Visual-Language Navigation Pretraining via Prompt-based Environmental Self-exploration
arXiv (Cornell University)(2022)
摘要
Vision-language navigation (VLN) is a challenging task due to its large searching space in the environment. To address this problem, previous works have proposed some methods of fine-tuning a large model that pretrained on large-scale datasets. However, the conventional fine-tuning methods require extra human-labeled navigation data and lack self-exploration capabilities in environments, which hinders their generalization of unseen scenes. To improve the ability of fast cross-domain adaptation, we propose Prompt-based Environmental Self-exploration (ProbES), which can self-explore the environments by sampling trajectories and automatically generates structured instructions via a large-scale cross-modal pretrained model (CLIP). Our method fully utilizes the knowledge learned from CLIP to build an in-domain dataset by self-exploration without human labeling. Unlike the conventional approach of fine-tuning, we introduce prompt-based learning to achieve fast adaptation for language embeddings, which substantially improves the learning efficiency by leveraging prior knowledge. By automatically synthesizing trajectory-instruction pairs in any environment without human supervision and efficient prompt-based learning, our model can adapt to diverse vision-language navigation tasks, including VLN and REVERIE. Both qualitative and quantitative results show that our ProbES significantly improves the generalization ability of the navigation model.
更多查看译文
关键词
visual-language,prompt-based,self-exploration
AI 理解论文
溯源树
样例
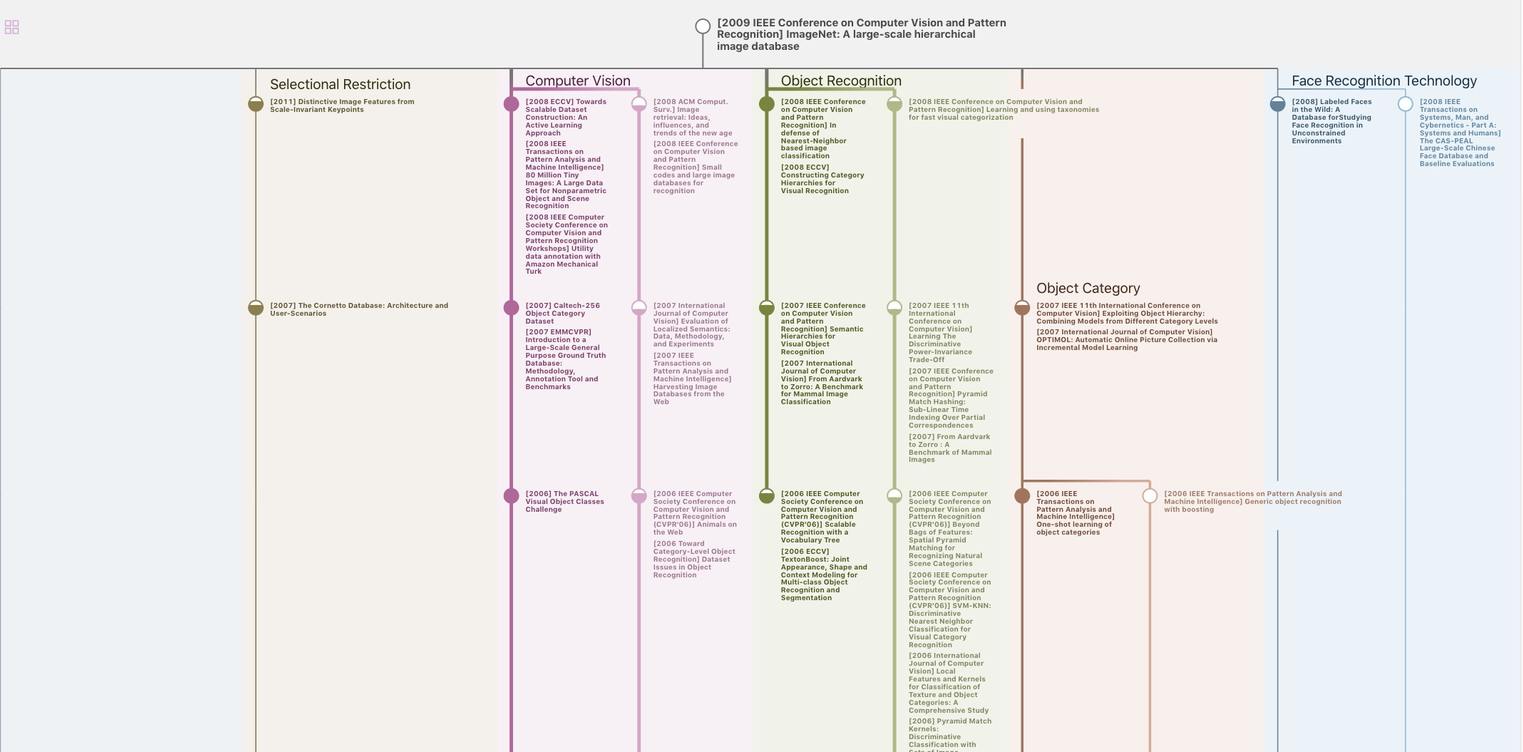
生成溯源树,研究论文发展脉络
Chat Paper
正在生成论文摘要