Measuring Community Resilience Inequality to Inland Flooding Using Location Aware Big Data
Cities(2024)
Key words
Community resilience,Inequality,Priority,Inland flooding,Location aware big data
AI Read Science
Must-Reading Tree
Example
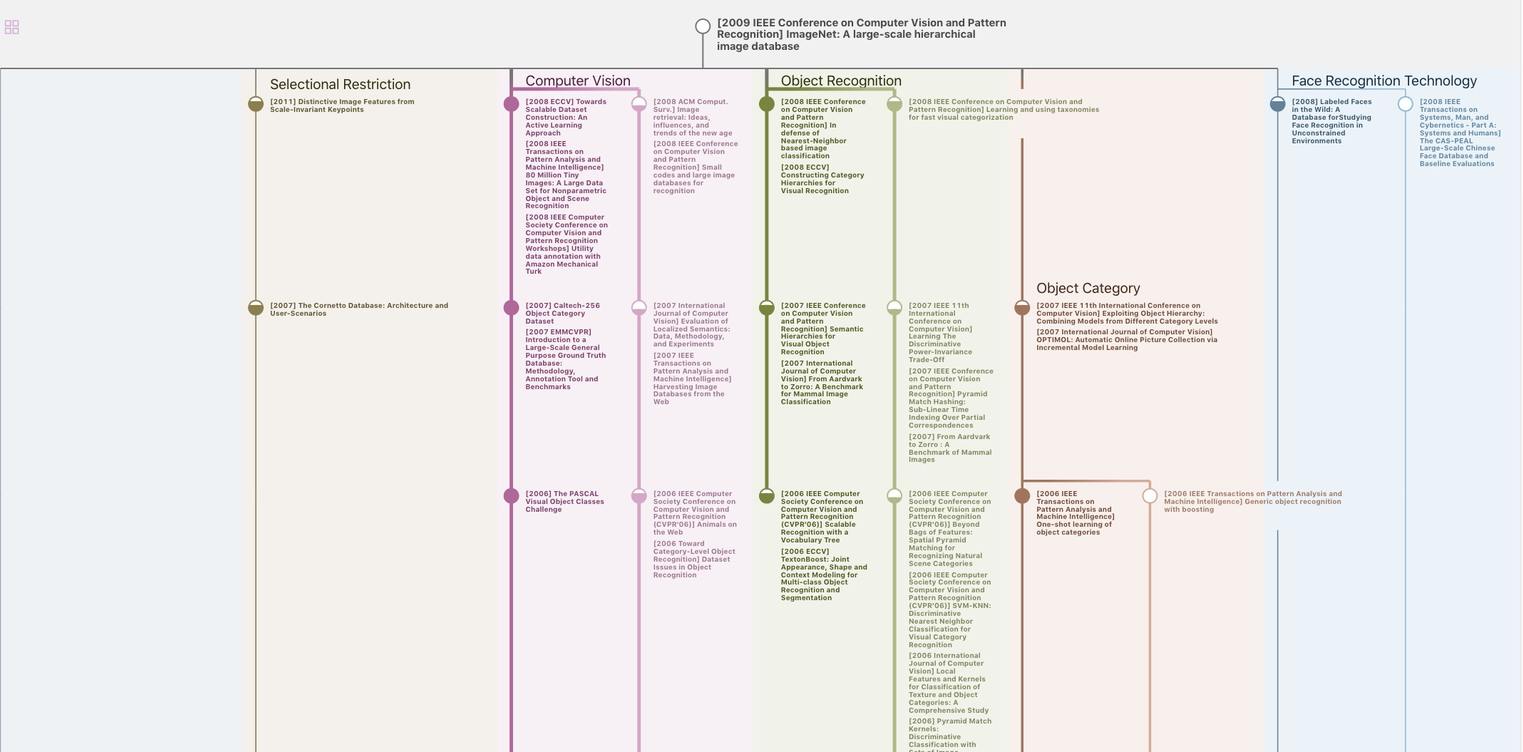
Generate MRT to find the research sequence of this paper
Chat Paper
Summary is being generated by the instructions you defined