tUbe net: a generalisable deep learning tool for 3D vessel segmentation
bioRxiv (Cold Spring Harbor Laboratory)(2023)
摘要
Deep learning has become an invaluable tool for bioimage analysis but, while open-source cell annotation software such as cellpose are widely used, an equivalent tool for three-dimensional (3D) vascular annotation does not exist. With the vascular system being directly impacted by a broad range of diseases, there is significant medical interest in quantitative analysis for vascular imaging. However, existing deep learning approaches for this task are specialised to particular tissue types or imaging modalities. We present a new deep learning model for segmentation of vasculature that is generalisable across tissues, modalities, scales and pathologies. To create a generalisable model, a 3D convolutional neural network was trained using data from multiple modalities including optical imaging, computational tomography and photoacoustic imaging. Through this varied training set, the model was forced to learn common features of vessels cross-modality and scale. Following this, the general model was fine-tuned to different applications with a minimal amount of manually labelled ground truth data. It was found that the general model could be specialised to segment new datasets, with a high degree of accuracy, using as little as 0.3% of the volume of that dataset for fine-tuning. As such, this model enables users to produce accurate segmentations of 3D vascular networks without the need to label large amounts of training data.
更多查看译文
关键词
3d vessel segmentation,generalisable deep learning tool,tube net,deep learning
AI 理解论文
溯源树
样例
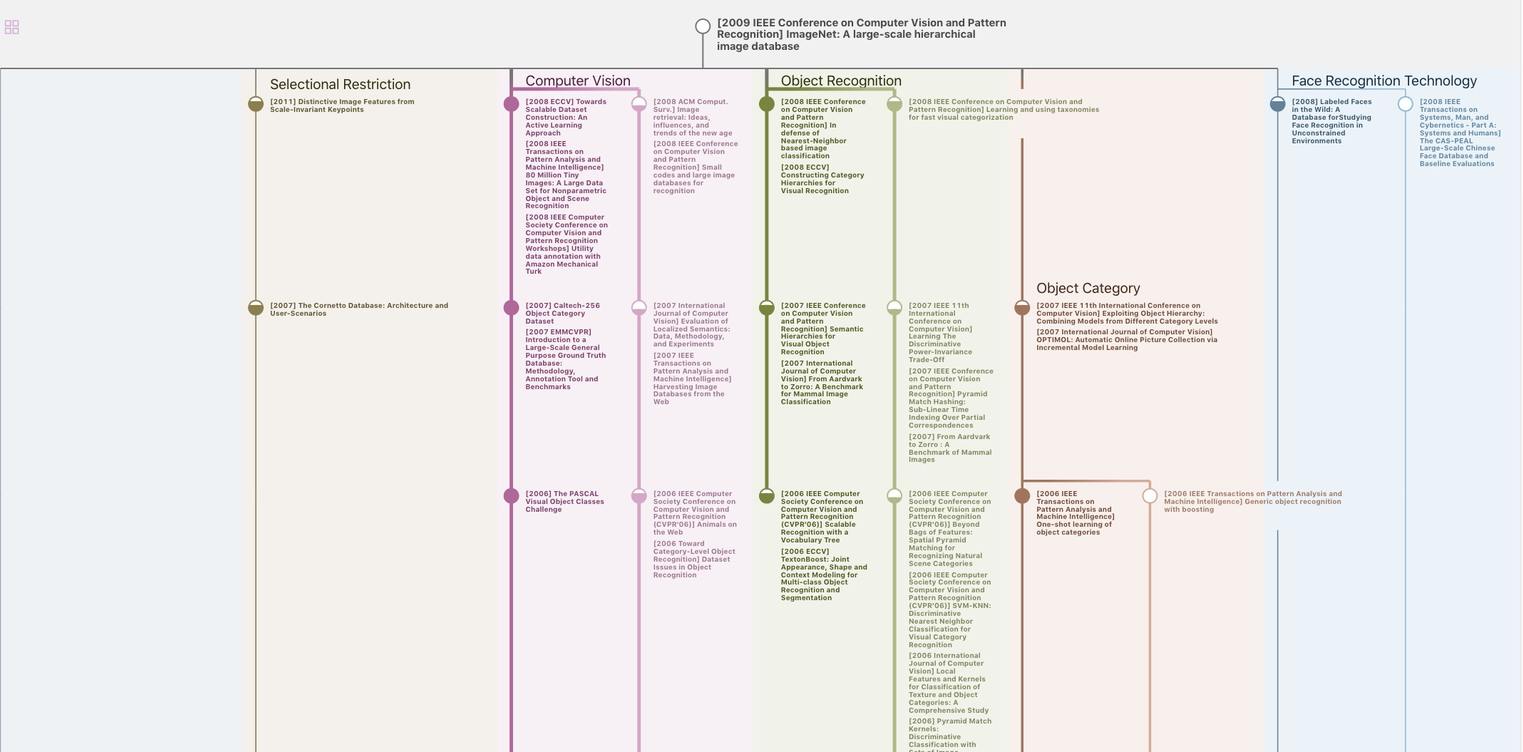
生成溯源树,研究论文发展脉络
Chat Paper
正在生成论文摘要