i-Sample: Augment Domain Adversarial Adaptation Models for WiFi-based HAR.
ACM Trans. Sens. Networks(2024)
摘要
Recently, using deep learning to achieve WiFi-based human activity recognition (HAR) has drawn significant attention. While capable of achieving accurate identification in a single domain (i.e., training and testing in the same consistent WiFi environment), it would become extremely tough when WiFi environments change significantly. As such, domain adversarial neural networks-based approaches have been proposed to handle such diversities across domains, yet often found to share the same limitation in practice: the imbalance between high-capacity of feature extractors and data insufficiency of source domains. This article proposes i-Sample, an intermediate sample generation-based framework, striving to tackle this issue for WiFi-based HAR. i-Sample is mainly designed as two-stage training, where four data augmentation operations are proposed to train a coarse domain-invariant feature extractor in the first stage. In the second stage, we leverage the gradients of classification error to generate intermediate samples to refine the classifiers together with original samples, making i-Sample also capable to be integrated into most domain adversarial adaptation methods without neural network modification. We have implemented a prototype system to evaluate i-Sample, which shows that i-Sample can effectively augment the performance of nowadays mainstream domain adversarial adaptation models for WiFi-based HAR, especially when source domain data is insufficient.
更多查看译文
关键词
Wifi-based human activity recognition,domain adversarial adaptation,inter-level sample generation
AI 理解论文
溯源树
样例
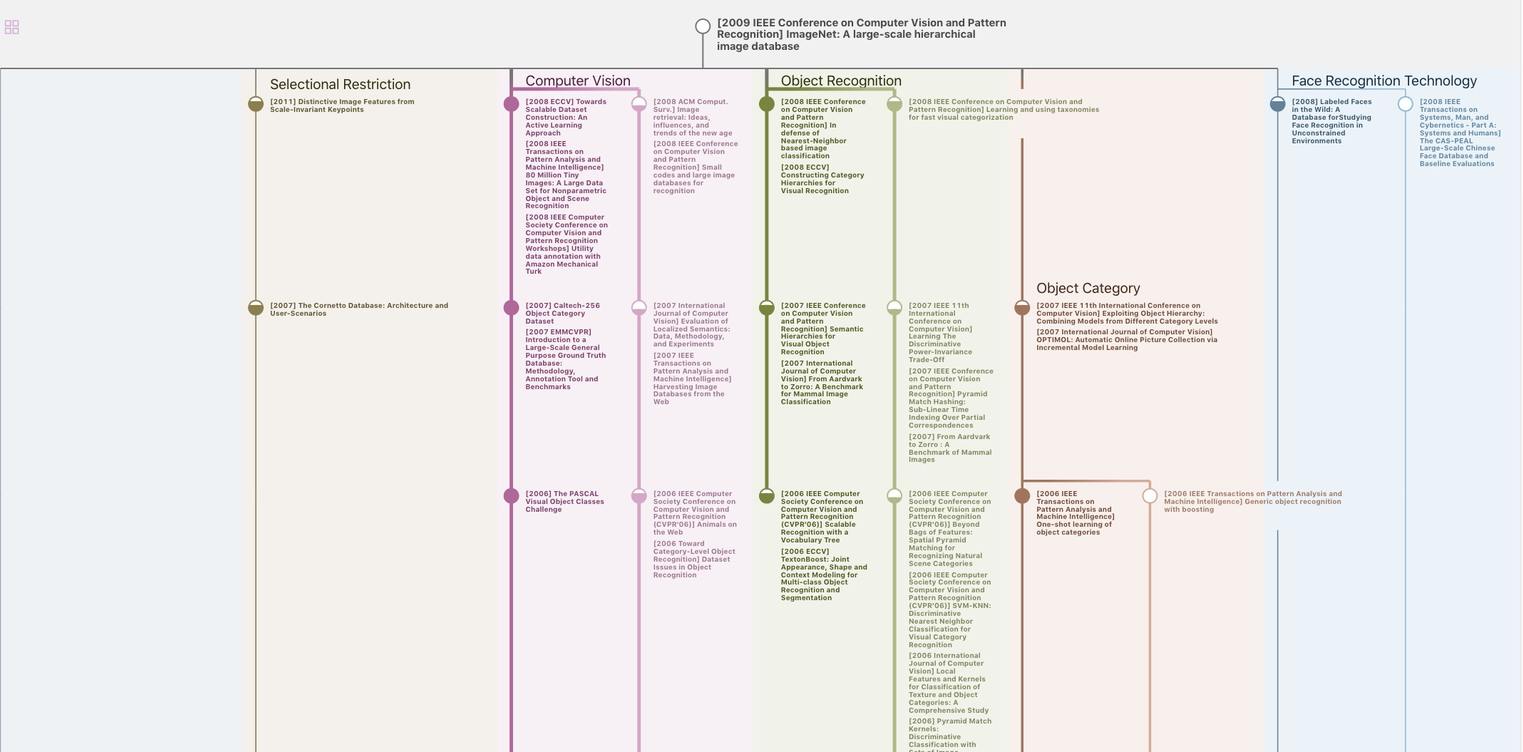
生成溯源树,研究论文发展脉络
Chat Paper
正在生成论文摘要