Uncertainty-weighted Multi-tasking for T1ρ and T2 Mapping in the Liver with Self-supervised Learning
2023 45th Annual International Conference of the IEEE Engineering in Medicine & Biology Society (EMBC)(2023)
摘要
Multi-parametric mapping of MRI relaxations in liver has the potential of revealing pathological information of the liver. A self-supervised learning based multi-parametric mapping method is proposed to map T
1ρ
and T
2
simultaneously, by utilising the relaxation constraint in the learning process. Data noise of different mapping tasks is utilised to make the model uncertainty-aware, which adaptively weight different mapping tasks during learning. The method was examined on a dataset of 51 patients with non-alcoholic fatter liver disease. Results showed that the proposed method can produce comparable parametric maps to the traditional multi-contrast pixel wise fitting method, with a reduced number of images and less computation time. The uncertainty weighting also improves the model performance. It has the potential of accelerating MRI quantitative imaging.
更多查看译文
AI 理解论文
溯源树
样例
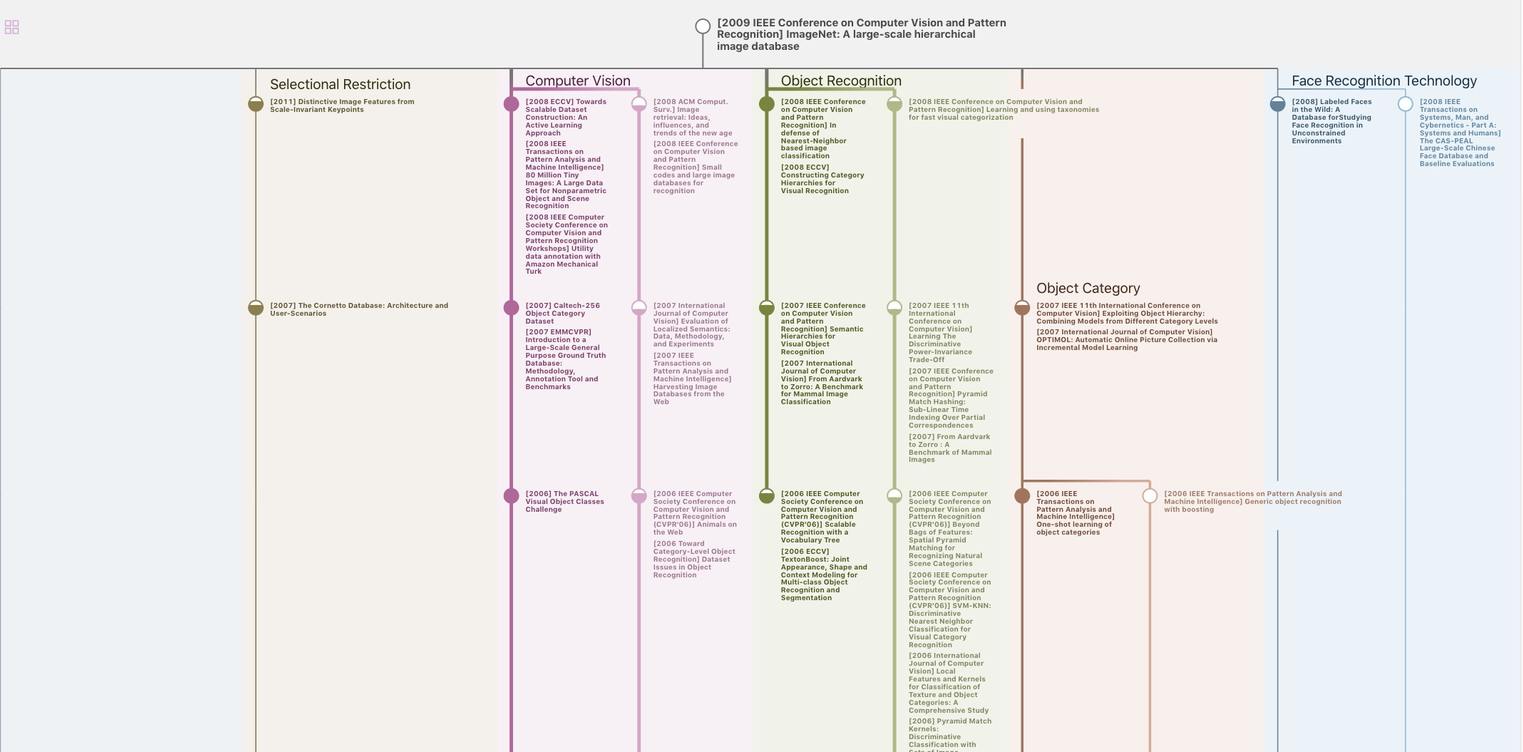
生成溯源树,研究论文发展脉络
Chat Paper
正在生成论文摘要