HeGA
Proceedings of the 31st ACM International Conference on Information & Knowledge Management(2022)
摘要
Trajectory prediction enables the fast and accurate response of autonomous driving navigation in complex and dense traffics. In this paper, we present a novel trajectory prediction network called Heterogeneous Graph Aggregation (HeGA) for high-density heterogeneous traffic, where the traffic agents of various categories interact densely with each other. To predict the trajectory of a target agent, HeGA first automatically selects neighbors that interact with it by our proposed adaptive neighbor selector, and then aggregates their interactions based on a novel two-phase aggregation transformer block. At last, the historical residual connection LSTM enhances the historical information awareness and decodes the spatial coordinates as the prediction results. Extensive experiments on real data demonstrate that the proposed network significantly outperforms the existing state-of-the-art competitors by over 27% on average displacement error (ADE) and over 31% on final displacement error (FDE). We also deploy HeGA in a state-of-the-art framework for autonomous driving, demonstrating its superior applicability based on three simulated environments with different densities and complexities.
更多查看译文
AI 理解论文
溯源树
样例
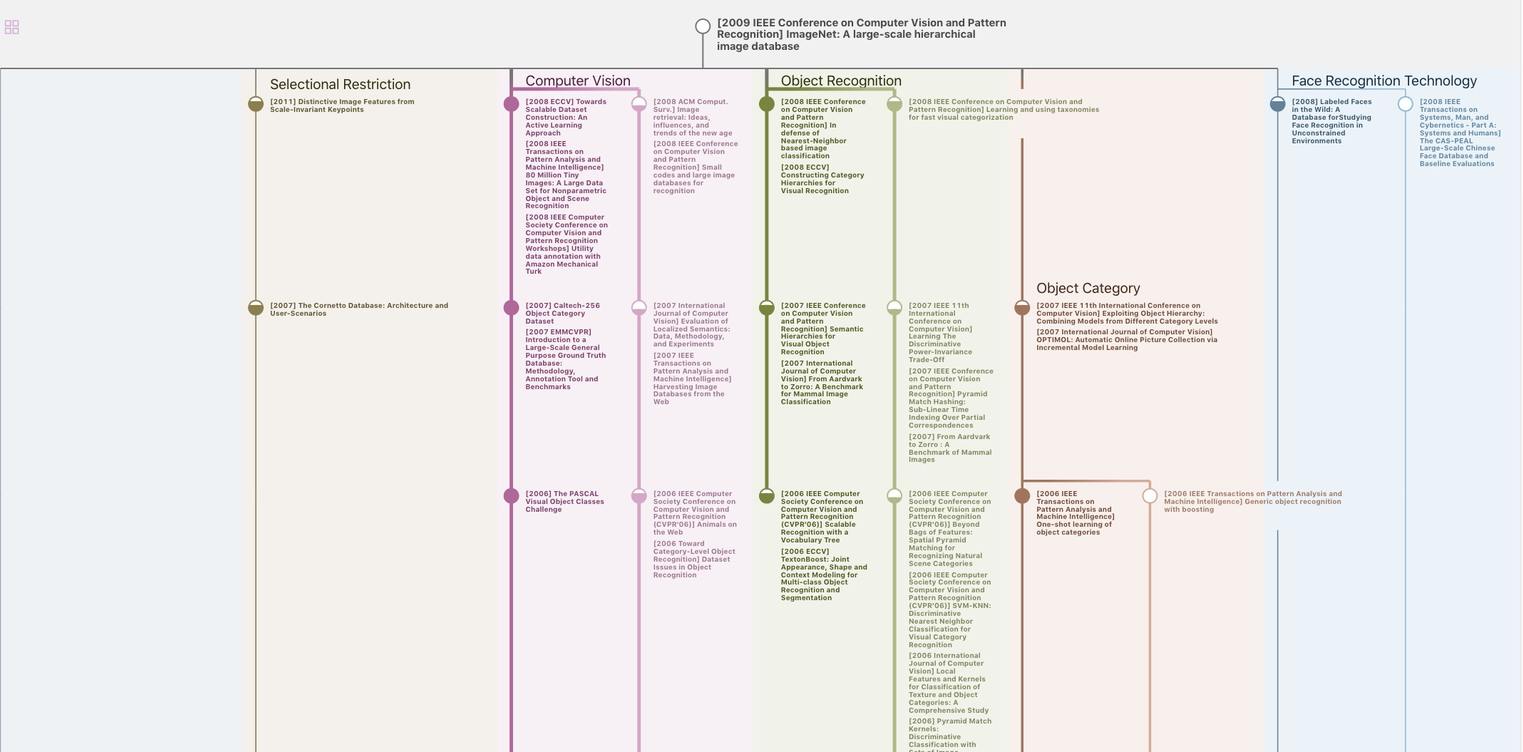
生成溯源树,研究论文发展脉络
Chat Paper
正在生成论文摘要