Cap2Aug: Caption guided Image to Image data Augmentation
arXiv (Cornell University)(2022)
Abstract
Visual recognition in a low-data regime is challenging and often prone to overfitting. To mitigate this issue, several data augmentation strategies have been proposed. However, standard transformations, e.g., rotation, cropping, and flipping provide limited semantic variations. To this end, we propose Cap2Aug, an image-to-image diffusion model-based data augmentation strategy using image captions as text prompts. We generate captions from the limited training images and using these captions edit the training images using an image-to-image stable diffusion model to generate semantically meaningful augmentations. This strategy generates augmented versions of images similar to the training images yet provides semantic diversity across the samples. We show that the variations within the class can be captured by the captions and then translated to generate diverse samples using the image-to-image diffusion model guided by the captions. However, naive learning on synthetic images is not adequate due to the domain gap between real and synthetic images. Thus, we employ a maximum mean discrepancy (MMD) loss to align the synthetic images to the real images for minimizing the domain gap. We evaluate our method on few-shot and long-tail classification tasks and obtain performance improvements over state-of-the-art, especially in the low-data regimes.
MoreTranslated text
Key words
learning,diffusion,few-shot
AI Read Science
Must-Reading Tree
Example
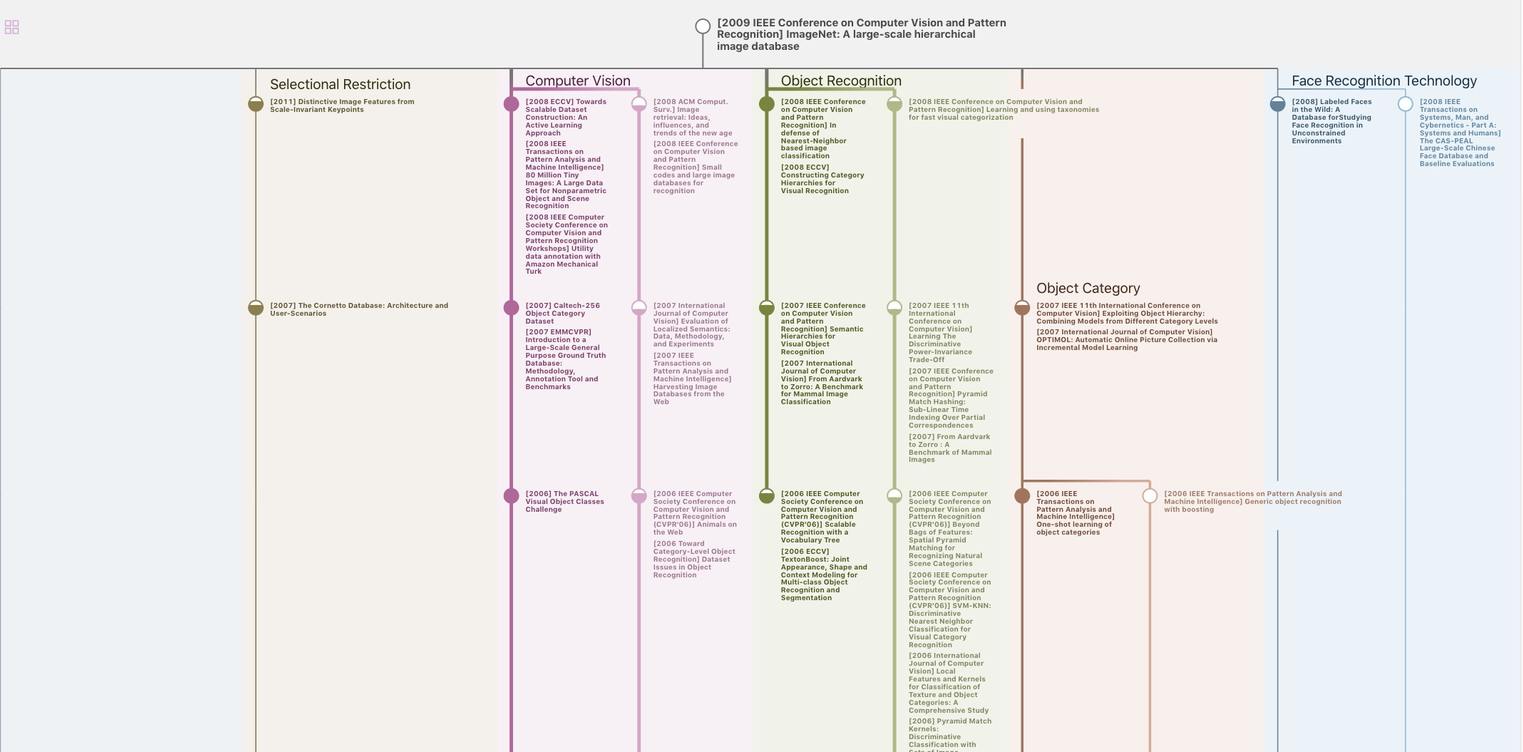
Generate MRT to find the research sequence of this paper
Chat Paper
Summary is being generated by the instructions you defined