BenchCLAMP: A Benchmark for Evaluating Language Models on Semantic Parsing
arXiv (Cornell University)(2022)
摘要
We introduce BenchCLAMP, a Benchmark to evaluate Constrained LAnguage Model Parsing, which produces semantic outputs based on the analysis of input text through constrained decoding of a prompted or fine-tuned language model. Developers of pretrained language models currently benchmark on classification, span extraction and free-text generation tasks. Semantic parsing is neglected in language model evaluation because of the complexity of handling task-specific architectures and representations. Recent work has shown that generation from a prompted or fine-tuned language model can perform well at semantic parsing when the output is constrained to be a valid semantic representation. BenchCLAMP includes context-free grammars for six semantic parsing datasets with varied output meaning representations, as well as a constrained decoding interface to generate outputs covered by these grammars. We provide low, medium, and high resource splits for each dataset, allowing accurate comparison of various language models under different data regimes. Our benchmark supports both prompt-based learning as well as fine-tuning, and provides an easy-to-use toolkit for language model developers to evaluate on semantic parsing.
更多查看译文
关键词
evaluating language models,semantic
AI 理解论文
溯源树
样例
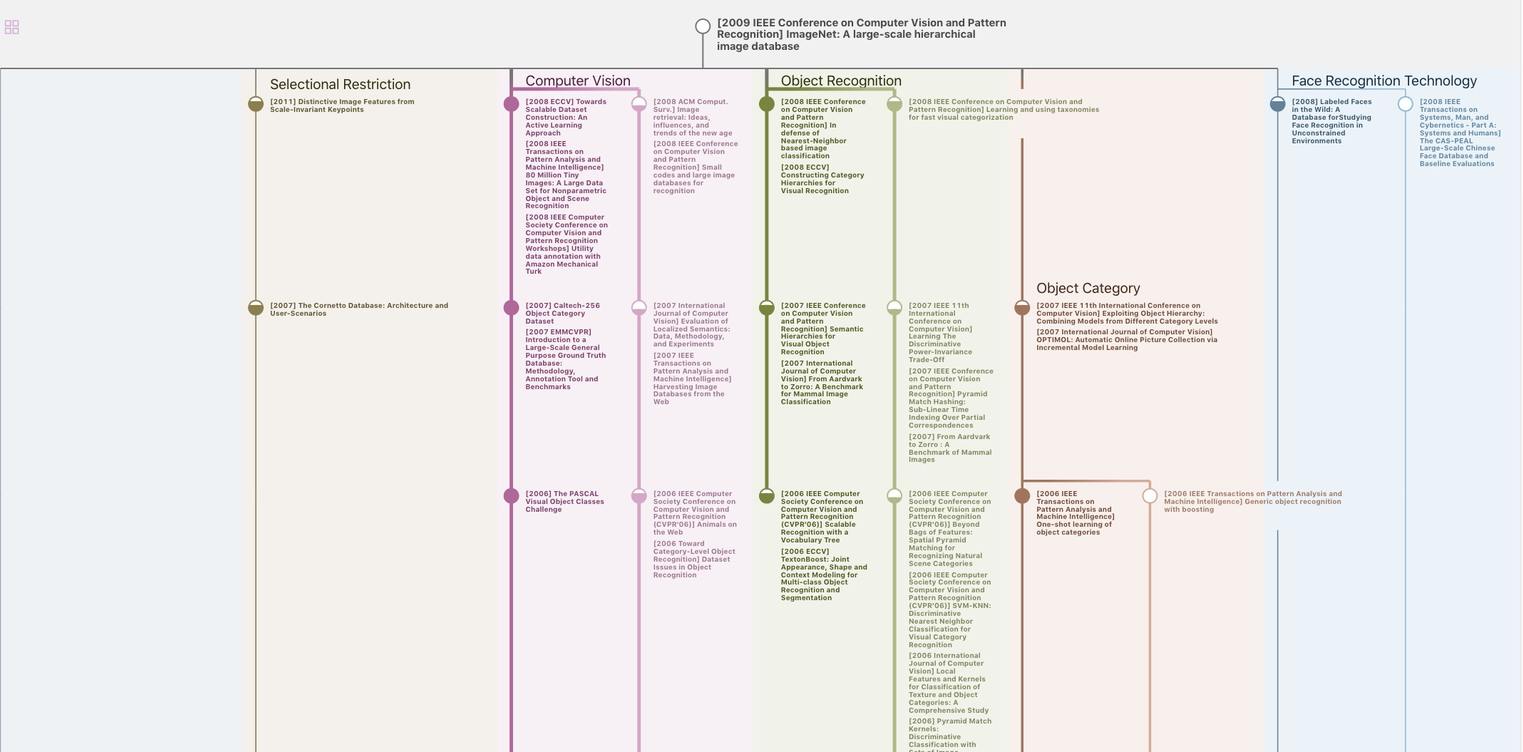
生成溯源树,研究论文发展脉络
Chat Paper
正在生成论文摘要