Randomized Low-Rank Nonlinear RX Detector
Communications in computer and information science(2023)
摘要
Anomaly Detection is an important topic in various application areas, including image analysis and network intrusion detection. The Reed–Xiaoli (RX) detector is an efficient and accurate anomaly detector that can be used if analyzed data is Gaussian distributed. However, in the real-world, data is rarely Gaussian distributed. For nonlinear data, kernel RX (KRX) method is proposed and widely employed. The biggest issue with the KRX method is its high computational complexity, which prevents using it for big data or in real-time scenarios. As a remedy, in the literature, Random Fourier Features approximation of the KRX method is proposed, namely RRX. Another weakness of the KRX is numerical issues, basically kernel matrix being bad-conditioned, which can be solved by regularization. This situation also applies to the RRX method. In this study, we extend the RRX method with randomized Singular Value Decomposition (SVD) as an efficient solution owing to SVD being the best low-rank approximation. Our proposed method, randomized low-rank RX (RLR-RX), provides better computational complexity and better detection performance than both RRX and KRX methods. Experiments on the Airport-Beach-urban (ABU) Dataset shows that proposed RLR-RX method provides better detection performance as its Area Under the Curve (AUC) is 0.9707 where AUC is 0.9660 for RRX and 0.8842 for KRX while RLR-RX is 30% faster than RRX and 44 times faster than KRX.
更多查看译文
关键词
low-rank
AI 理解论文
溯源树
样例
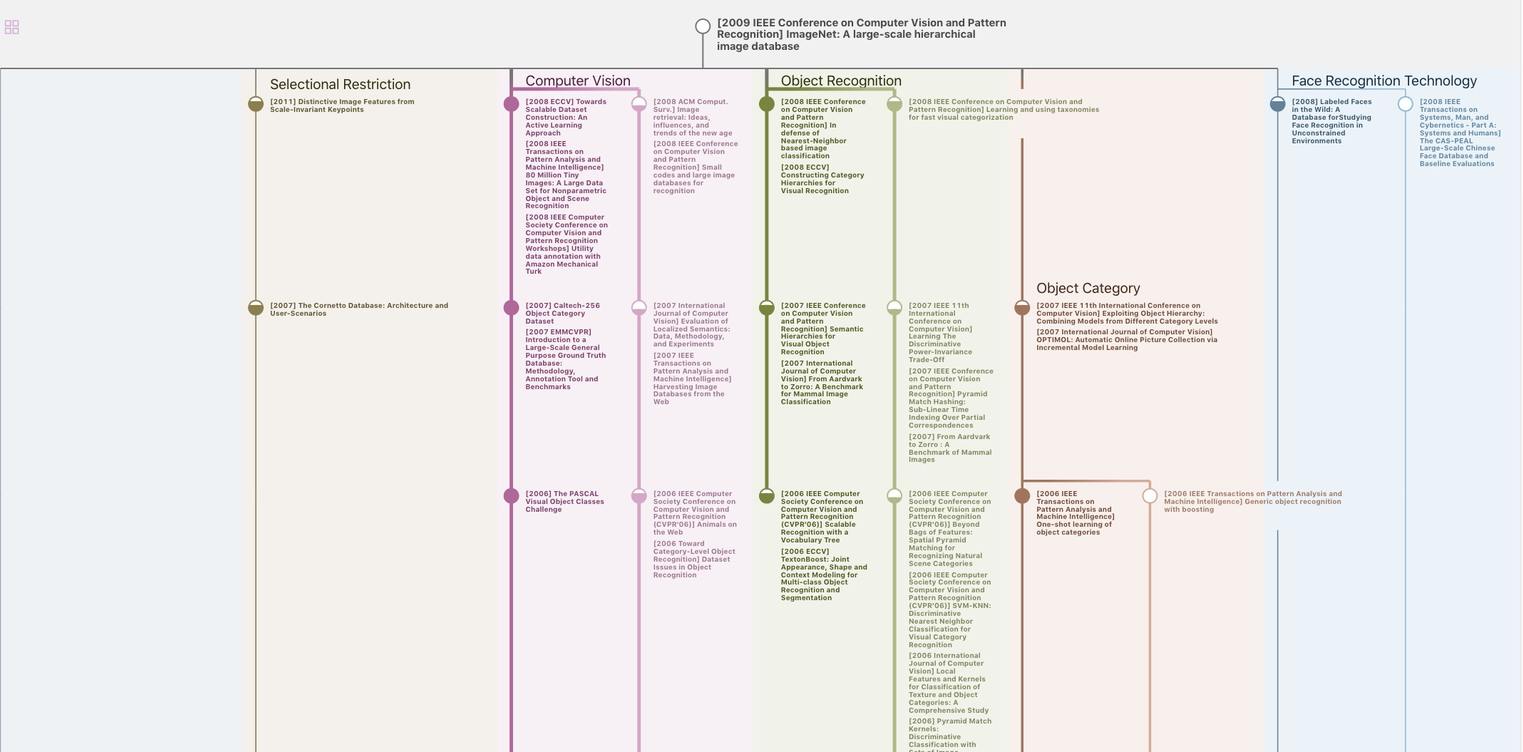
生成溯源树,研究论文发展脉络
Chat Paper
正在生成论文摘要