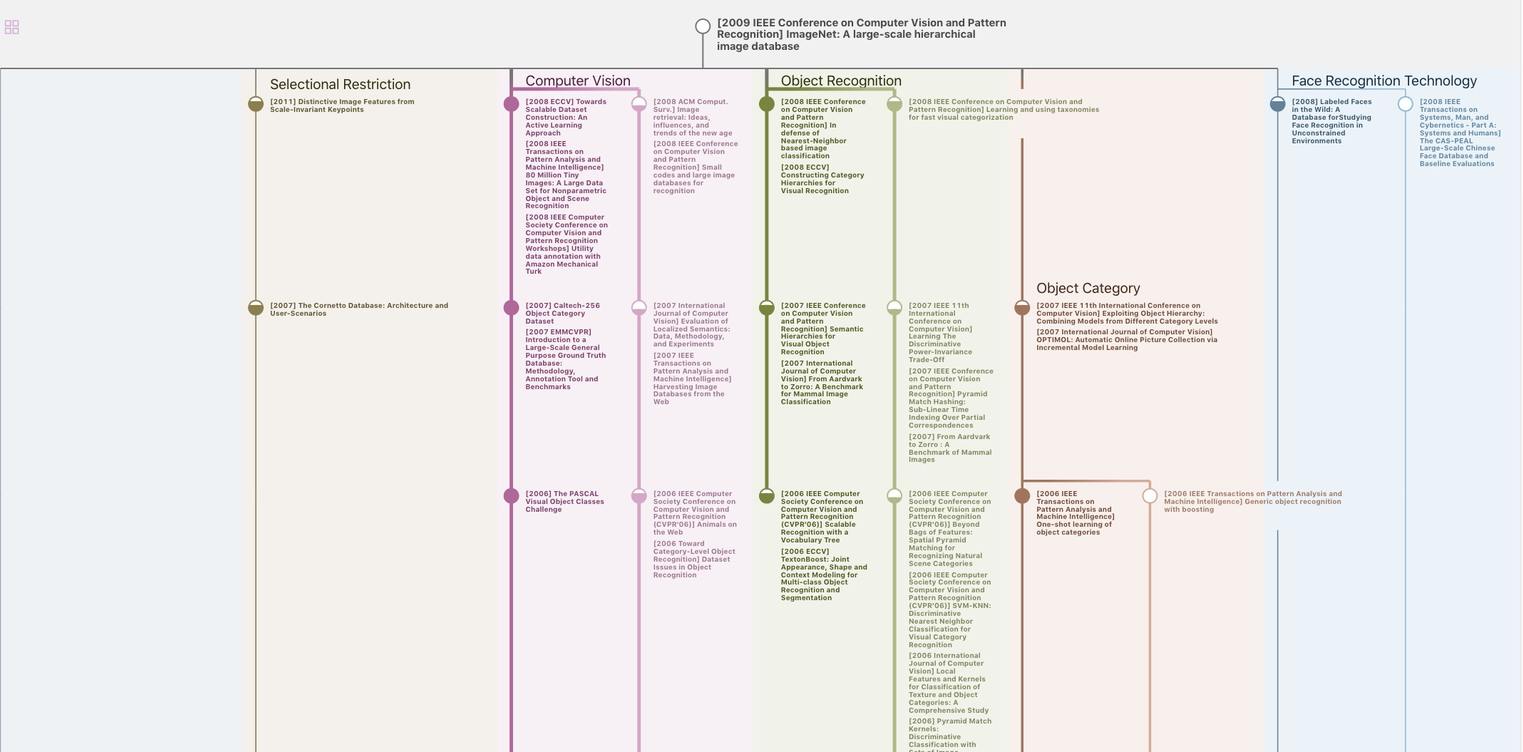
生成溯源树,研究论文发展脉络
Chat Paper
正在生成论文摘要