DetFed: Dynamic Resource Scheduling for Deterministic Federated Learning Over Time-Sensitive Networks.
IEEE Trans. Mob. Comput.(2024)
Abstract
In this paper, we present a three-layer (i.e., device, field, and factory layers) deterministic federated learning (FL) framework, named DetFed, which accelerates collaborative learning process for ultra-reliable and low-latency industrial Internet of Things (IoT) via integrating 6G-oriented Time-sensitive Networks (TSN). Utilizing dispersive local data, industrial IoT devices distributively train a deep neural network (DNN) model, and the updated model parameters are aggregated at their associated field servers every round or at a centralized factory server every a few rounds. Aiming at optimizing the learning accuracy of FL without affecting the co-transmission of burst traffic (e.g., safety-critical traffic), an integrated TSN is considered to establish connections among the three layers, where a cyclic queuing and forwarding mechanism is deployed in each switch to support deterministic model parameter transmission with microsecond-level delay and near-zero packet loss requirements. To improve the FL performance, we formulate a multi-objective stochastic optimization problem to simultaneously maximize the scheduling success ratio and learning accuracy while satisfying the deterministic requirements of delay, jitter, and packet loss. Since the objective function is implicit and the available time slots of the considered TSN in each FL round are temporally correlated, the problem is difficult to solve in real time. Therefore, we transform the problem into a Markov decision process formulation and propose a dynamic resource scheduling algorithm, based on deep reinforcement learning, to make optimal resource scheduling decisions while adapting to device heterogeneity and network dynamics. Experimental results based on real-world dataset demonstrate that the proposed DetFed significantly accelerates FL convergence and improves learning accuracy as compared to state-of-the-art benchmarks.
MoreTranslated text
Key words
Deterministic federated learning,industrial Internet of Things,co-transmission,resource scheduling,deep reinforcement learning
AI Read Science
Must-Reading Tree
Example
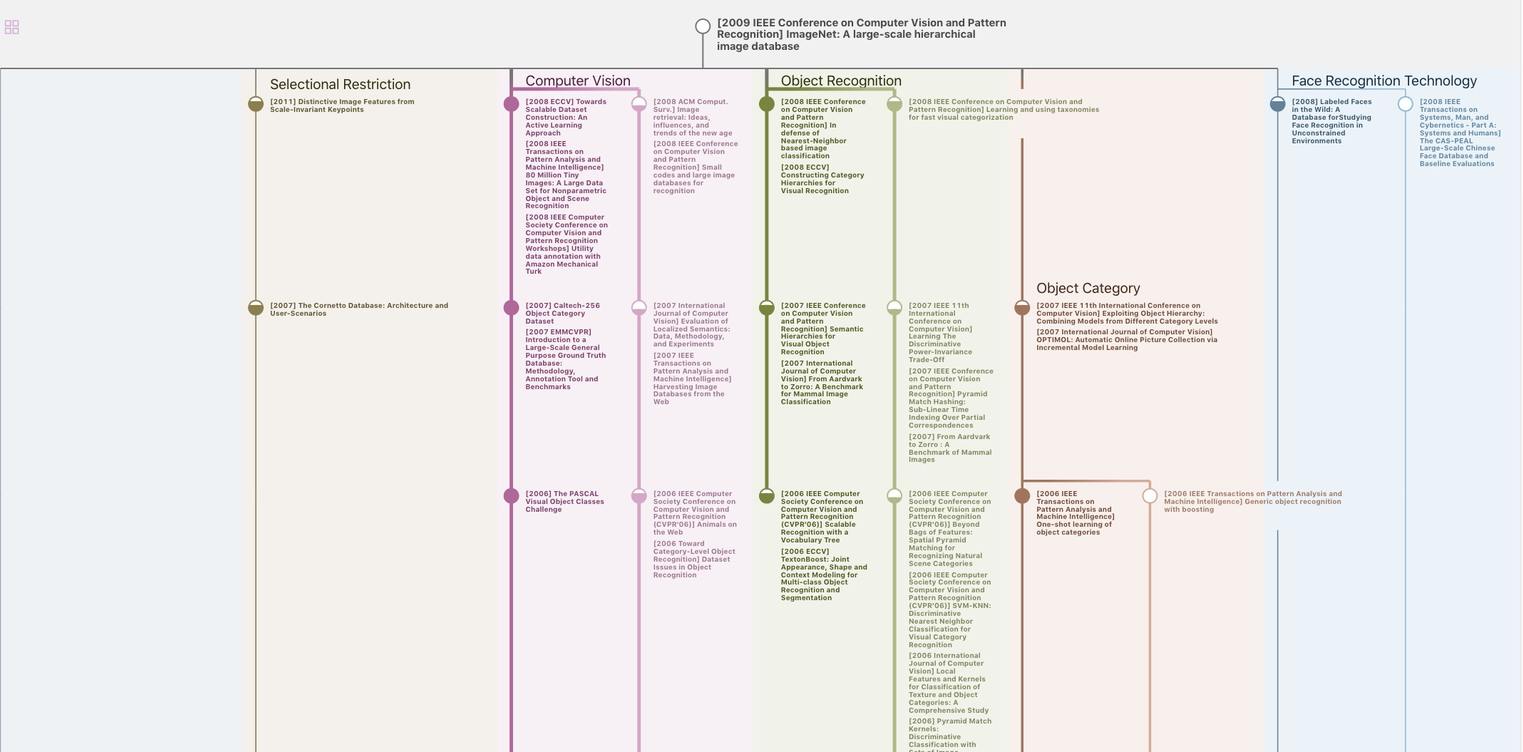
Generate MRT to find the research sequence of this paper
Chat Paper
Summary is being generated by the instructions you defined