Feasibility of Categorizing Rehabilitation Gestures for Automated Fidelity Assessment on Strategy Training using Deep Learning.
2023 IEEE 11th International Conference on Healthcare Informatics (ICHI)(2023)
摘要
One-third to half of people with acute stroke result in newly acquired cognitive impairments. Stroke-related cognitive impairments are associated with significant functional disability. Strategy training is an intervention designed to reduce this type of disability. Randomized controlled clinical trials demonstrated the feasibility and efficacy of this intervention. To measure adherence to treatment principles of strategy training, a fidelity assessment can be performed by examining guided and directed verbal and gesture cues made by therapists in video recordings of rehabilitation sessions. One major challenge of this fidelity assessment is that the manual procedure is labor intensive, time consuming, and expensive when it is applied in a large scale. To address this challenge, in one earlier study, we leveraged natural language processing techniques to automatically identify guided and directed verbal cues from the transcripts of recorded rehabilitation sessions. The current work evaluates the feasibility for using deep learning to automatically identify guided and directed therapists’ gestures from recorded videos. The results can be used to guide the design for future study in this area.
更多查看译文
关键词
video annotation,action recognition,rehabilitation training,machine learning
AI 理解论文
溯源树
样例
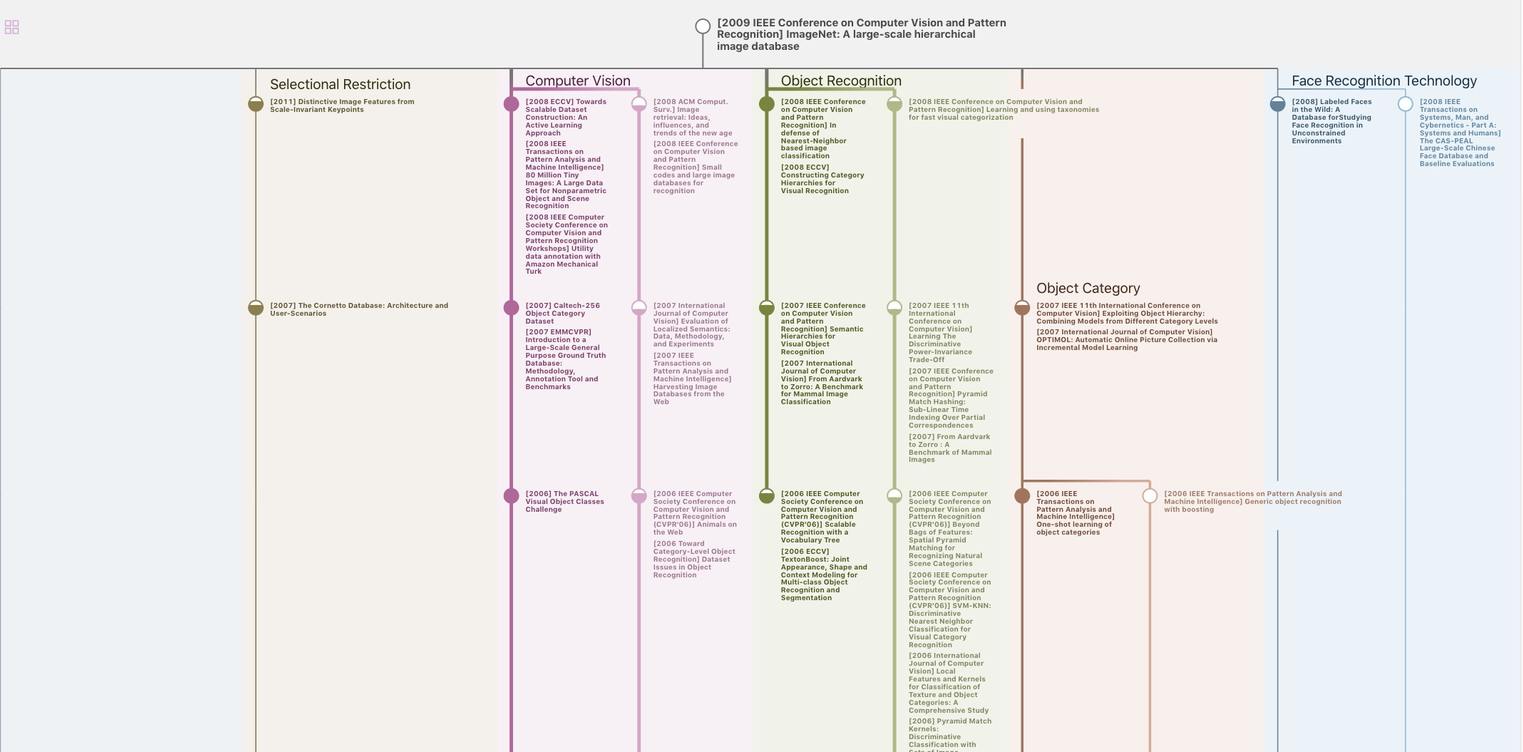
生成溯源树,研究论文发展脉络
Chat Paper
正在生成论文摘要