Temporally Language Grounding With Multi-Modal Multi-Prompt Tuning.
IEEE Trans. Multim.(2024)
摘要
The task of temporally language grounding (TLG), aiming to locate a video moment within an untrimmed video that matches a given textual query, has attracted considerable research attention in recent years. Typical retrieval-based TLG methods are inefficient due to their reliance on a large number of pre-segmented candidate moments, while localization-based TLG solutions adopt reinforcement learning, resulting in unstable convergence. Meanwhile, the cutting-edge capabilities of multi-modal architecture, especially pre-training paradigm, have not been fully exploited. Therefore, how to perform TLG task efficiently and stably is a non-trivial task. In this work, we propose a novel TLG solution named Multi-modal Multi-Prompt Tuning (MMPT), which formulates the TLG task as a prompt-based multi-modal problem and integrates multiple sub-tasks to tune the performance. In this way, off-the-shelf pre-trained models can be directly leveraged to achieve more stable performance. Specifically, a flexible multi-prompt strategy is contributed to rewrite the query firstly, which contains the query, the start and end timestamps. Among them, various prompt templates are integrated to enhance robustness. Thereafter, a multi-modal Transformer is adopted to fully learn the multi-modal context. Moreover, we design various sub-tasks to optimize this novel framework including the matching task, localization task and joint learning task. Extensive experiments on two real-world datasets validate the effectiveness and rationality of our proposed solution.
更多查看译文
关键词
Temporally language grounding,prompt learning,multi-modal understanding
AI 理解论文
溯源树
样例
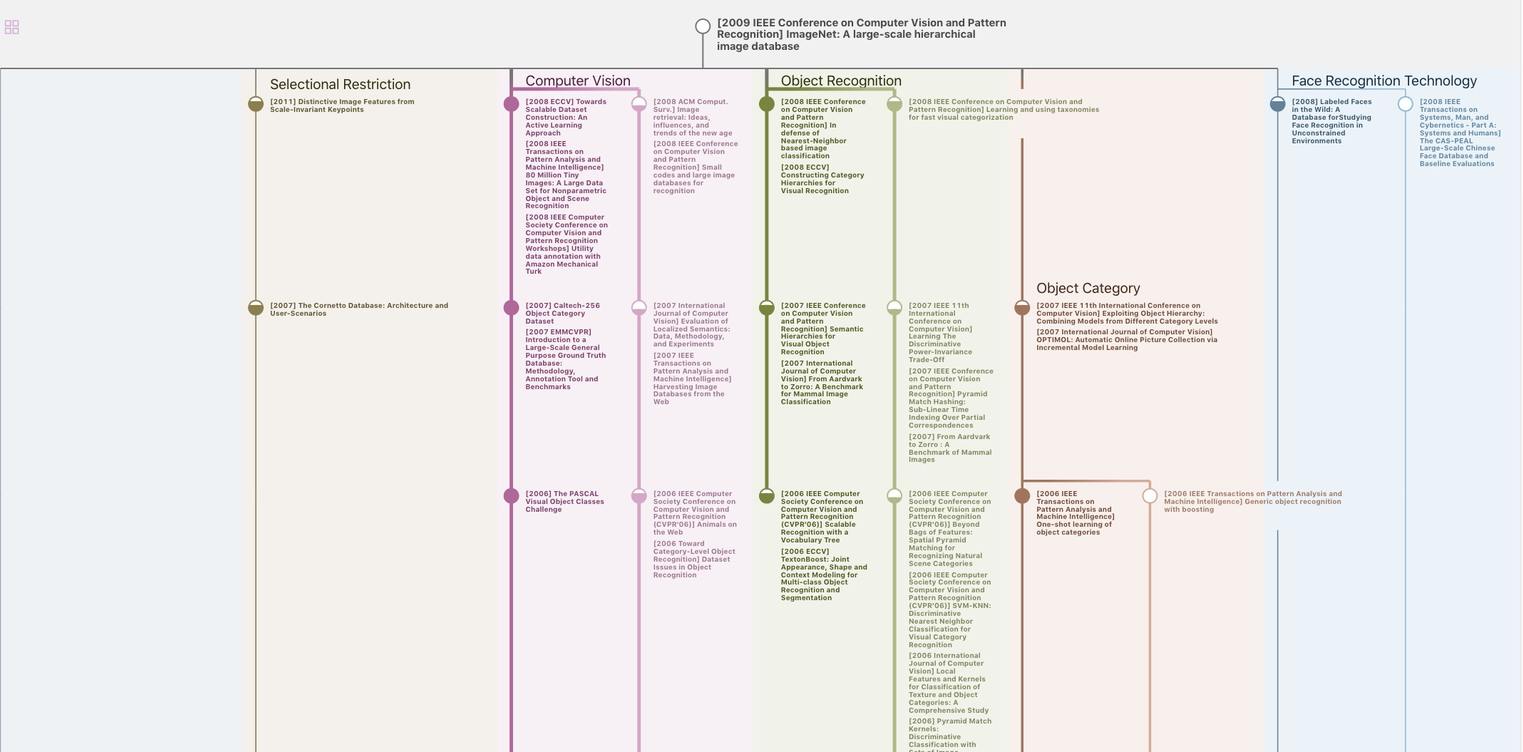
生成溯源树,研究论文发展脉络
Chat Paper
正在生成论文摘要