Multivariate Statistical Process Control
Statistics for industry, technology, and engineering(2023)
摘要
As was discussed in Chap. 4 in Modern Statistics (Kenett et al. (Modern statistics: a computer-based approach with Python, 1st edn. Springer, Birkhäuser, 2022)), multivariate observations require special techniques for visualization and analysis. This chapter presents techniques for multivariate statistical process control (MSPC) based on the Mahalanobis T2 chart. Like in previous chapters, examples of MSPC using Python are provided. Section 4.3 introduces the reader to multivariate extensions of process capability indices. These are expansions of the capability indices presented in Chap. 2 . A special role is played in this context by the concept of multivariate tolerance regions (TR). Section 4.4 considers four scenarios for setting up and running MSPC: (1) internally derived targets, (2) using an external reference sample, (3) externally assigned targets and (4) measurements units considered as batches. These four cases cover most practical applications of MSPC. Two subsections cover the special cases of measurement units considered as batches and a variable decomposition of indices used for process monitoring. Section 4.5 is a special application of MSPC to the monitoring of bioequivalence of drug product dissolution profiles. In this application tablets manufactured by a generic drug company are compared to the original product at several dissolution times. The Food and Drug Administration allows for a gap of at most 15%, a requirement that define multivariate specification limits. We show how TR are used in such cases. More on multivariate applications in pharmaceuticals will be discussed in Chap. 6 on Quality by Design.
更多查看译文
关键词
process control
AI 理解论文
溯源树
样例
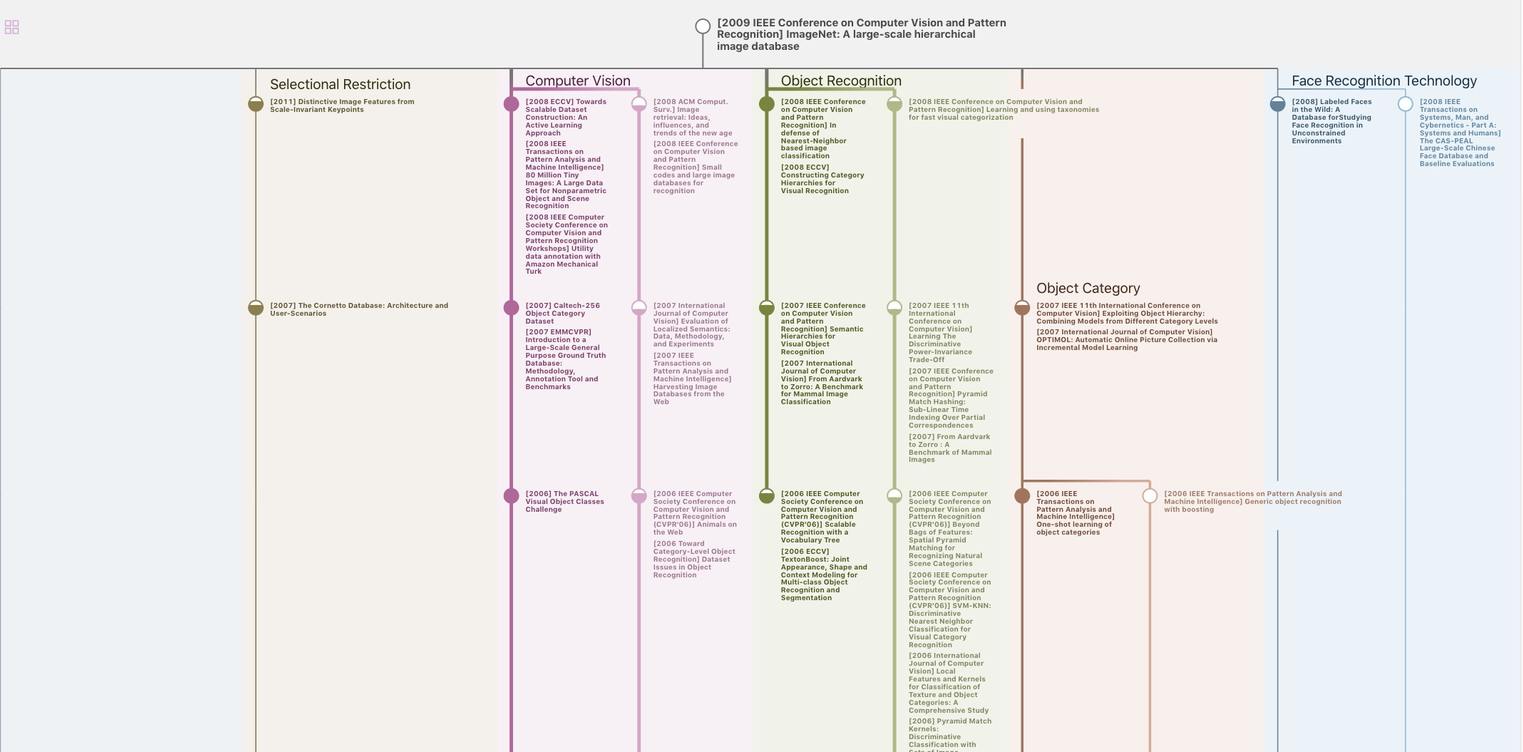
生成溯源树,研究论文发展脉络
Chat Paper
正在生成论文摘要