Accurate Throughput Prediction for Improving QoE in Mobile Adaptive Streaming.
IEEE Trans. Mob. Comput.(2024)
摘要
Video streaming is the most important mobile application today. To improve users' quality of experience (QoE), the client player runs adaptive bitrate (ABR) algorithms that dynamically select the bitrate for video chunks based on throughput or delivery time predictions. This paper aims to design an accurate predictor for mobile adaptive streaming by investigating all its components, including input features, output target, and mapping function. We construct the first theoretical framework that reveals potential factors affecting chunk throughput and delivery time. To verify this framework, we provide formulation analysis and measurement observations based on 2500+ video sessions collected in real-world mobile networks. We find that previous works have failed to achieve accurate prediction due to overlooking the impact of the transport mechanism and application behavior on throughput. Furthermore, we show that throughput is a better target for data-driven predictors than delivery time, due to the long-tailed distribution of delivery time. Based on the above, we propose Lumos, a decision-tree-based throughput predictor that can be integrated into various ABR algorithms. Extensive experiments in real-world mobile Internet show that Lumos achieves high prediction accuracy and improves the QoE of MPC by 6.3%, and MPC+Lumos outperforms Pensieve by 19.2%.
更多查看译文
关键词
Adaptive streaming,mobile internet,QoE improvement,throughput prediction
AI 理解论文
溯源树
样例
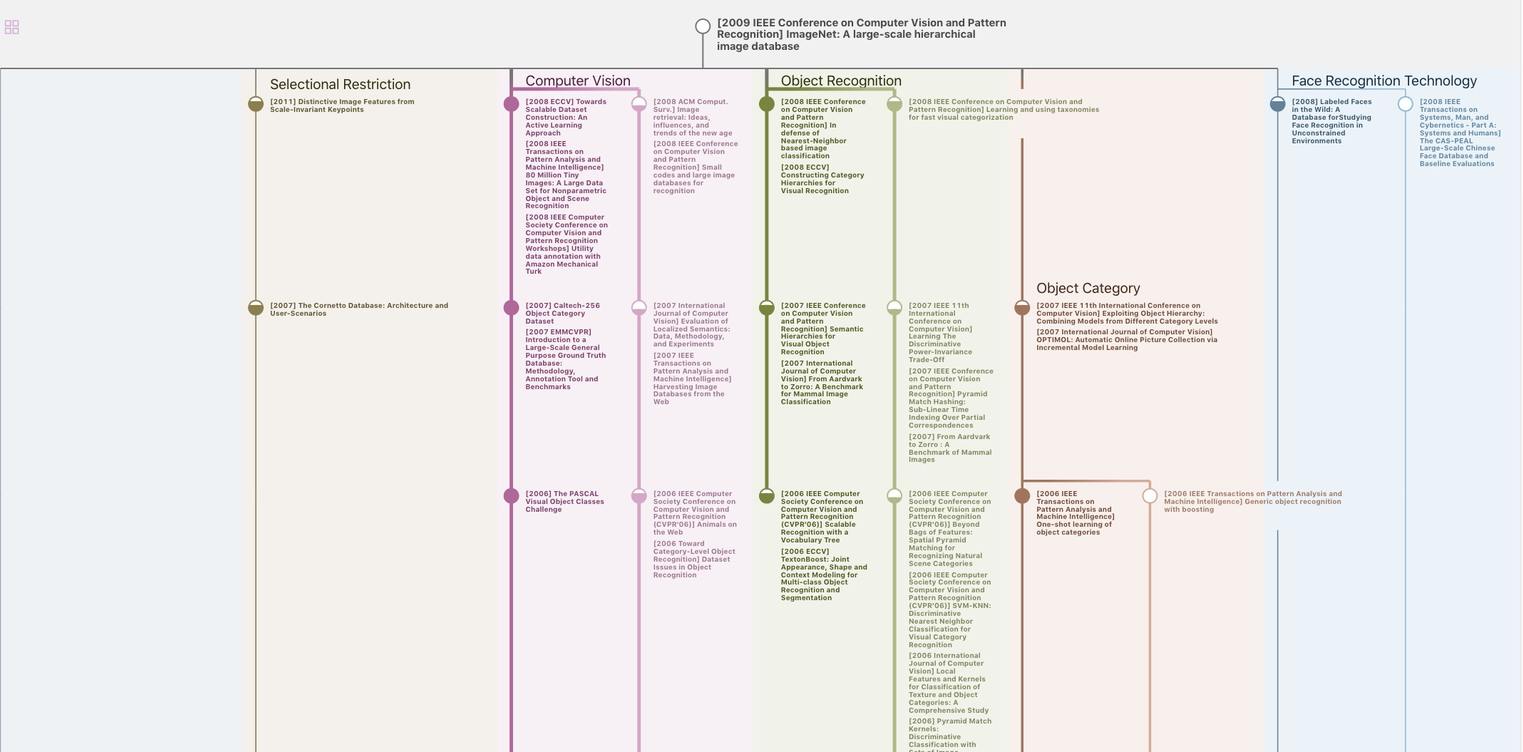
生成溯源树,研究论文发展脉络
Chat Paper
正在生成论文摘要