Regularized fingerprinting in detection and attribution of climate change with weight matrix optimizing the efficiency in scaling factor estimation
The Annals of Applied Statistics(2023)
摘要
Detection and attribution analyses play a central role in establishing the causal effect of human activities on global warming. The most commonly used method in such analyses, optimal fingerprinting, is a multiple regression where each covariate has a measurement error whose covariance matrix is the same as that of the regression error up to a known scale. Inferences about the regression coefficients are critical not only for making statements about detection and attribution but also for quantifying the uncertainty in important outcomes derived from detection and attribution analyses. When there are no errors-in-variables (EIV), the optimal weight matrix in estimating the regression coefficients is the precision matrix of the regression error. This matrix, however, is never known and has to be estimated from climate model simulations with regularization. The consequence is that the optimal fingerprinting method is not optimal, as believed in practice. We construct a weight matrix by inverting a nonlinear shrinkage estimate of the error covariance matrix that minimizes loss functions directly targeting the uncertainty of the resulting regression coefficient estimator. The resulting estimator of the regression coefficients is asymptotically optimal as the sample size of the climate model simulations and the matrix dimension go to infinity together with a limiting ratio. When EIVs are present, the estimator of the regression coefficients, based on the proposed weight matrix, is asymptotically more efficient than that based on the inverse of the existing linear shrinkage estimator of the error covariance matrix. The performance of the method is confirmed in finite sample simulation studies mimicking realistic situations in terms of the length of the confidence intervals and empirical coverage rates for the regression coefficients. In an application to detection and attribution analyses of the mean temperature at different spatial scales, the method yielded shorter confidence intervals which are important for such analyses in practice.
更多查看译文
关键词
climate change,scaling,fingerprinting,weight matrix
AI 理解论文
溯源树
样例
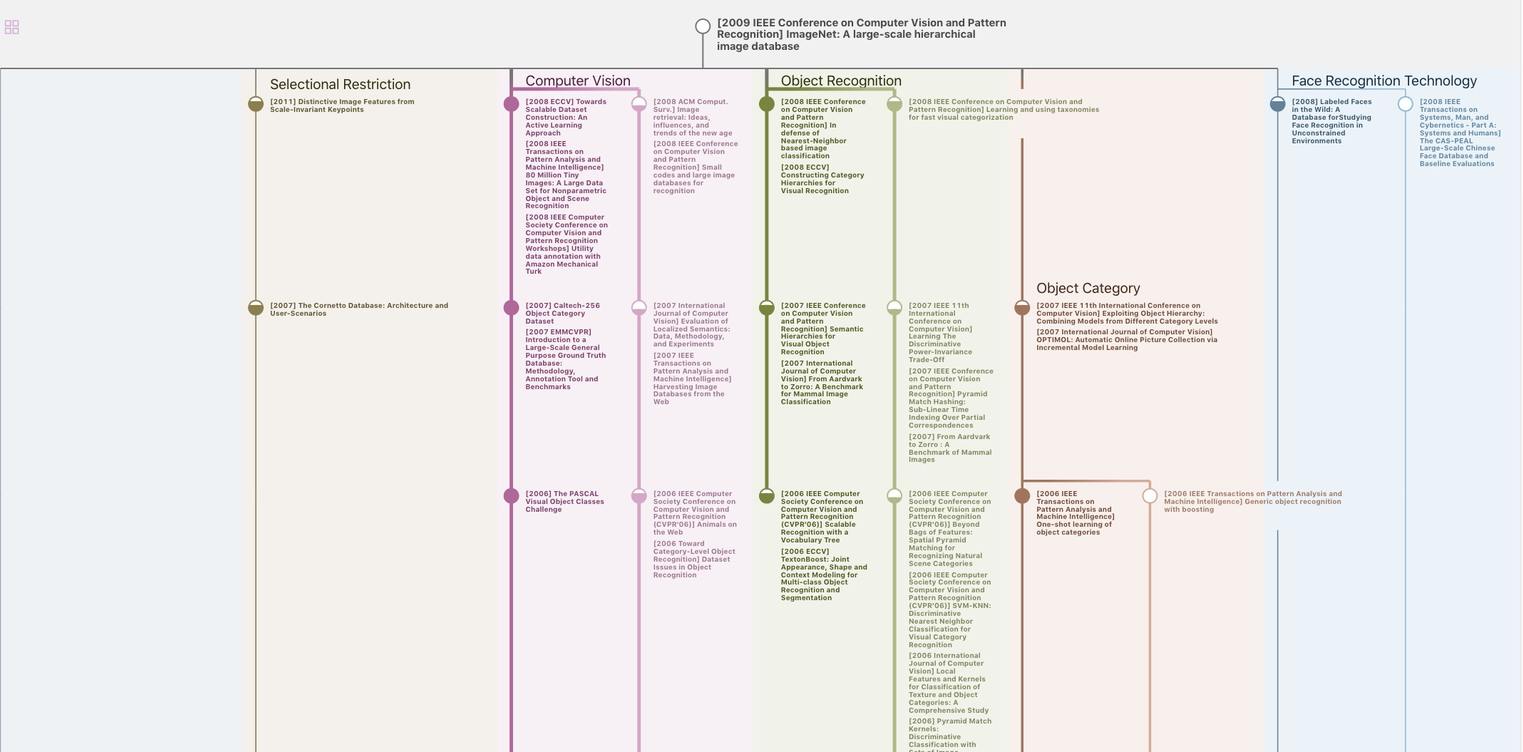
生成溯源树,研究论文发展脉络
Chat Paper
正在生成论文摘要