Online Trajectory Optimization for Energy-Efficient Cellular-Connected UAVs With Map Reconstruction.
IEEE Trans. Veh. Technol.(2024)
摘要
In this paper, we leverage the outage probability knowledge map to characterize the connection between unmanned aerial vehicles (UAVs) and cellular networks. The outage probability knowledge map is a database that simulates the connection between UAV and the cellular network during real hovers, which helps to enhance the UAV's awareness of the environment and reduce the connection interruption under complex real-time channel state information. We assume that the UAV roughly samples from the actual radio environment of the airspace in advance, and calculates the outage probability of the sampled points. After that, the UAV reconstructs the actual outage knowledge map, and flies in the airspace to learn the optimal UAV trajectory planning policy based on the reconstructed map. The optimization objective is to minimize the flight energy cost of the UAV performing tasks. In this paper, we propose a deep image prior based radio map reconstruction (DIPRMR) method to reconstruct the map, and then propose a deep reinforcement learning based trajectory optimization algorithm. The UAV that performs the task adjusts the flight trajectory based on the outage probability knowledge obtained from the reconstructed complete map. Simulation results show that the proposed online trajectory optimization scheme based on outage probability knowledge map can obtain great returns in terms of maintaining connectivity, reducing task completion time and energy consumption.
更多查看译文
关键词
Deep reinforcement learning,radio map,energy-efficient UAV,trajectory design,image reconstruction,cellular-connected UAV
AI 理解论文
溯源树
样例
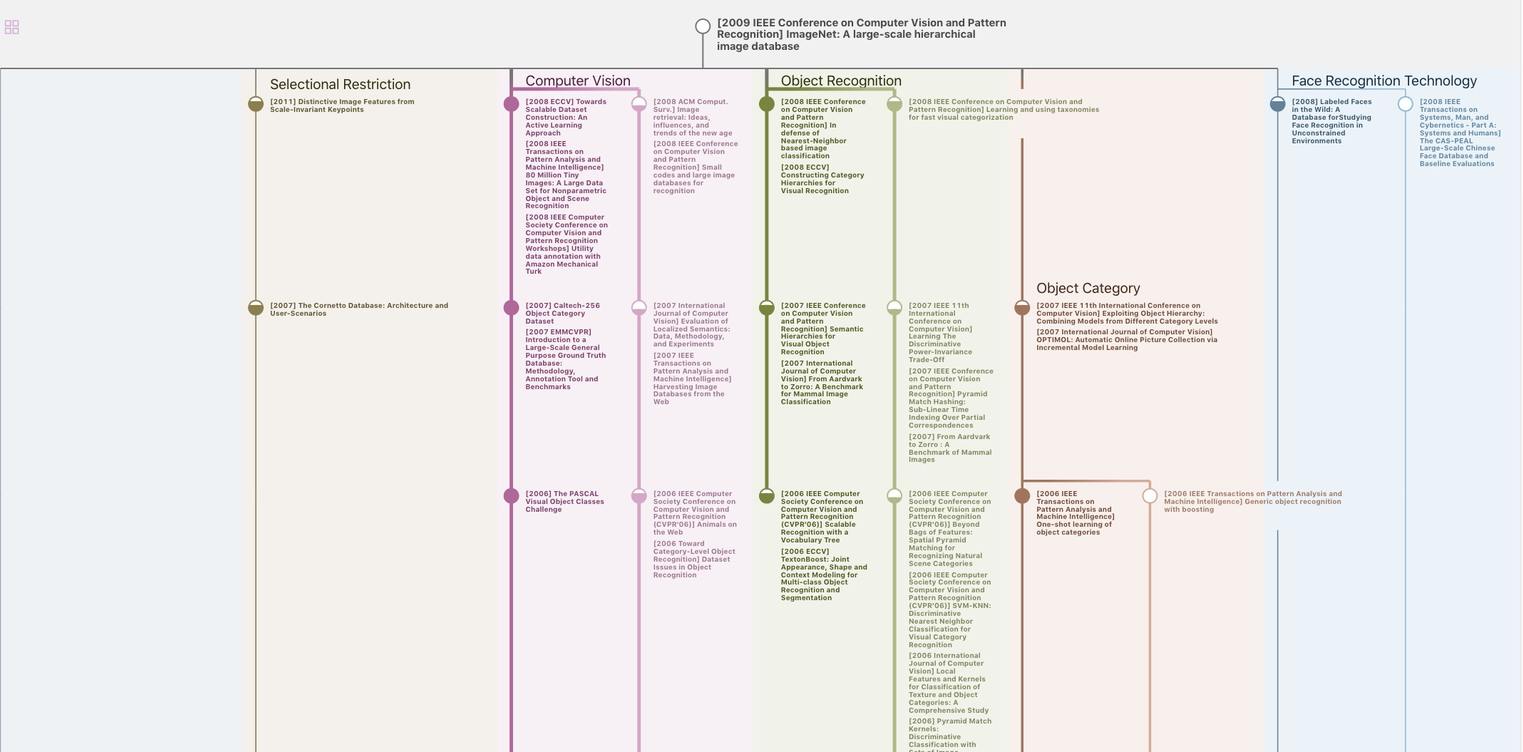
生成溯源树,研究论文发展脉络
Chat Paper
正在生成论文摘要