pFedEff: An Efficient and Personalized Federated Cognitive Learning Framework in Multiagent Systems.
IEEE Transactions on Cognitive and Developmental Systems(2024)
摘要
With the increase in data volume and environment complexity, real-world problems require more advanced algorithms to acquire useful information for further analysis or decision-making. Cognitive Learning effectively handles incomplete information, and multi-agent systems can provide enough data for analysis. Inspired by Distributed Machine Learning, Federated Learning has become an efficient framework for implementing Cognitive Learning algorithms in multi-agent systems while preserving user privacy. However, traditional communication optimizations on the federated learning framework suffer from either large communication volumes or large accuracy loss. In this paper, we propose pFedEff, a personalized federated learning framework with efficient communication that can reduce communication volume and preserve training accuracy. pFedEff uses two magnitude masks, two importance masks, and a personalized aggregation method to reduce the model and update size while maintaining the training accuracy. Specifically, we use a pre-training magnitude mask for approximated regularization to reduce the magnitude of ineffective parameters during training. We also use a post-training magnitude mask to eliminate the low-magnitude parameters after training. Furthermore, we use uploading and downloading importance masks to reduce the communication volume in both upload and download streams. Our experimental results show that pFedEff reduces up to 94% communication volume with only a 1% accuracy loss over other state-of-the-art federated learning algorithms. In addition, we conduct multiple ablation studies to evaluate the influence of hyperparameters in pFedEff, which shows the flexibility of pFedEff and its applicability in different scenarios.
更多查看译文
关键词
Multi-agent System,Cognitive Learning,Personalized Federated Learning,Model Pruning
AI 理解论文
溯源树
样例
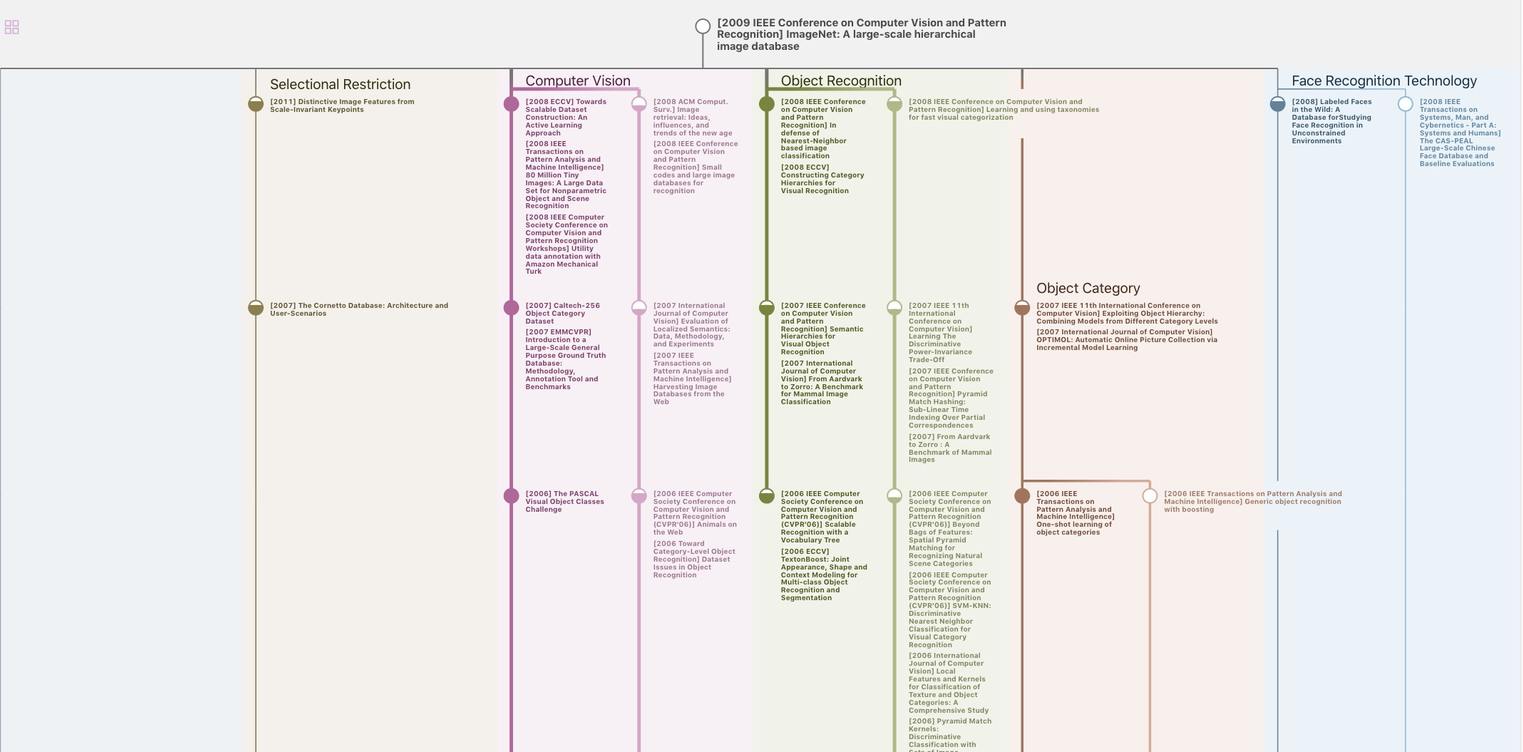
生成溯源树,研究论文发展脉络
Chat Paper
正在生成论文摘要