Aries
Proceedings of the 31st ACM International Conference on Information & Knowledge Management(2022)
摘要
With the prevalence of location-based services (LBS), trajectories are being generated rapidly. As is widely used in LBS, top-k trajectory similarity query serves as a key operation, deeply empowering applications such as travel route recommendation and carpooling. Given the rise of deep learning, trajectory representation has been well-proven to speed up this operator. However, existing representation-based computing modes remain two major problems understudied: the low quality of trajectory representation and insufficient support for various trajectory similarity metrics, which make them difficult to apply in practice. Therefore, we propose an Accurate metric-based representation learning approach for fast top-k trajectory similarity query, named Aries. Specifically, Aries has two sophisticated modules: (1) An novel trajectory embedding strategy enhanced by the bidirectional LSTM encoder and spatial attention mechanism, which can extract more precise and comprehensive knowledge. (2) A deep metric learning network aggregating multiple measures for better top-k query. Extensive experiments conducted on real trajectory dataset show that Aries achieves both impressive accuracy and lower training time compared with state-of-the-art solutions. In particular, it achieves 5x-10x speedup and 10%-20% accuracy improvement over Euclidean, Hausdorff, DTW, and EDR measures. Besides, our method can maintain stable performance when handling various scenarios, without repeated training in order to adapt to diverse similarity metrics.
更多查看译文
AI 理解论文
溯源树
样例
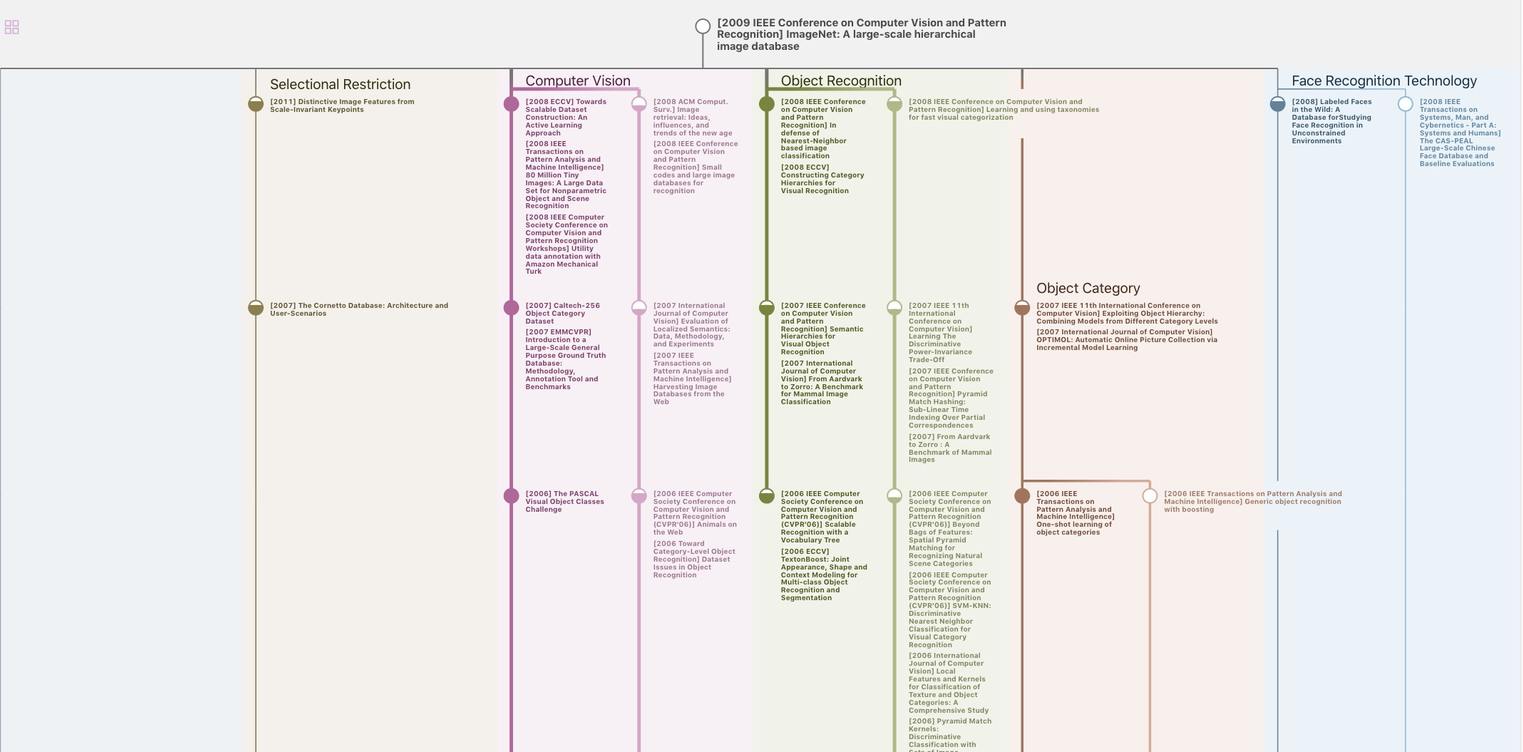
生成溯源树,研究论文发展脉络
Chat Paper
正在生成论文摘要