Stochastic optimization of industrial grinding operation through data-driven robust optimization
Elsevier eBooks(2023)
摘要
While modeling the industrial grinding circuits (IGCs), the presence of uncertain process parameters intensifies the level of difficulty, especially when the objectives aimed to be optimized are conflicting. In this chapter, considering various sources of uncertainties, a three objective IGC stochastic optimization problem is solved by means of robust optimization (RO) approach. Conventionally, researchers adopted specific geometric shape based uncertainty sets like box, diamond, and so on, for generating samples from the uncertain variable space. Nonetheless, in real scenarios, since the uncertain parameter data may be sparse and might not necessarily follow any distribution, the existing uncertainty sets may lead to conservative solutions. Thus, a new data based sampling technique for RO is presented, which utilizes unsupervised machine learning and novel generative modeling framework for identifying the intended space more accurately and sampling in the desired regions of uncertainty. An industrial grinding circuit, consisting of three decision variables, 10 parameters that depict the uncertainties in the model and feed stream and three conflicting objectives based on productivity, quality and energy savings in the process, is used to demonstrate the effectiveness of the novel framework followed by comparison studies.
更多查看译文
关键词
stochastic optimization,data-driven
AI 理解论文
溯源树
样例
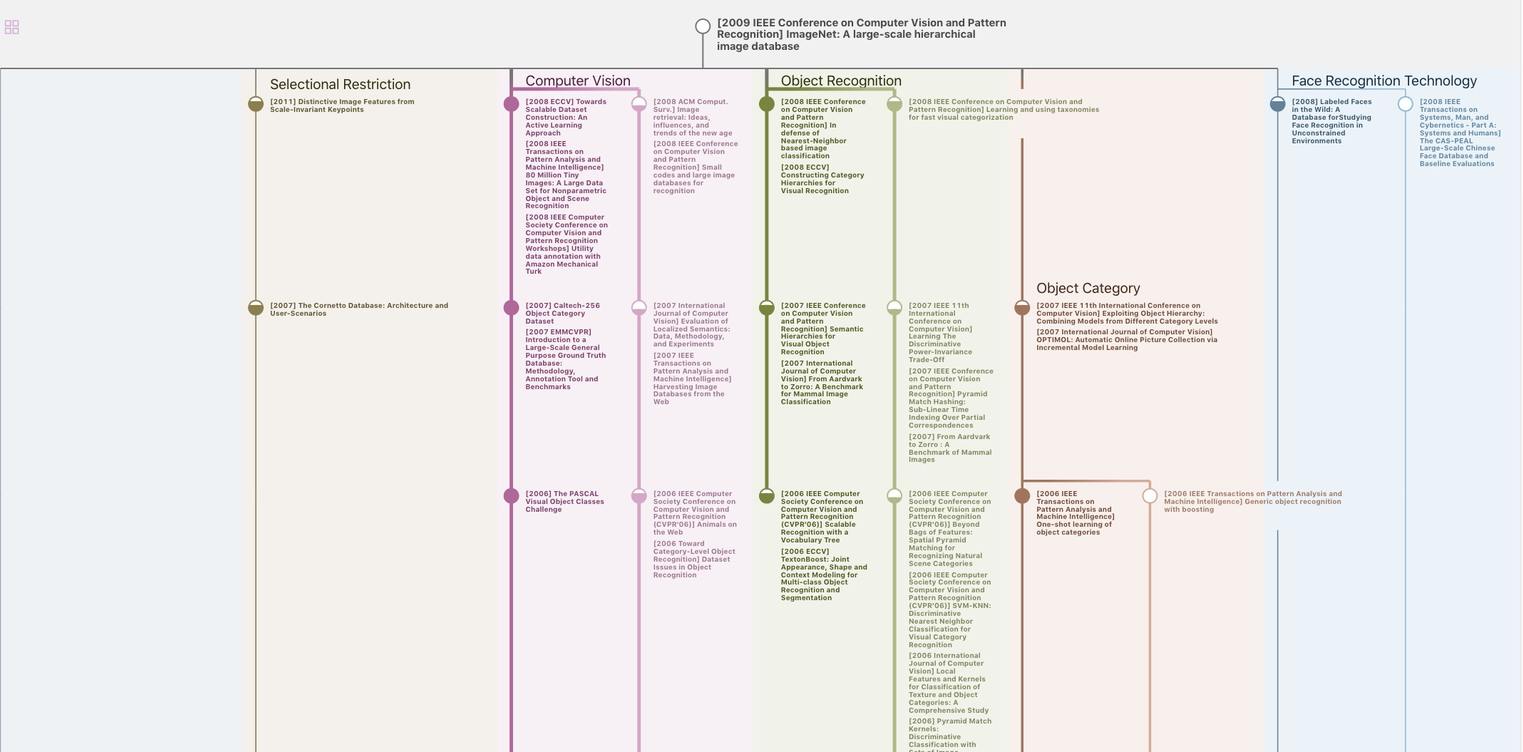
生成溯源树,研究论文发展脉络
Chat Paper
正在生成论文摘要