Abstract 4270: Prediction of cancer transcriptomes from whole-slide images with Vis-Gene
Cancer Research(2023)
摘要
Abstract Introduction: Whole slide images (WSIs) are a crucial tool used by pathologists for diagnosing and grading cancers. In recent years, deep learning techniques have revolutionized the area, helping pathologists in the detection and classification of cancers. Earlier studies have related morphological features of tissues to molecular profiles, such as mutations and gene expression, and several machine learning-based approaches have been proposed to use WSIs to predict gene expression. However, most of the established methods treat different parts of the tissues in an isolated manner, not using the spatial relation between the tiles. In this work, we proposed Vis-Gene, a deep learning approach using a vision transformer for predicting gene expression from WSIs. Methods: WSIs and RNA-seq data of five cancer types from the TCGA project were used for training and evaluation, including brain (GBM, n = 212), lung (LUAD, n = 520), kidney (KIRP, n = 295), colon (COAD, n = 290) and pancreas (PAAD, n = 180). The datasets were split into 80% for training and 20% for testing. In addition, data from healthy lung and brain tissues were obtained from the GTEx project. WSIs were split into tiles of 256 × 256 pixels, and 4,000 tiles of each image were used for training. Image features of each tile were extracted using a pre-trained Resnet-50. We clustered similar tiles using the k-Means algorithm, and the mean feature value of each cluster was used. We then used a vision transformer to “translate” image features to gene expression. To improve accuracy, we leveraged a transfer learning approach by pretraining the vision transformer on data from healthy tissues. Results: We carried out five-fold cross-validations to assess the performance of Vis-Gene in each cancer type. The root-mean-squared error (RMSE) of the top 500 most accurately predicted genes in GBM was 0.12, and the standard deviation (SD) was 0.007. The RMSE of the top 100 most accurate genes in LUAD was 0.58 (SD: 0.02), KIRP was 0.63 (SD: 0.02), COAD was 0.53 (SD: 0.03), and PAAD was 0.56 (SD: 0.04). In all the tested cancers, Vis-Gene achieved significantly lower RMSE values and higher correlation coefficients (r) compared to a baseline model and existing computational models. Gene set analysis showed that the top accurately predicted genes in GBM were related to neuropeptide signaling pathway, gliogenesis, and inflammatory response. The top accurate genes in LUAD were related to NF-kappaB signaling and regulation of cell adhesion. Using spatial transcriptomic datasets, we further validated the results of Vis-Gene in predicting intra-tumoral heterogeneity of gene expression. Conclusion: We established a new machine learning framework that can accurately predict gene expression from WSIs. This allows us to link histology features of cancers to molecular phenotype. Vis-Gene has the potential to identify clinically relevant endpoint expressions of the target genes. Citation Format: Yuanning Zheng, Marija Pizurica, Francisco Carrillo-Perez, Christian Wohlfart, Wei Yao, Nadia Shamout, Olivier Gevaert, Antoaneta Vladimirova. Prediction of cancer transcriptomes from whole-slide images with Vis-Gene. [abstract]. In: Proceedings of the American Association for Cancer Research Annual Meeting 2023; Part 1 (Regular and Invited Abstracts); 2023 Apr 14-19; Orlando, FL. Philadelphia (PA): AACR; Cancer Res 2023;83(7_Suppl):Abstract nr 4270.
更多查看译文
关键词
cancer transcriptomes,images,prediction,whole-slide,vis-gene
AI 理解论文
溯源树
样例
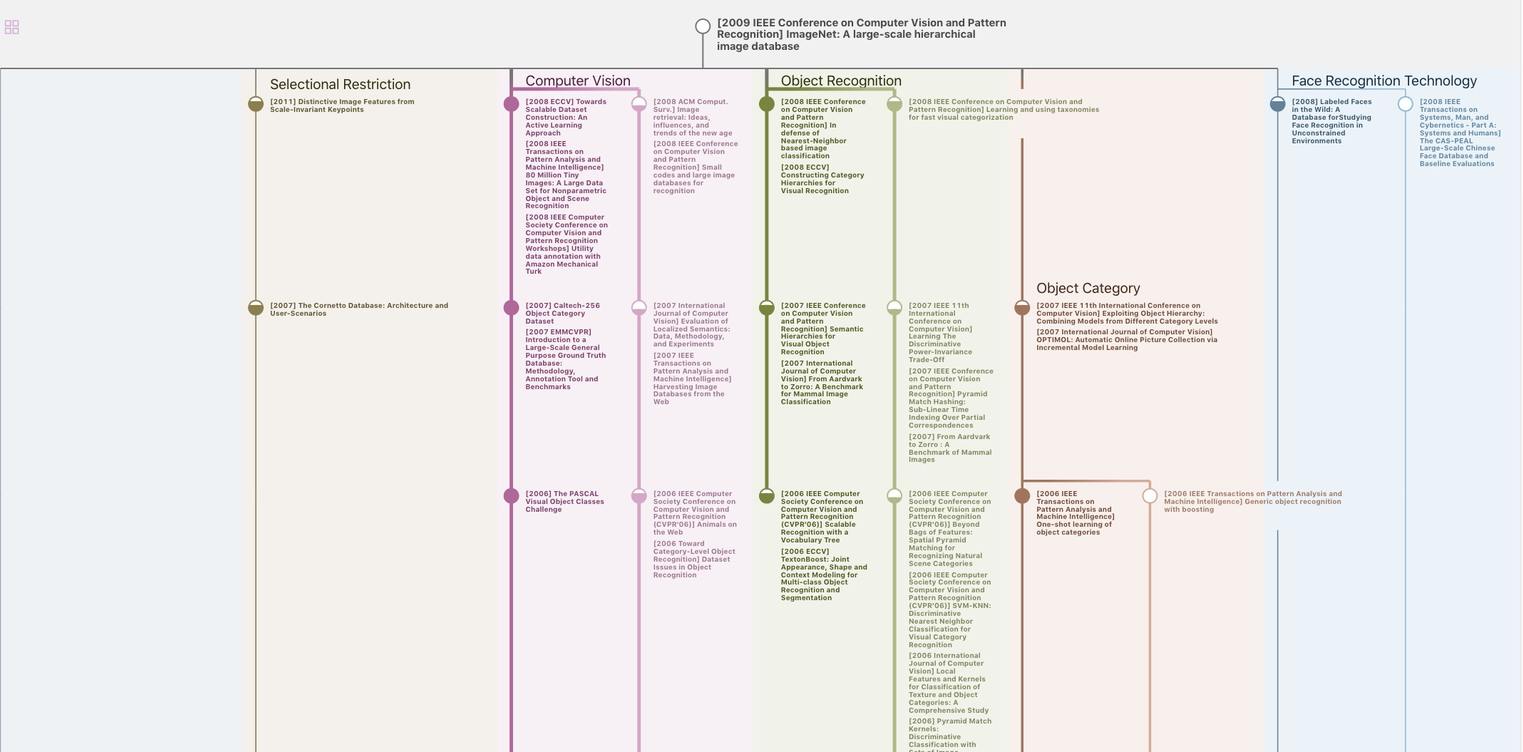
生成溯源树,研究论文发展脉络
Chat Paper
正在生成论文摘要