Motion Matters: Neural Motion Transfer for Better Camera Physiological Measurement.
IEEE/CVF Winter Conference on Applications of Computer Vision(2024)
摘要
Machine learning models for camera-based physiological measurement can have weak generalization due to a lack of representative training data. Body motion is one of the most significant sources of noise when attempting to recover the subtle cardiac pulse from a video. We explore motion transfer as a form of data augmentation to introduce motion variation while preserving physiological changes of interest. We adapt a neural video synthesis approach to augment videos for the task of remote photoplethysmography (rPPG) and study the effects of motion augmentation with respect to 1) the magnitude and 2) the type of motion. After training on motion-augmented versions of publicly available datasets, we demonstrate a 47% improvement over existing inter-dataset results using various state-of-the-art methods on the PURE dataset. We also present inter-dataset results on five benchmark datasets to show improvements of up to 79% using TS-CAN, a neural rPPG estimation method. Our findings illustrate the usefulness of motion transfer as a data augmentation technique for improving the generalization of models for camera-based physiological sensing. We release our code for using motion transfer as a data augmentation technique on three publicly available datasets, UBFC-rPPG, PURE, and SCAMPS, and models pre-trained on motion-augmented data here: https://motion-matters.github.io/
更多查看译文
关键词
Applications,Biomedical / healthcare / medicine,Applications,Remote Sensing
AI 理解论文
溯源树
样例
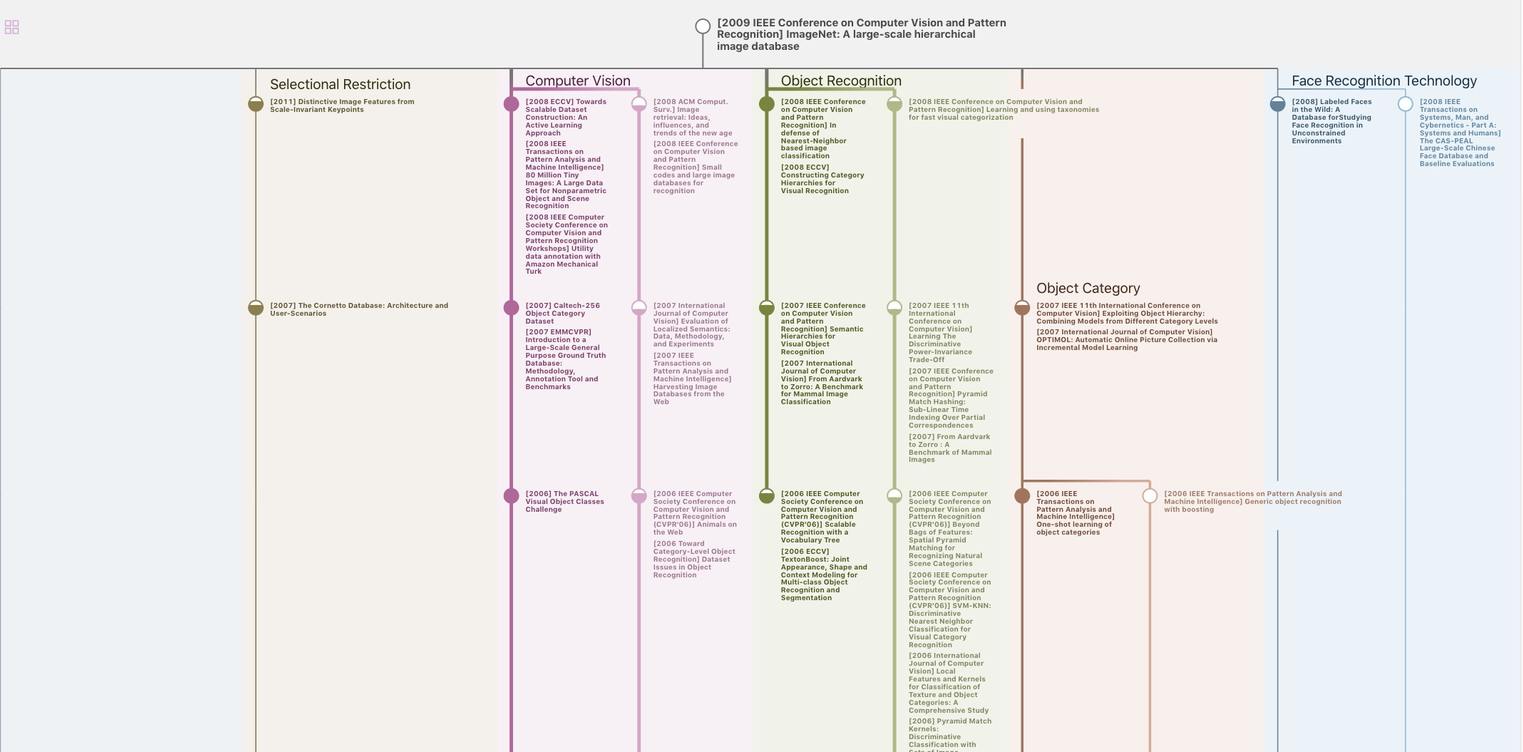
生成溯源树,研究论文发展脉络
Chat Paper
正在生成论文摘要