Transfer Learning with Ensembles of Deep Neural Networks for Skin Cancer Classification in Imbalanced Data Sets.
arXiv (Cornell University)(2021)
摘要
Several machine learning techniques for accurate detection of skin cancer from medical images have been reported. Many of these techniques are based on pre-trained convolutional neural networks (CNNs), which enable training the models based on limited amounts of training data. However, the classification accuracy of these models still tends to be severely limited by the scarcity of representative images from malignant tumours. We propose a novel ensemble-based CNN architecture where multiple CNN models, some of which are pre-trained and some are trained only on the data at hand, along with auxiliary data in the form of metadata associated with the input images, are combined using a meta-learner. The proposed approach improves the model's ability to handle limited and imbalanced data. We demonstrate the benefits of the proposed technique using a dataset with 33126 dermoscopic images from 2056 patients. We evaluate the performance of the proposed technique in terms of the F1-measure, area under the ROC curve (AUC-ROC), and area under the PR-curve (AUC-PR), and compare it with that of seven different benchmark methods, including two recent CNN-based techniques. The proposed technique compares favourably in terms of all the evaluation metrics.
更多查看译文
关键词
skin cancer classification,imbalanced data sets,deep neural networks,skin cancer
AI 理解论文
溯源树
样例
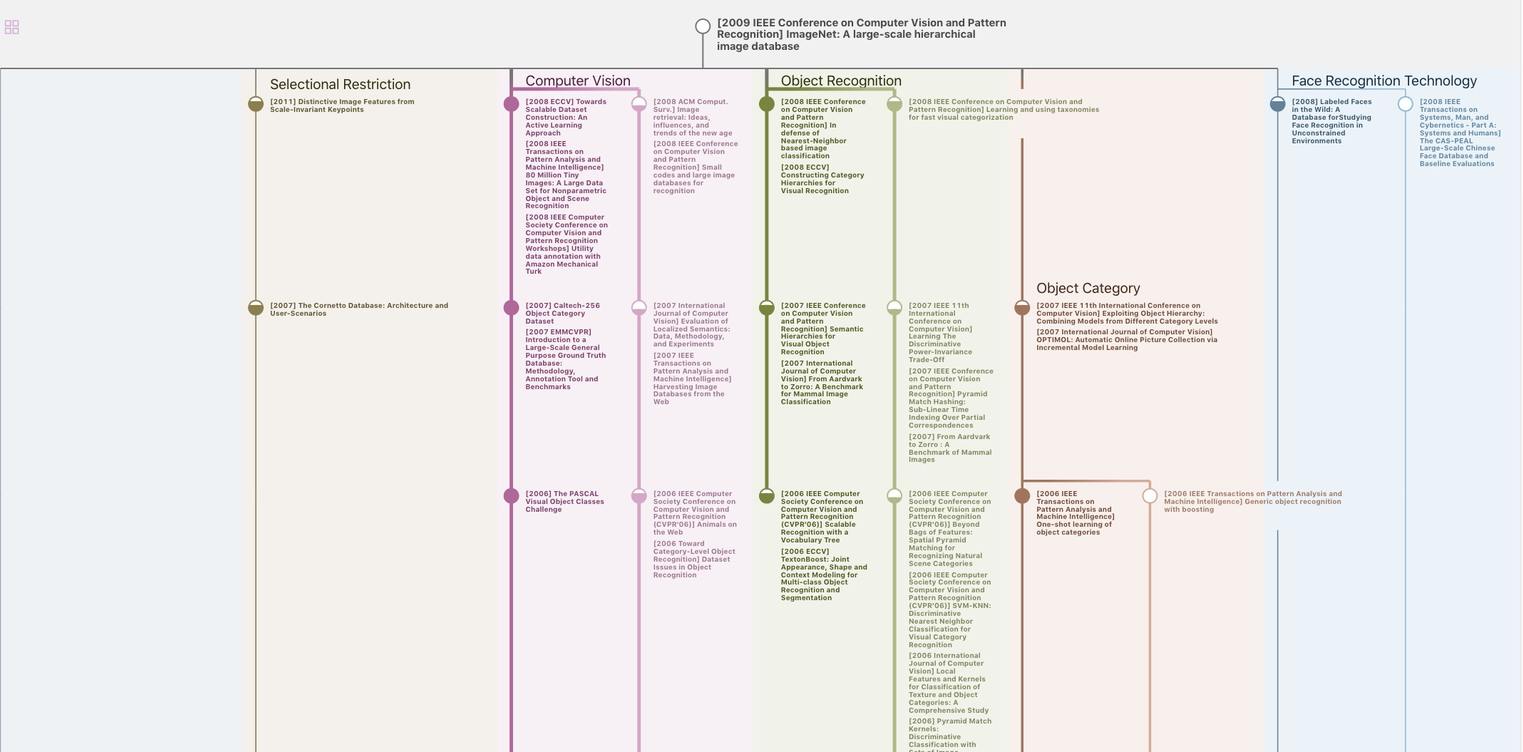
生成溯源树,研究论文发展脉络
Chat Paper
正在生成论文摘要