PartSLIP: Low-Shot Part Segmentation for 3D Point Clouds via Pretrained Image-Language Models
arXiv (Cornell University)(2022)
摘要
Generalizable 3D part segmentation is important but challenging in vision and robotics. Training deep models via conventional supervised methods requires large-scale 3D datasets with fine-grained part annotations, which are costly to collect. This paper explores an alternative way for low-shot part segmentation of 3D point clouds by leveraging a pretrained image-language model, GLIP, which achieves superior performance on open-vocabulary 2D detection. We transfer the rich knowledge from 2D to 3D through GLIP-based part detection on point cloud rendering and a novel 2D-to-3D label lifting algorithm. We also utilize multi-view 3D priors and few-shot prompt tuning to boost performance significantly. Extensive evaluation on PartNet and PartNet-Mobility datasets shows that our method enables excellent zero-shot 3D part segmentation. Our few-shot version not only outperforms existing few-shot approaches by a large margin but also achieves highly competitive results compared to the fully supervised counterpart. Furthermore, we demonstrate that our method can be directly applied to iPhone-scanned point clouds without significant domain gaps.
更多查看译文
关键词
3d point clouds,partslip,low-shot,image-language
AI 理解论文
溯源树
样例
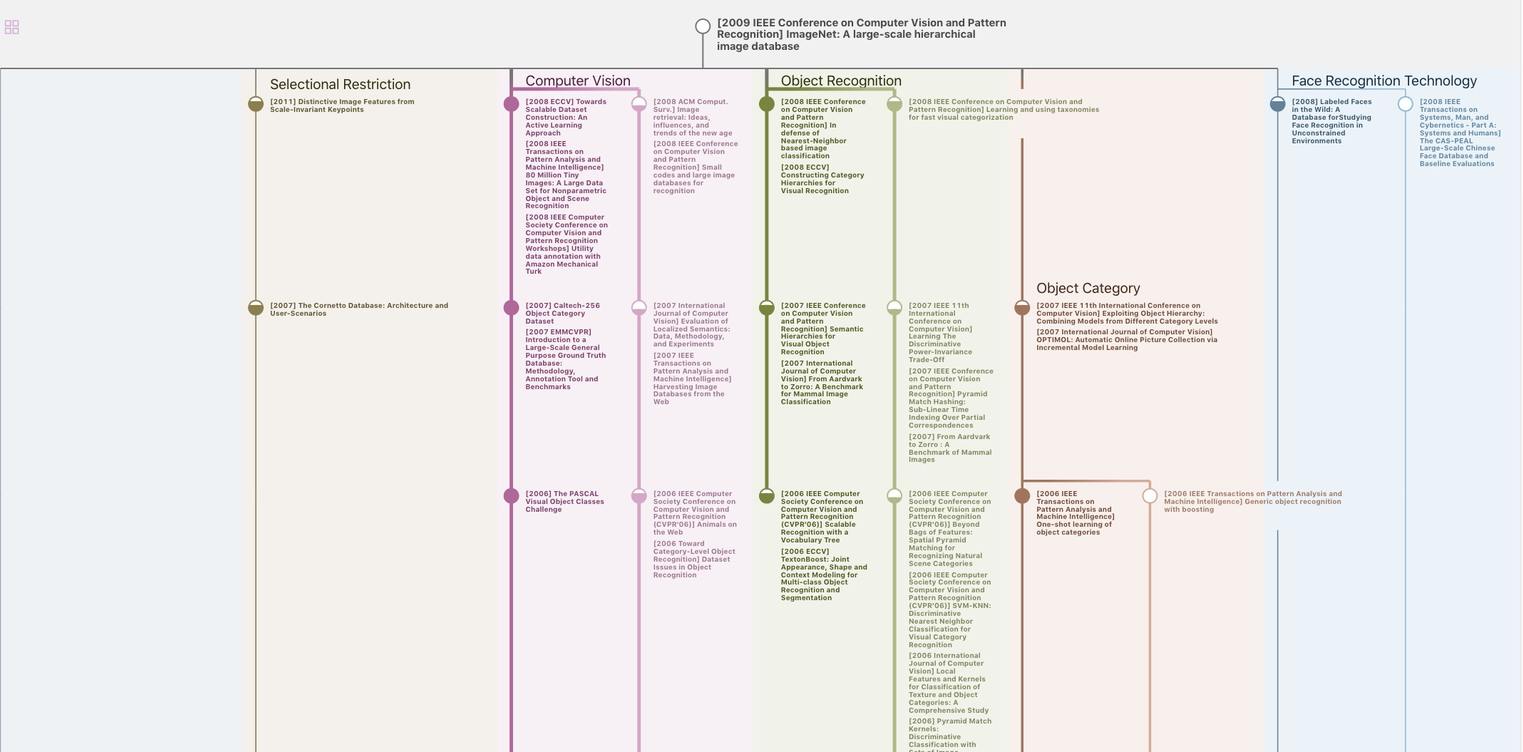
生成溯源树,研究论文发展脉络
Chat Paper
正在生成论文摘要