Rate-Adaptive Neural Network for Image Compressive Sensing.
IEEE Trans. Multim.(2024)
摘要
Deep learning-based image compressive sensing (CS) methods have achieved great success in the past few years. However, most of them are content-independent, with a spatially uniform sampling rate allocation for the entire image. Such practises may potentially degrade the performance of image CS with block-based sampling, since the content of different blocks in an image is different. In this paper, we propose a novel rate-adaptive image CS neural network (dubbed RACSNet) to achieve adaptive sampling rate allocation based on the content characteristics of the image with a single model. Specifically, a measurement domain-based reconstruction distortion is first used to guide the sampling rate allocation for different blocks in an image without access to the ground truth image. Then, a step-wise training strategy is designed to train a reusable sampling matrix, which is capable of sampling image blocks to generate the compressed measurements under arbitrary sampling rates. Subsequently, a pyramid-shaped initial reconstruction sub-network and a hierarchical deep reconstruction sub-network that fuse the measurement information of different scales are put forward to reconstruct image blocks from the compressed measurements. Finally, a reconstruction distortion map and an improved loss function are developed to eliminate the blocking artifacts and further enhance the CS reconstruction. Experimental results on both objective metrics and subjective visual qualities show that the proposed RACSNet achieves significant improvements over the state-of-the-art methods.
更多查看译文
关键词
Image compressive sensing,adaptive sampling,sampling matrix,deep networks,image compression
AI 理解论文
溯源树
样例
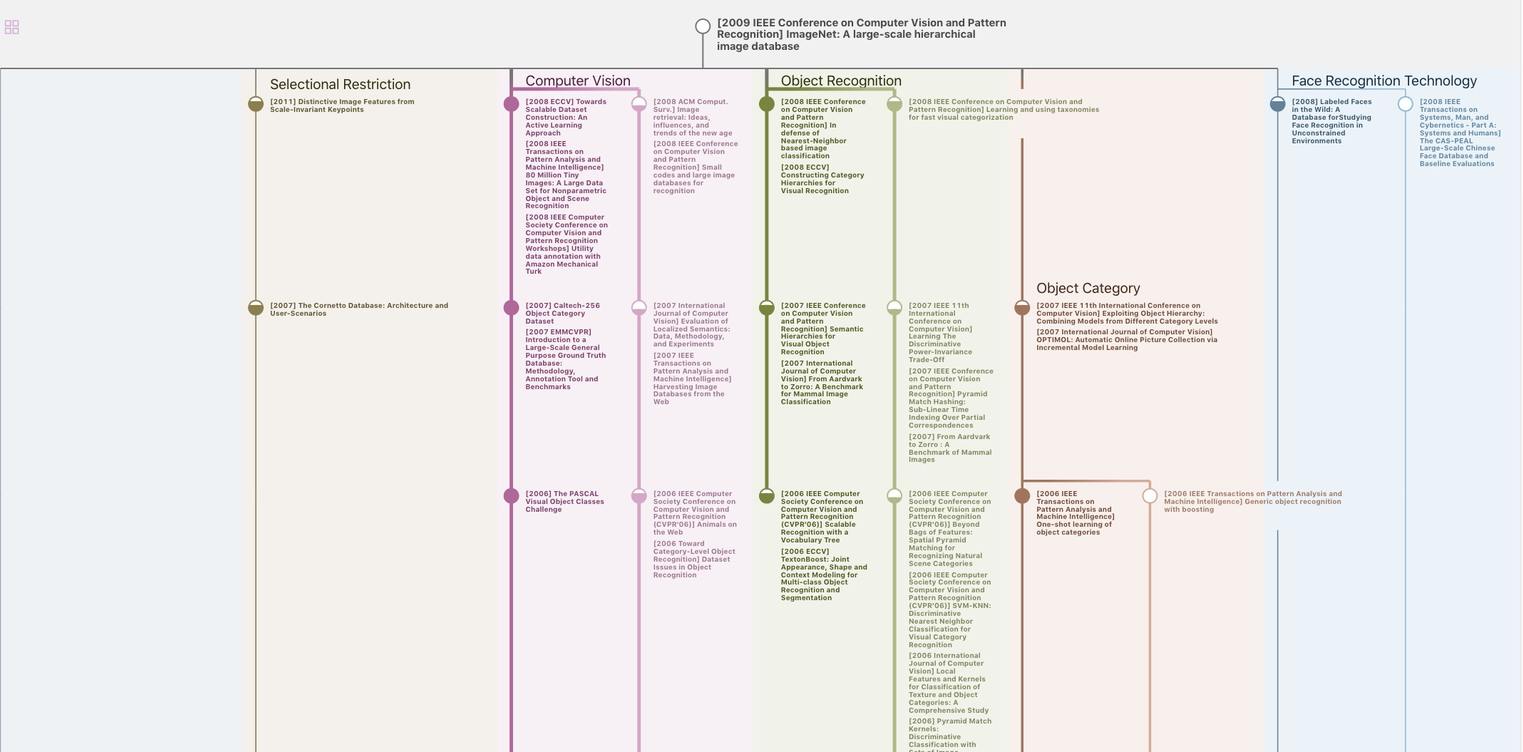
生成溯源树,研究论文发展脉络
Chat Paper
正在生成论文摘要