Learning-Based Query Scheduling and Resource Allocation for Low-Latency Mobile-Edge Video Analytics.
IEEE Internet Things J.(2024)
摘要
Mobile edge computing can help enable low-latency and accurate video analytics. However, it is difficult to make efficient utilization of limited edge resources because of the diverse requirements of video queries. In this paper, we investigate edge coordination for resource-efficient video query processing, in order to accommodate real-time queries on end cameras, edge nodes, or the cloud, with accuracy guarantee. This problem is challenging because 1) video queries are with unpredictable arrivals and different resource demands, 2) the decision space of both query scheduling and resource allocation varies over time, and 3) it is critical to maintain long-term accurate analytics for all arrived queries. This problem boils down to making scheduling and resource allocation decisions, which is formulated as a mixed-integer non-linear programming with a long-term accuracy constraint. Observing that both the scheduling and resource allocation of each query have Markovian property, Markov decision process and Lyapunov optimization are adopted to decompose the problem into sequential subproblems. An adaptive reinforcement learning-based approach relying on edge coordination is proposed. Extensive experimental results show that our proposal outperforms other benchmarks on latency and accuracy at a higher level of resource utilization efficiency in real-world datasets.
更多查看译文
关键词
Video analytics,low-latency,mobile edge computing,task scheduling,resource allocation
AI 理解论文
溯源树
样例
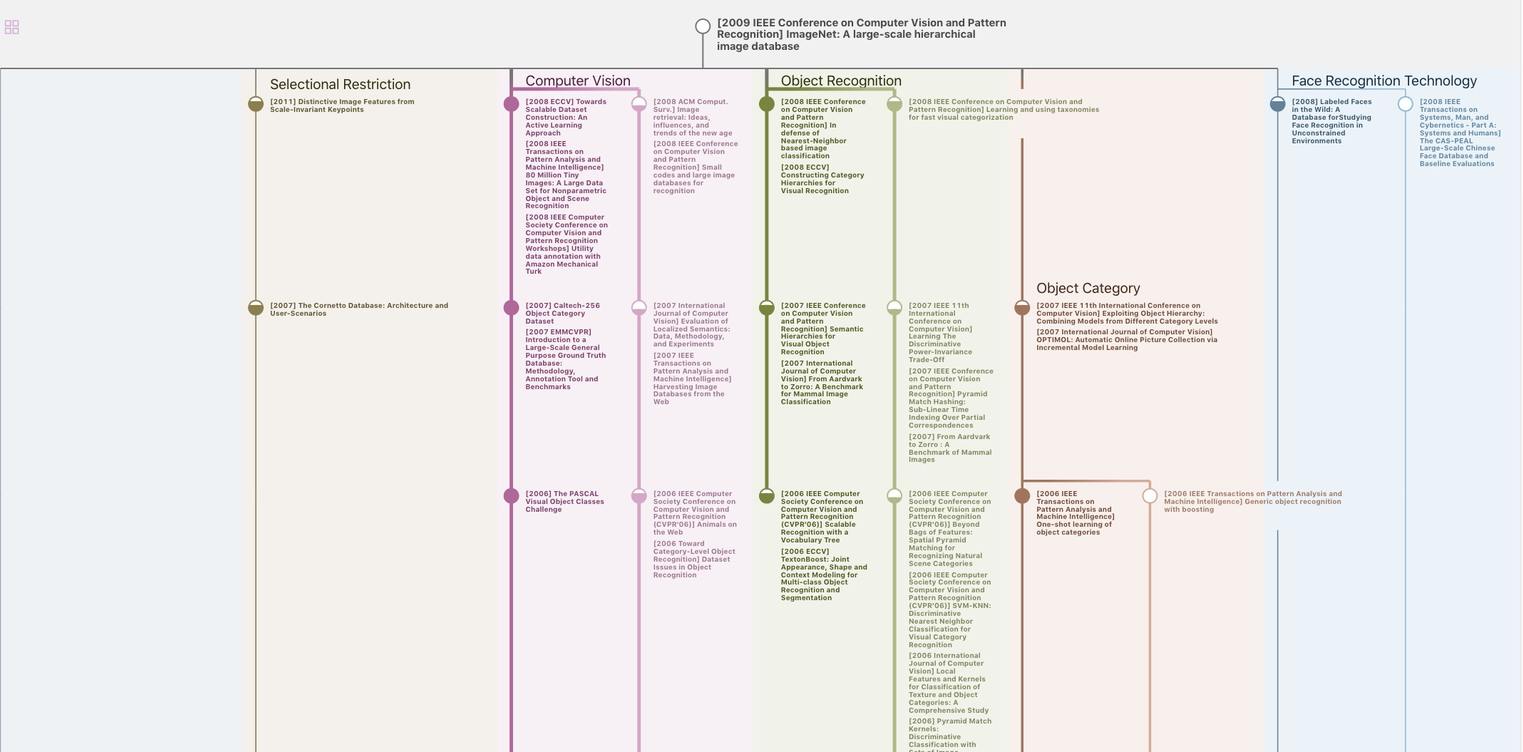
生成溯源树,研究论文发展脉络
Chat Paper
正在生成论文摘要